An improved adaptive Kriging model-based metamodel importance sampling reliability analysis method
Engineering with Computers(2024)
摘要
An improved adaptive Kriging model-based metamodel importance sampling (IS) reliability analysis method is proposed to increase the efficiency of failure probability calculation. First, the silhouette plot method is introduced to judge the optimal number of clusters for k-means to establish the IS density function, thus avoiding the problem of only assuming clusters arbitrarily. Second, considering the prediction uncertainty of the Kriging model, a novel learning function established from the uncertainty of failure probability is proposed for adaptive Kriging model establishment. The proposed learning function is established based on the variance information of failure probability. The major benefit of the proposed learning function is that the distribution characteristic of the IS density function is considered, thus fully reflecting the impact of the IS function on active learning. Finally, the coefficient of variation (COV) information of failure probability is adopted to define a novel stopping criterion for learning function. The performance of the proposed method is verified through different numerical examples. The findings demonstrate that the refined learning strategy effectively identifies samples with substantial contributions to failure probability, showcasing commendable convergence. Particularly notable is its capacity to significantly reduce function call volumes with heightened accuracy for scenarios featuring variable dimensions below 10.
更多查看译文
关键词
Reliability analysis,Importance sampling,Adaptive Kriging model,Learning function,Stopping criterion
AI 理解论文
溯源树
样例
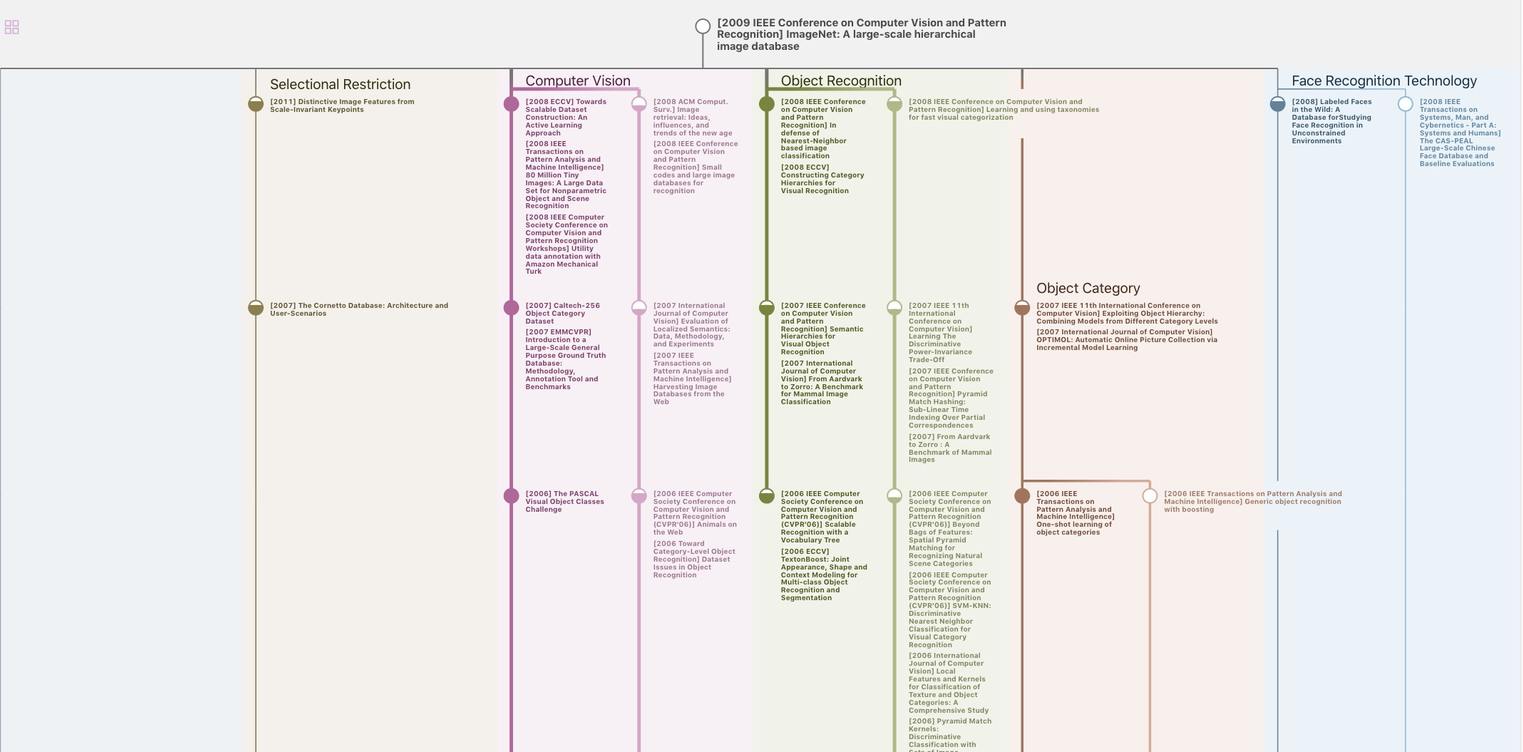
生成溯源树,研究论文发展脉络
Chat Paper
正在生成论文摘要