Online Adaptation of Sampling-Based Motion Planning with Inaccurate Models
arxiv(2024)
摘要
Robotic manipulation relies on analytical or learned models to simulate the
system dynamics. These models are often inaccurate and based on offline
information, so that the robot planner is unable to cope with mismatches
between the expected and the actual behavior of the system (e.g., the presence
of an unexpected obstacle). In these situations, the robot should use
information gathered online to correct its planning strategy and adapt to the
actual system response. We propose a sampling-based motion planning approach
that uses an estimate of the model error and online observations to correct the
planning strategy at each new replanning. Our approach adapts the cost function
and the sampling bias of a kinodynamic motion planner when the outcome of the
executed transitions is different from the expected one (e.g., when the robot
unexpectedly collides with an obstacle) so that future trajectories will avoid
unreliable motions. To infer the properties of a new transition, we introduce
the notion of context-awareness, i.e., we store local environment information
for each executed transition and avoid new transitions with context similar to
previous unreliable ones. This is helpful for leveraging online information
even if the simulated transitions are far (in the state-and-action space) from
the executed ones. Simulation and experimental results show that the proposed
approach increases the success rate in execution and reduces the number of
replannings needed to reach the goal.
更多查看译文
AI 理解论文
溯源树
样例
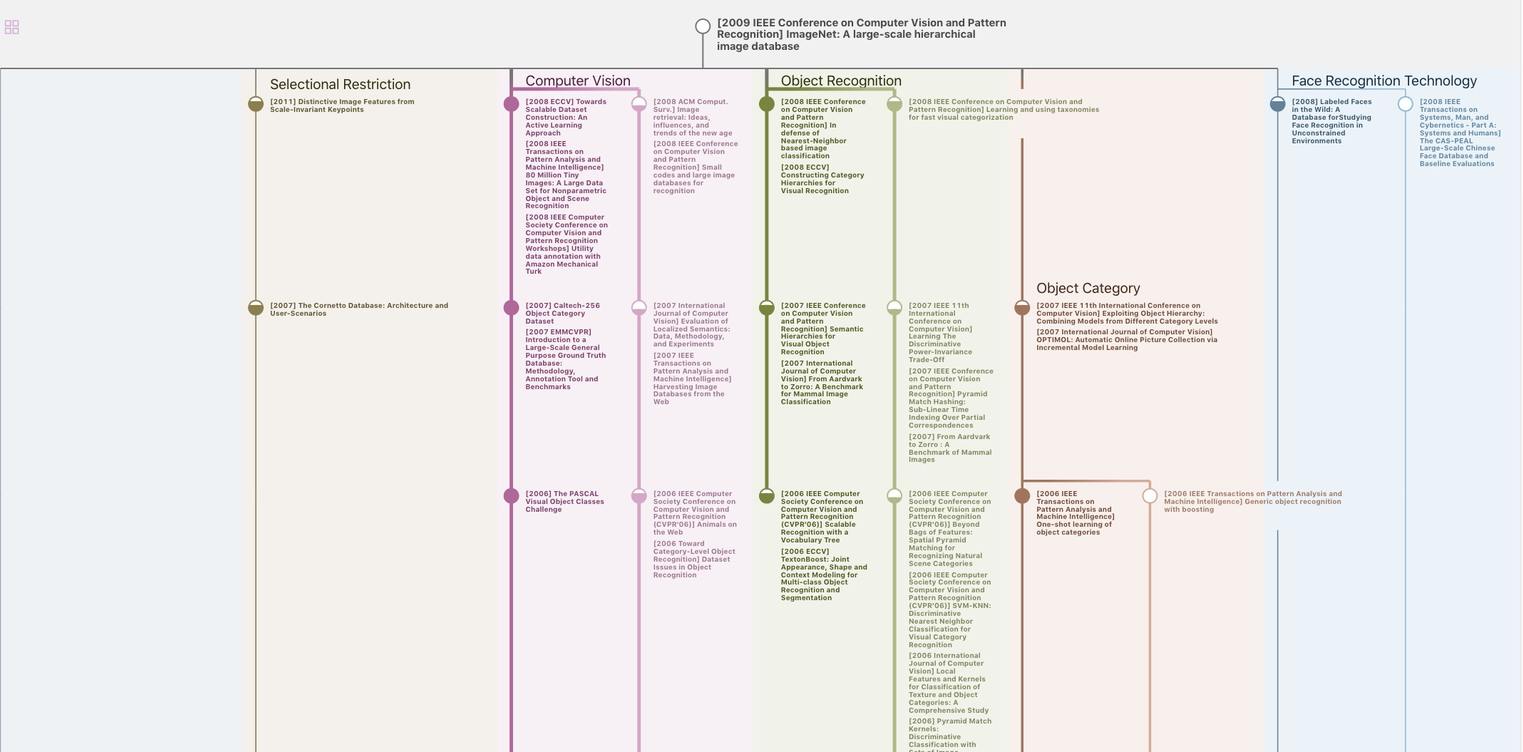
生成溯源树,研究论文发展脉络
Chat Paper
正在生成论文摘要