A Fast and Robust Attention-free Heterogeneous Graph Convolutional Network
IEEE Transactions on Big Data(2024)
摘要
Due to the widespread applications of heterogeneous graphs in the real world, heterogeneous graph neural networks (HGNNs) have developed rapidly and made a great success in recent years. To effectively capture the complex interactions in heterogeneous graphs, various attention mechanisms are widely used in designing HGNNs. However, the employment of these attention mechanisms brings two key problems: high computational complexity and poor robustness. To address these problems, we propose a
Fast
and
Ro
bust attention-free
H
eterogeneous
G
raph
C
onvolutional
N
etwork (FastRo-HGCN) without any attention mechanisms. Specifically, we first construct virtual links based on the topology similarity and feature similarity of the nodes to strengthen the connections between the target nodes. Then, we design type normalization to aggregate and transfer the intra-type and inter-type node information. The above methods are used to reduce the interference of noisy information. Finally, we further enhance the robustness and relieve the negative effects of oversmoothing with the self-loops of nodes. Extensive experimental results on three real-world datasets fully demonstrate that the proposed FastRo-HGCN significantly outperforms the state-of-the-art models. The codes and data of this work are available at
https://github.com/ZZY-GraphMiningLab/FastRo-HGCN
.
更多查看译文
关键词
graph neural network,heterogeneous graph,heterogeneous graph neural network,attention mechanism
AI 理解论文
溯源树
样例
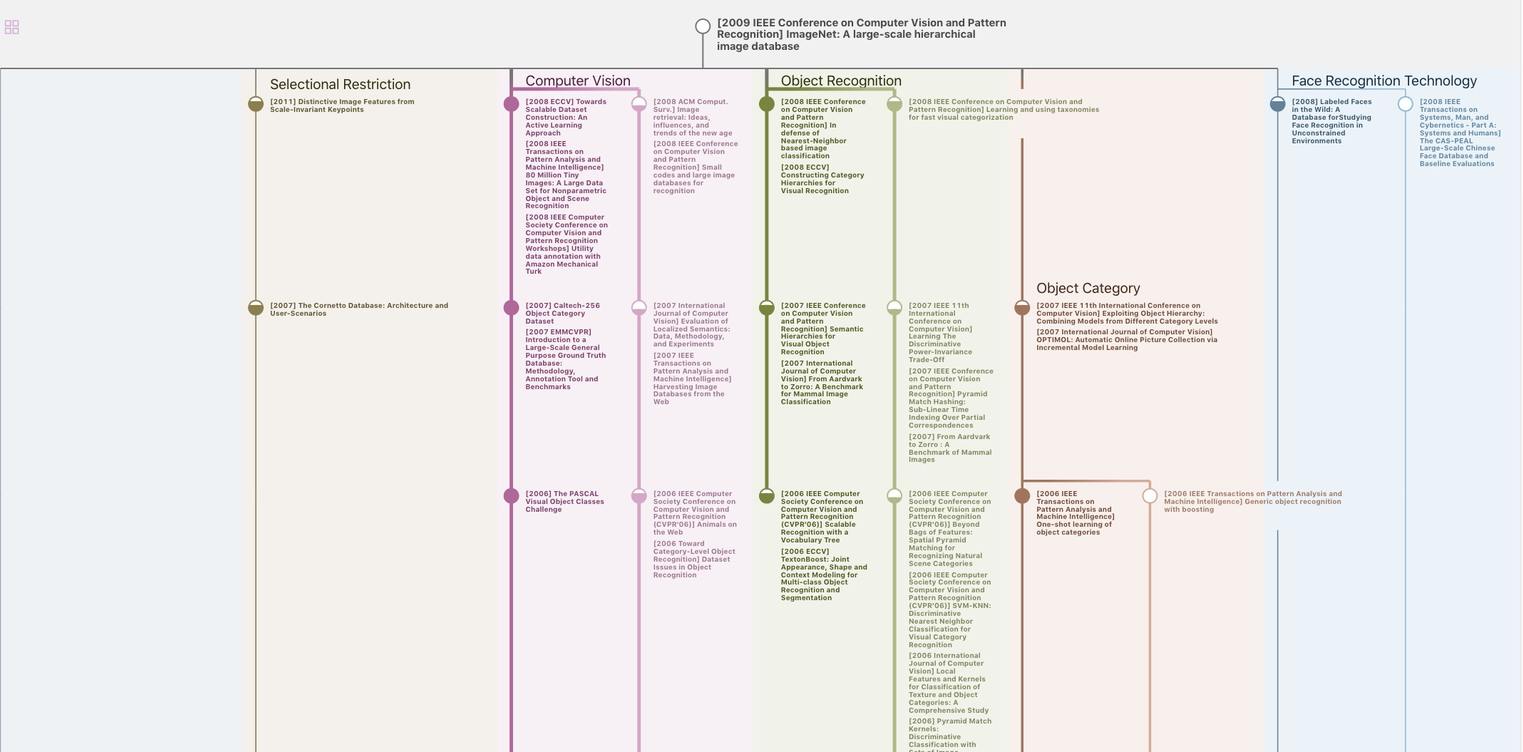
生成溯源树,研究论文发展脉络
Chat Paper
正在生成论文摘要