Time-first approach for land cover mapping using big Earth observation data time-series in a data cube - a case study from the Lake Geneva region (Switzerland)
BIG EARTH DATA(2024)
摘要
Accurate, consistent, and high-resolution Land Use & Cover (LUC) information is fundamental for effectively monitoring landscape dynamics and better apprehending drivers, pressures, state, and impacts on land systems. Nevertheless, the availability of such national products with high thematic accuracy is still limited and consequently researchers and policymakers are constrained to work with data that do not necessarily reflect on-the-ground realities impending to correctly capture details of landscape features as well as limiting the identification and quantification of drivers and rate of change. Hereafter, we took advantage of the Switzerland's official LUC statistical sampling survey and dense time-series of Sentinel-2 data, combining them with Machine and Deep Learning methods to produce an accurate and high spatial resolution land cover map over the Lake Geneva region. Findings suggest that time-first approach is a valuable alternative to space-first approaches, accounting for the intra-annual variability of classes, hence improving classification performances. Results demonstrate that Deep Learning methods outperform more traditional Machine Learning ones such as Random Forest, providing more accurate predictions with lower uncertainty. The produced land cover map has a high accuracy, an improved spatial resolution, while at the same time preserving the statistical significance (i.e. class proportion) of the official national dataset. This work paves the way towards the objective to produce a yearly high resolution land cover map of Switzerland and potentially implement a continuous land change monitoring capability. However further work is required to properly address challenges such as the need for increased temporal resolution for LUC information or the quality of training samples.
更多查看译文
关键词
Land cover,Sentinel-2,time-series,SITS,Arealstatistik
AI 理解论文
溯源树
样例
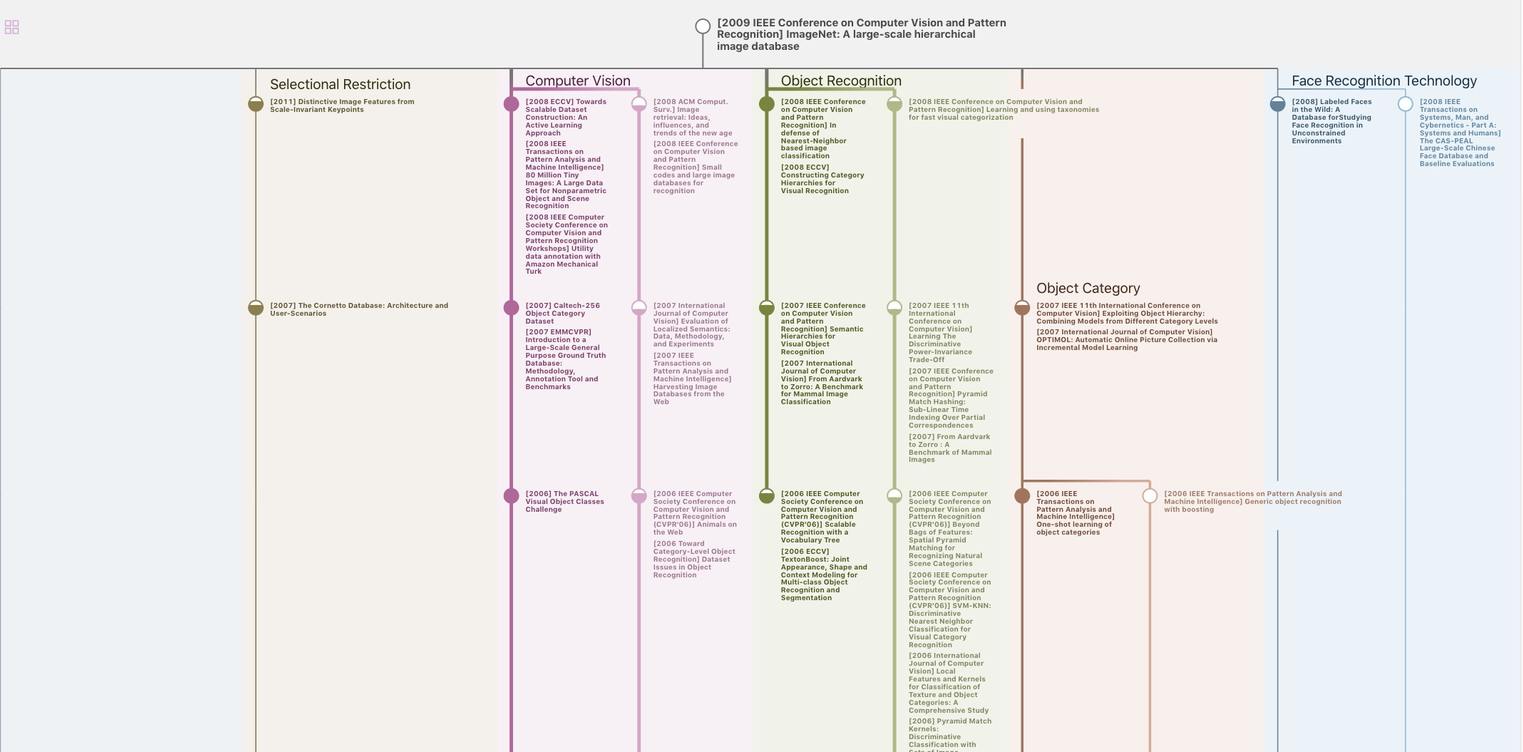
生成溯源树,研究论文发展脉络
Chat Paper
正在生成论文摘要