Consequential Advancements of Self-Supervised Learning (SSL) in Deep Learning Contexts
MATHEMATICS(2024)
摘要
Self-supervised learning (SSL) is a potential deep learning (DL) technique that uses massive volumes of unlabeled data to train neural networks. SSL techniques have evolved in response to the poor classification performance of conventional and even modern machine learning (ML) and DL models of enormous unlabeled data produced periodically in different disciplines. However, the literature does not fully address SSL's practicalities and workabilities necessary for industrial engineering and medicine. Accordingly, this thorough review is administered to identify these prominent possibilities for prediction, focusing on industrial and medical fields. This extensive survey, with its pivotal outcomes, could support industrial engineers and medical personnel in efficiently predicting machinery faults and patients' ailments without referring to traditional numerical models that require massive computational budgets, time, storage, and effort for data annotation. Additionally, the review's numerous addressed ideas could encourage industry and healthcare actors to take SSL principles into an agile application to achieve precise maintenance prognostics and illness diagnosis with remarkable levels of accuracy and feasibility, simulating functional human thinking and cognition without compromising prediction efficacy.
更多查看译文
关键词
deep learning (DL),self-supervised learning (SSL),machine learning (ML),cognition,classification,data annotation
AI 理解论文
溯源树
样例
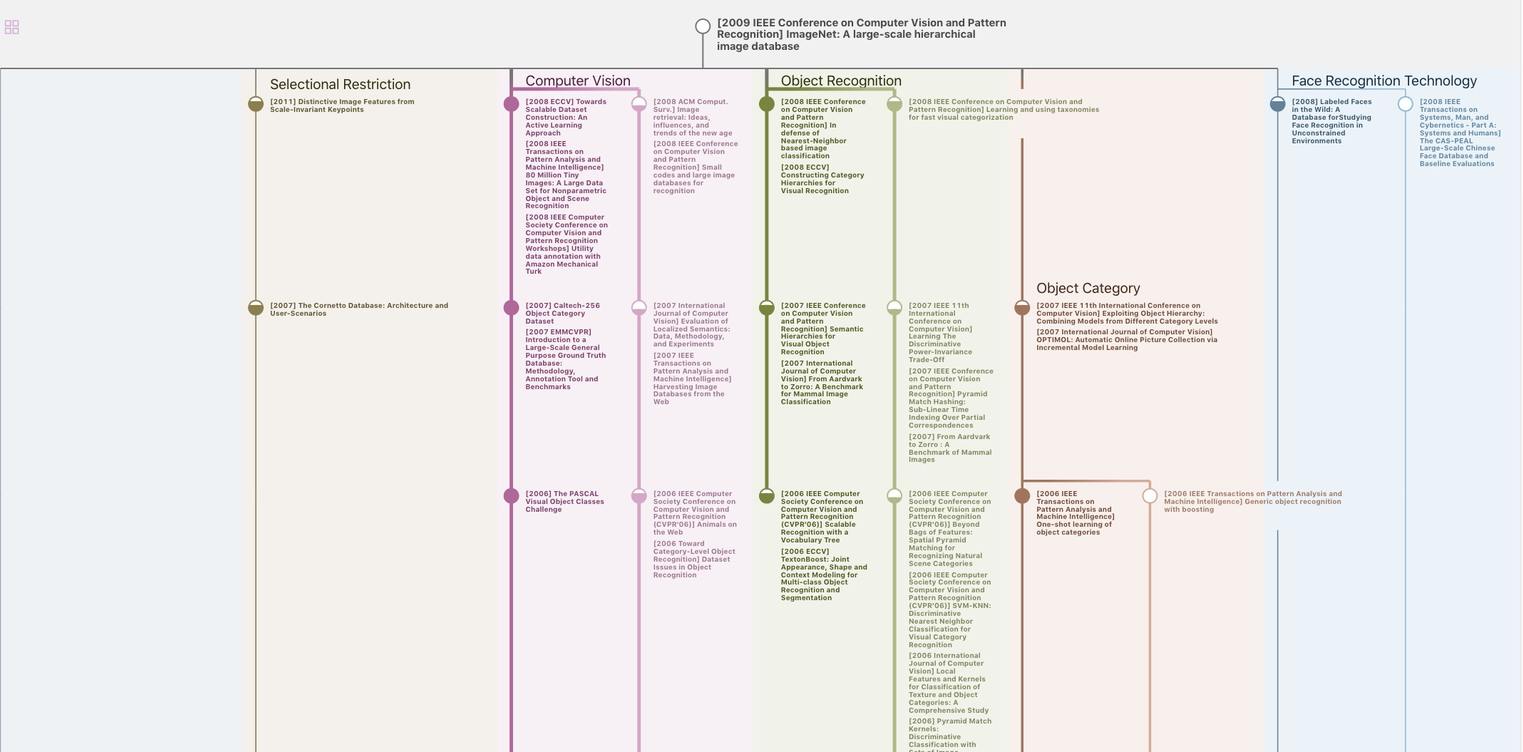
生成溯源树,研究论文发展脉络
Chat Paper
正在生成论文摘要