Using Large Langue Models to Augment (Rather Than Replace) Human Feedback in Higher Education Improves Perceived Feedback Quality
crossref(2024)
摘要
Formative feedback on assignments such as essays or theses is deemed necessary for students’ academic development in higher education. However, providing high quality feedback can be time-intensive and challenging, and students frequently report dissatisfaction with feedback quality. Here we explore a possible solution, namely using large language models (LLMs) to augment feedback provided by instructors. One potential obstacle to using LLM-augmented feedback is algorithm aversion, which might lead students to deprecate LLM-augmented feedback. Therefore, we examined students’ perceptions of human versus LLM-augmented feedback. In a pre-registered study, participants (N = 112) evaluated original human-generated versus LLM-augmented feedback on a previous assignment. Our results show evidence against algorithm aversion. Furthermore, participants rated the quality of LLM-augmented feedback substantially higher and strongly preferred it over the human-generated original. Our findings demonstrate the potential of LLMs to solve the persistent problem of low perceived feedback quality in higher education.
更多查看译文
AI 理解论文
溯源树
样例
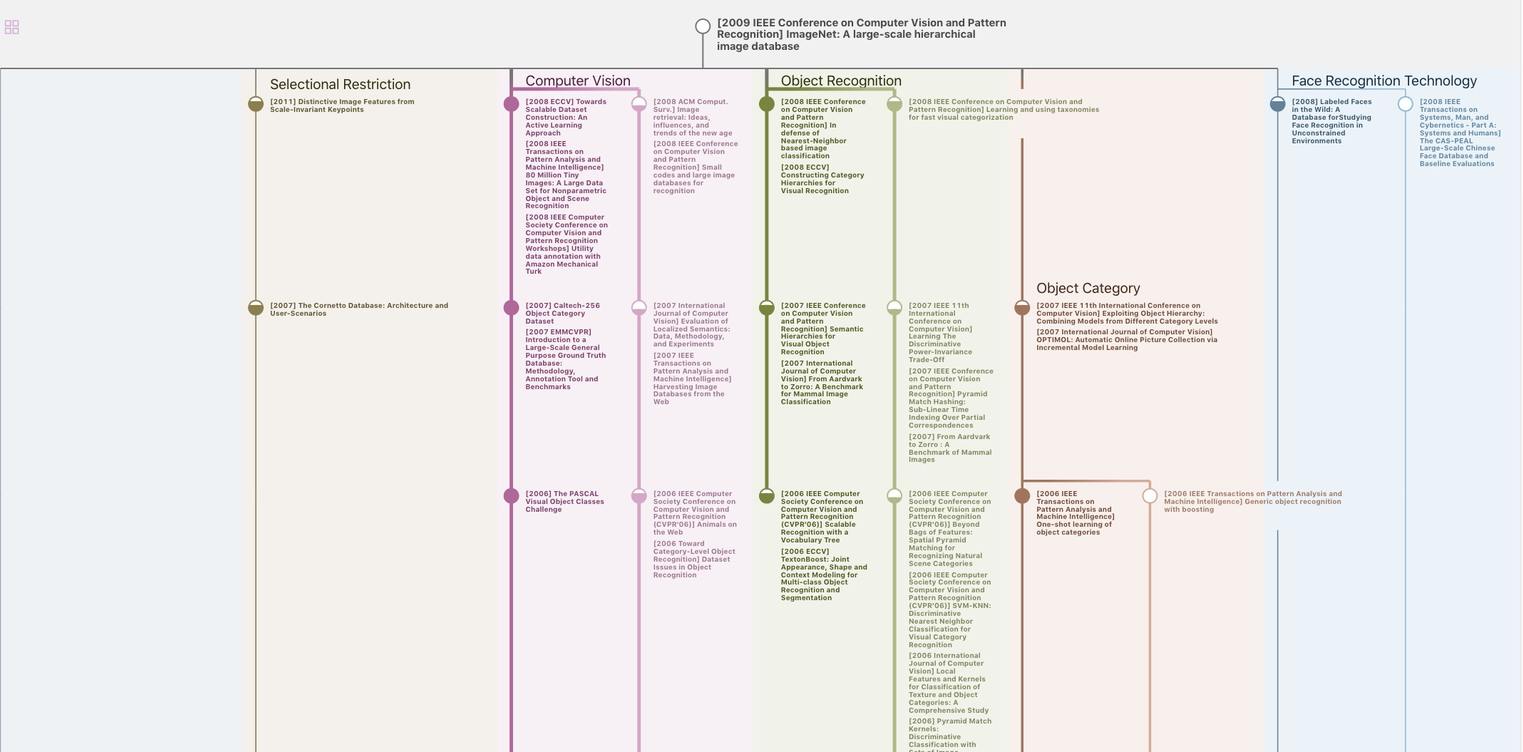
生成溯源树,研究论文发展脉络
Chat Paper
正在生成论文摘要