Cross-Domain Transfer Hashing for Efficient Cross-modal Retrieval
IEEE Transactions on Circuits and Systems for Video Technology(2024)
摘要
Unsupervised cross-modal hashing presents significant advantages in heterogeneous modality retrieval, offering label scalability, high retrieval efficiency, and low storage costs. However, the lack of explicit semantic supervision in this process results in a noticeable semantic deficit, impacting retrieval performance. In this paper, we address this challenge with a dual-pronged approach: Cross-Domain Transfer Hashing (CDTH), a lightweight weakly-supervised cross-modal hashing model. Our method leverages a semantically rich auxiliary domain to augment the target unsupervised cross-modal hash learning process. Simultaneously, we design a lightweight target cross-modal hashing network to reduce semantic requirements, lessening the burden of parameter optimization. Within the auxiliary domain, we perform direct semantic transfer with hashing network parameter transfer and indirect correlation semantic transfer by constructing an auxiliary semantic correlation graph with the identified cross-domain semantic consistent samples. In the target domain, we generate pseudo-labels using CLIP and establish a target weak semantic correlation graph. These two graphs collaborate to bolster the target cross-modal hashing training process. Extensive experiments on three publicly available datasets affirm the superiority of our approach in both retrieval accuracy and training efficiency. The source code for our method is accessible at: https://github.com/WangBowen7/CDTH.
更多查看译文
AI 理解论文
溯源树
样例
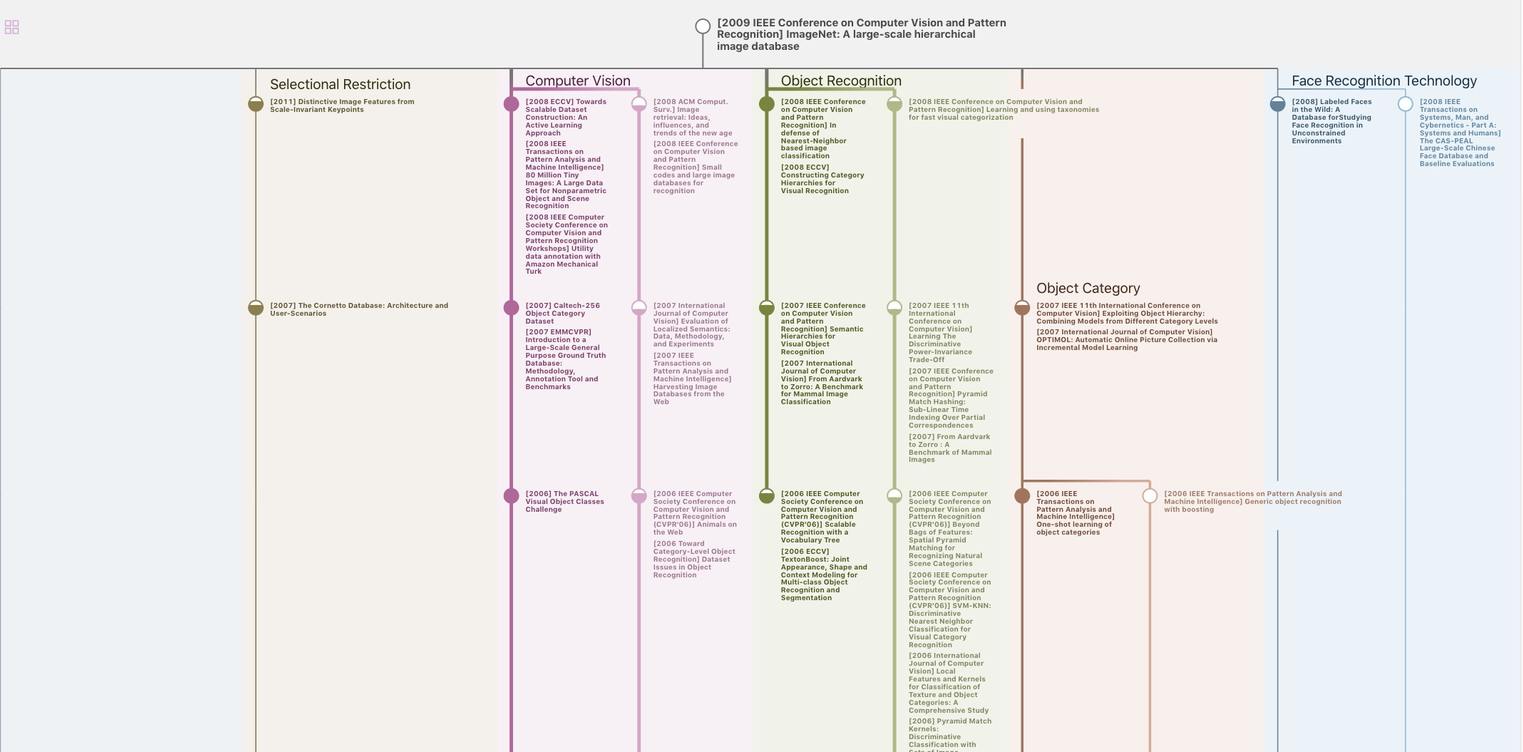
生成溯源树,研究论文发展脉络
Chat Paper
正在生成论文摘要