Style-Guided Adversarial Teacher for Cross-Domain Object Detection
ELECTRONICS(2024)
摘要
The teacher-student framework is widely employed for cross-domain object detection. However, it suffers from two problems. One is that large distribution discrepancies will cause critical performance drops. The other is that the samples that deviate from the overall distributions of both domains will greatly mislead the model. To solve these problems, we propose a style-guided adversarial teacher (SGAT) method for domain adaptation. Specifically, on the domain level, we generate target-like images based on source images to effectively narrow the gaps between domains. On the sample level, we denoise samples by estimating the probability density ratio of the 'target-style' and target distributions, which could filter out the unrelated samples and highlight the related ones. In this way, we could guarantee reliable samples. With these reliable samples, we learn the domain-invariant features through teacher-student mutual learning and adversarial learning. Extensive experiments verify the effectiveness of our method. In particular, we achieve 52.9% mAP on Clipart1k and 42.7% on Comic2k, which are 6.4% and 5.0% higher than the compared baselines.
更多查看译文
关键词
target-style image generation,domain adaptation,teacher-student framework,adversarial learning,sample denoising
AI 理解论文
溯源树
样例
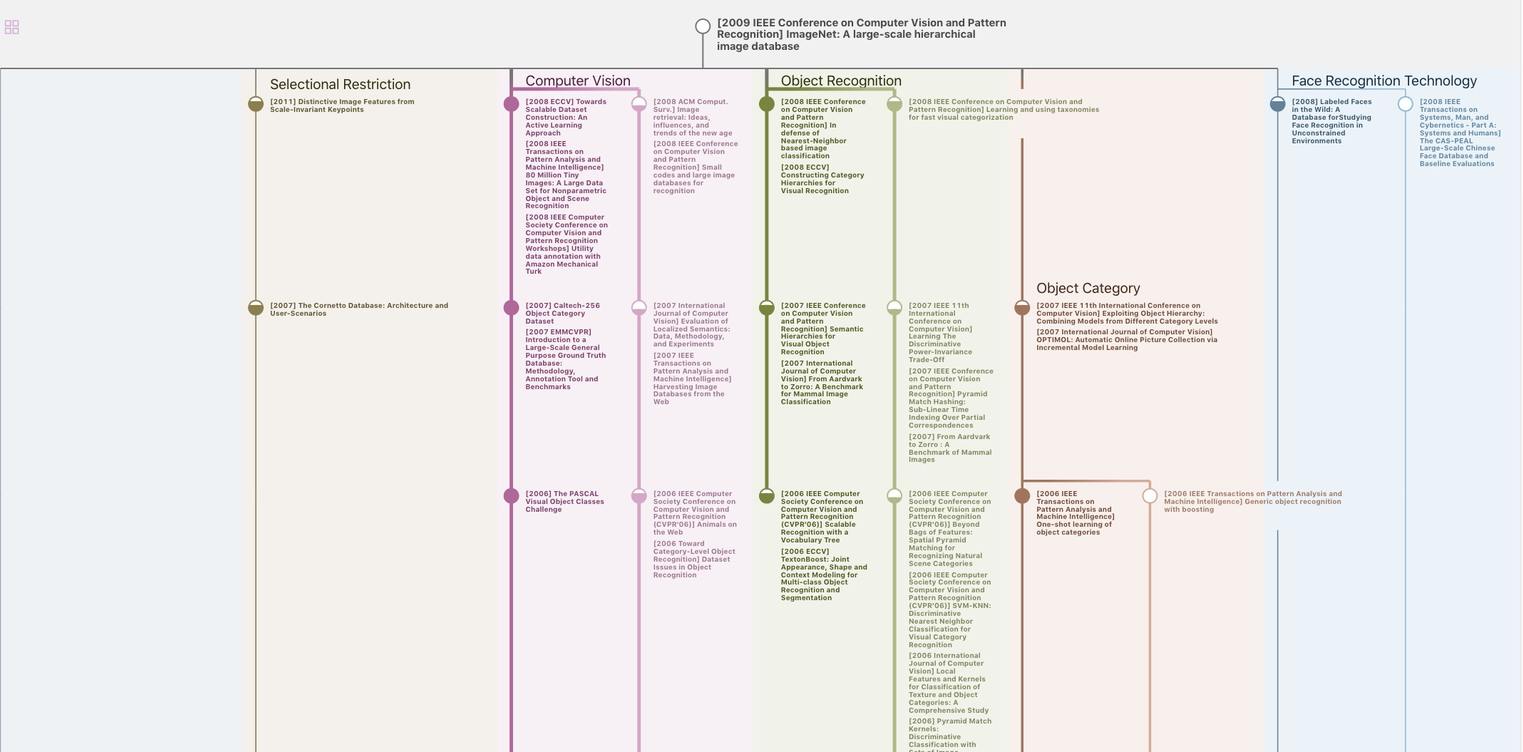
生成溯源树,研究论文发展脉络
Chat Paper
正在生成论文摘要