Advancing Ultrasonic Defect Detection in High-Speed Wheels via UT-YOLO
SENSORS(2024)
摘要
In the context of defect detection in high-speed railway train wheels, particularly in ultrasonic-testing B-scan images characterized by their small size and complexity, the need for a robust solution is paramount. The proposed algorithm, UT-YOLO, was meticulously designed to address the specific challenges presented by these images. UT-YOLO enhances its learning capacity, accuracy in detecting small targets, and overall processing speed by adopting optimized convolutional layers, a special layer design, and an attention mechanism. This algorithm exhibits superior performance on high-speed railway wheel UT datasets, indicating its potential. Crucially, UT-YOLO meets real-time processing requirements, positioning it as a practical solution for the dynamic and high-speed environment of railway inspections. In experimental evaluations, UT-YOLO exhibited good performance in best recall, mAP@0.5 and mAP@0.5:0.95 increased by 37%, 36%, and 43%, respectively; and its speed also met the needs of real-time performance. Moreover, an ultrasonic defect detection data set based on real wheels was created, and this research has been applied in actual scenarios and has helped to greatly improve manual detection efficiency.
更多查看译文
关键词
ultrasonic testing,B-scan images,defect detection,UT-YOLO
AI 理解论文
溯源树
样例
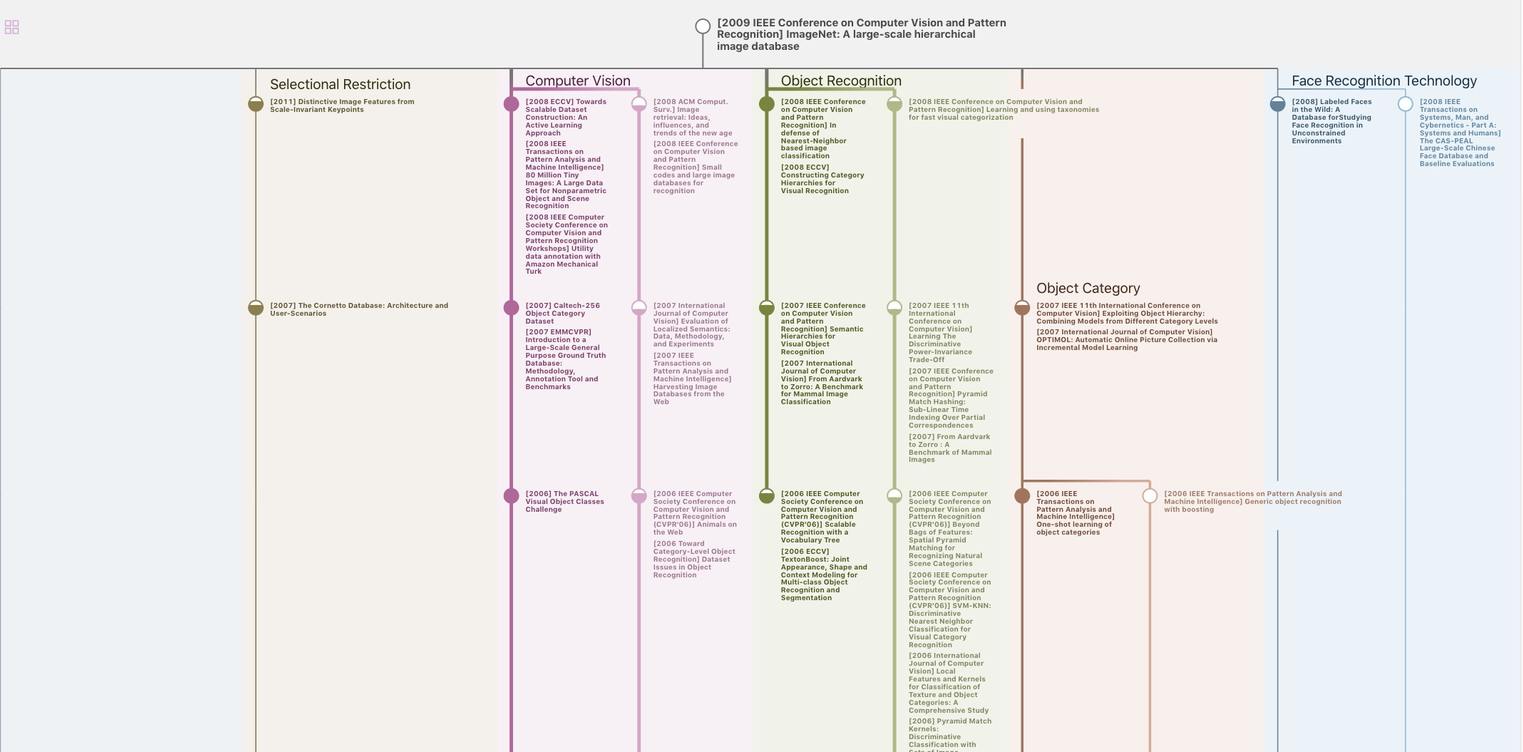
生成溯源树,研究论文发展脉络
Chat Paper
正在生成论文摘要