Solar Flare Forecasting Based on Magnetogram Sequences Learning with Multiscale Vision Transformers and Data Augmentation Techniques
Solar Physics(2024)
摘要
Solar flares are releases of electromagnetic energy generally occurring in active solar regions with magnetic fields, known as sunspots. The burst of radiation released by a solar flare can reach Earth’s atmosphere in a few minutes. High-intensity solar flares, M- or X-class flares, can significantly impact some of Earth’s activities and technologies, such as satellites, telecommunications, and electrical power systems. Therefore driving efforts in high-intensity solar flare forecasting systems is crucial. A forecasting model that observes the evolution of active regions may analyze a set of attributes that indicate which active regions can be precursors to solar flares. Recent work has focused on deep-learning models that consider the evolution of active regions in the Sun. However, M- and X-class flares are spurious in the solar-cycle period. That situation leads to an imbalanced dataset, increasing the effort to develop machine-learning models for forecasting. Therefore we propose transformers-based models to forecast ≥M-class flares, taking sequences of line-of-sight magnetogram images as input. In addition, we apply data augmentation techniques and other methods to deal with training on imbalanced datasets. Our fine-tuned models outperformed state-of-the-art work using image processing to forecast ≥M-class flares in the next 48 h with an approximate True Skill Statistic ( TSS ) of 0.8. Moreover, the data augmentation techniques applied to the training set kept the TSS stable and improved most of the secondary performance metrics analyzed.
更多查看译文
关键词
Deep learning,Image processing,Sunspots,Imbalanced dataset
AI 理解论文
溯源树
样例
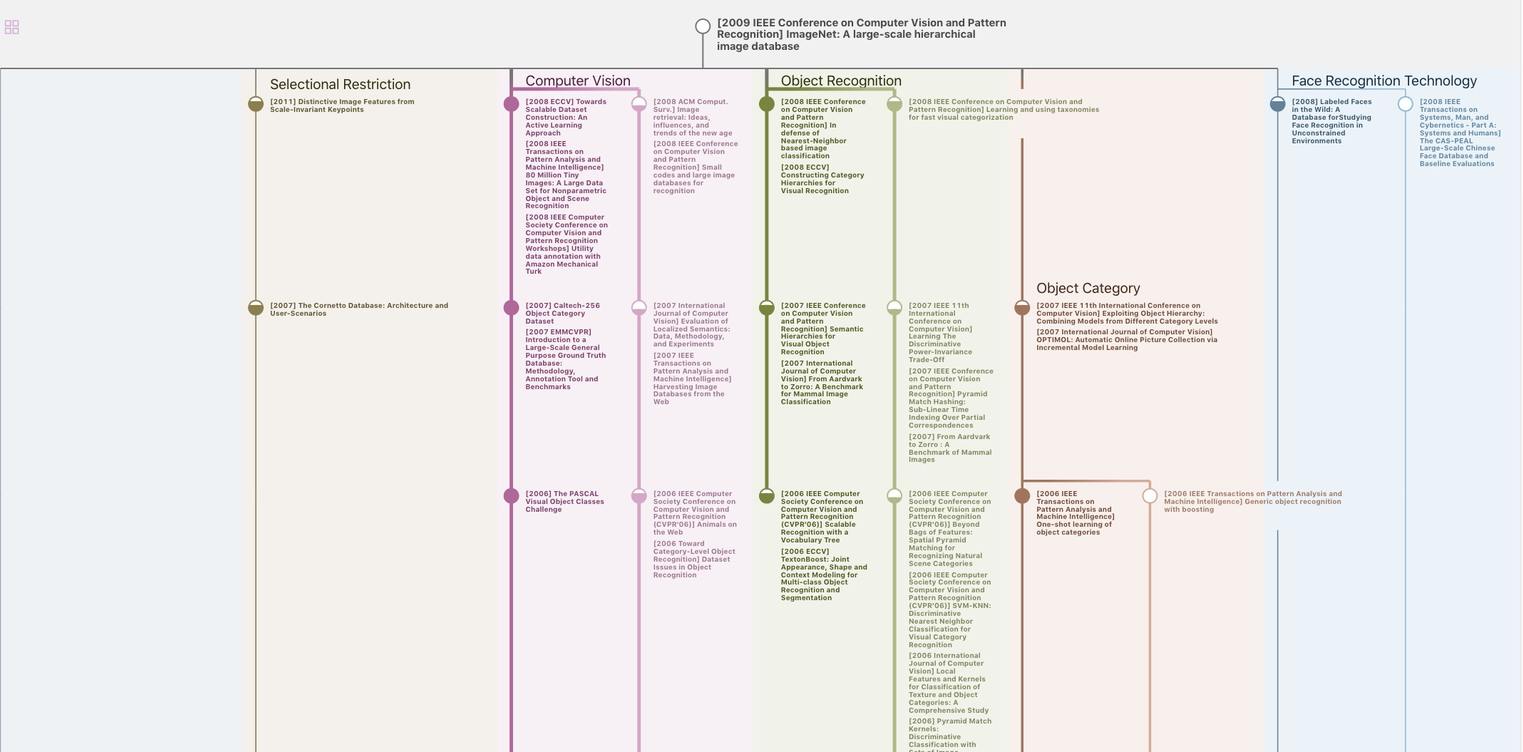
生成溯源树,研究论文发展脉络
Chat Paper
正在生成论文摘要