Spectral Super-Resolution via Deep Low-Rank Tensor Representation
IEEE TRANSACTIONS ON NEURAL NETWORKS AND LEARNING SYSTEMS(2024)
摘要
Spectral super-resolution has attracted the attention of more researchers for obtaining hyperspectral images (HSIs) in a simpler and cheaper way. Although many convolutional neural network (CNN)-based approaches have yielded impressive results, most of them ignore the low-rank prior of HSIs resulting in huge computational and storage costs. In addition, the ability of CNN-based methods to capture the correlation of global information is limited by the receptive field. To surmount the problem, we design a novel low-rank tensor reconstruction network (LTRN) for spectral super-resolution. Specifically, we treat the features of HSIs as 3-D tensors with low-rank properties due to their spectral similarity and spatial sparsity. Then, we combine canonical-polyadic (CP) decomposition with neural networks to design an adaptive low-rank prior learning (ALPL) module that enables feature learning in a 1-D space. In this module, there are two core modules: the adaptive vector learning (AVL) module and the multidimensionwise multihead self-attention (MMSA) module. The AVL module is designed to compress an HSI into a 1-D space by using a vector to represent its information. The MMSA module is introduced to improve the ability to capture the long-range dependencies in the row, column, and spectral dimensions, respectively. Finally, our LTRN, mainly cascaded by several ALPL modules and feedforward networks (FFNs), achieves high-quality spectral super-resolution with fewer parameters. To test the effect of our method, we conduct experiments on two datasets: the CAVE dataset and the Harvard dataset. Experimental results show that our LTRN not only is as effective as state-of-the-art methods but also has fewer parameters.
更多查看译文
关键词
Adaptive low-rank prior learning (ALPL),canonical-polyadic (CP) decomposition,multidimensionwise multihead self-attention (MMSA),spectral super-resolution
AI 理解论文
溯源树
样例
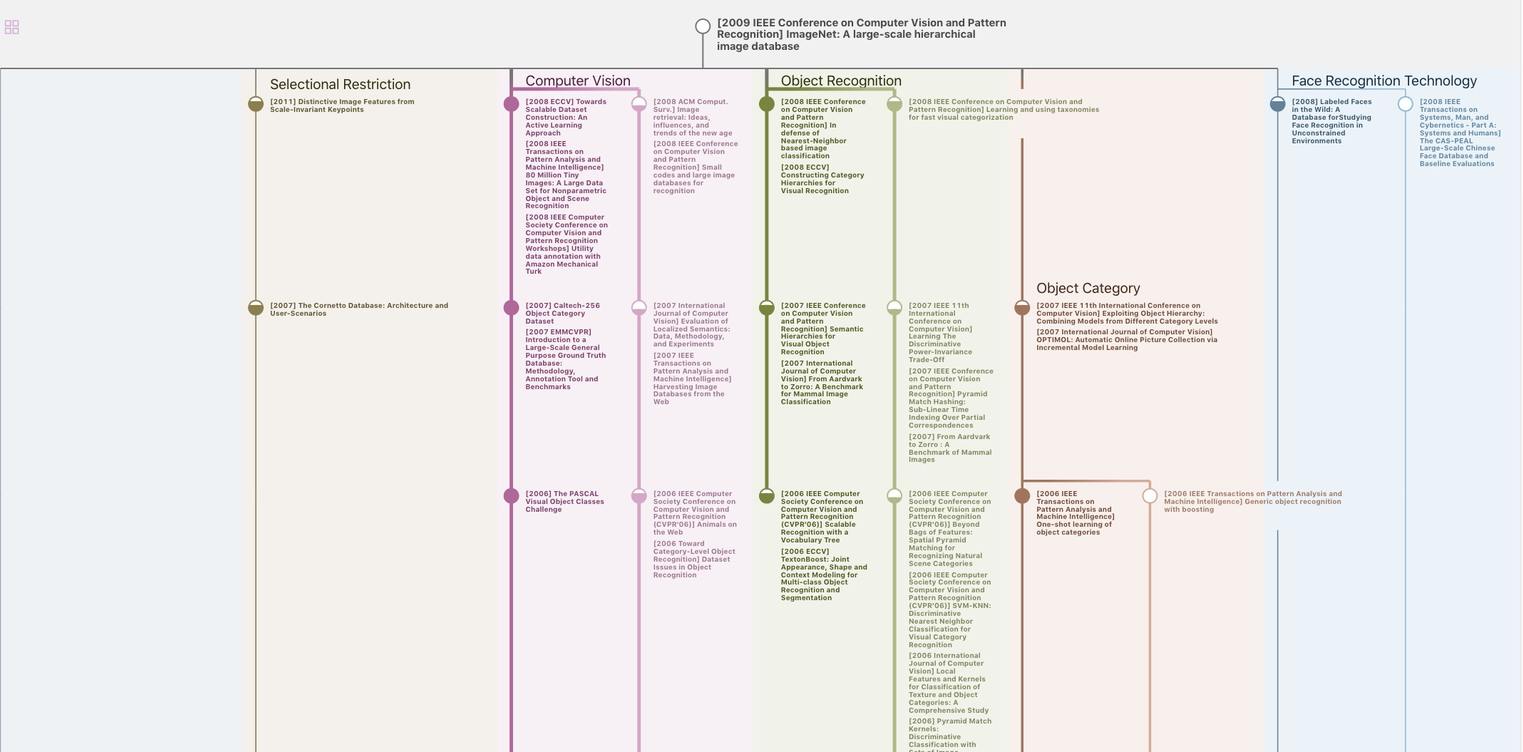
生成溯源树,研究论文发展脉络
Chat Paper
正在生成论文摘要