Siamese Networks for Clinically Relevant Bacteria Classification Based on Raman Spectroscopy
MOLECULES(2024)
Abstract
Identifying bacterial strains is essential in microbiology for various practical applications, such as disease diagnosis and quality monitoring of food and water. Classical machine learning algorithms have been utilized to identify bacteria based on their Raman spectra. However, convolutional neural networks (CNNs) offer higher classification accuracy, but they require extensive training sets and retraining of previous untrained class targets can be costly and time-consuming. Siamese networks have emerged as a promising solution. They are composed of two CNNs with the same structure and a final network that acts as a distance metric, converting the classification problem into a similarity problem. Classical machine learning approaches, shallow and deep CNNs, and two Siamese network variants were tailored and tested on Raman spectral datasets of bacteria. The methods were evaluated based on mean sensitivity, training time, prediction time, and the number of parameters. In this comparison, Siamese-model2 achieved the highest mean sensitivity of 83.61 +/- 4.73 and demonstrated remarkable performance in handling unbalanced and limited data scenarios, achieving a prediction accuracy of 73%. Therefore, the choice of model depends on the specific trade-off between accuracy, (prediction/training) time, and resources for the particular application. Classical machine learning models and shallow CNN models may be more suitable if time and computational resources are a concern. Siamese networks are a good choice for small datasets and CNN for extensive data.
MoreTranslated text
Key words
Siamese networks,machine learning,bacteria classification,Raman spectroscopy
AI Read Science
Must-Reading Tree
Example
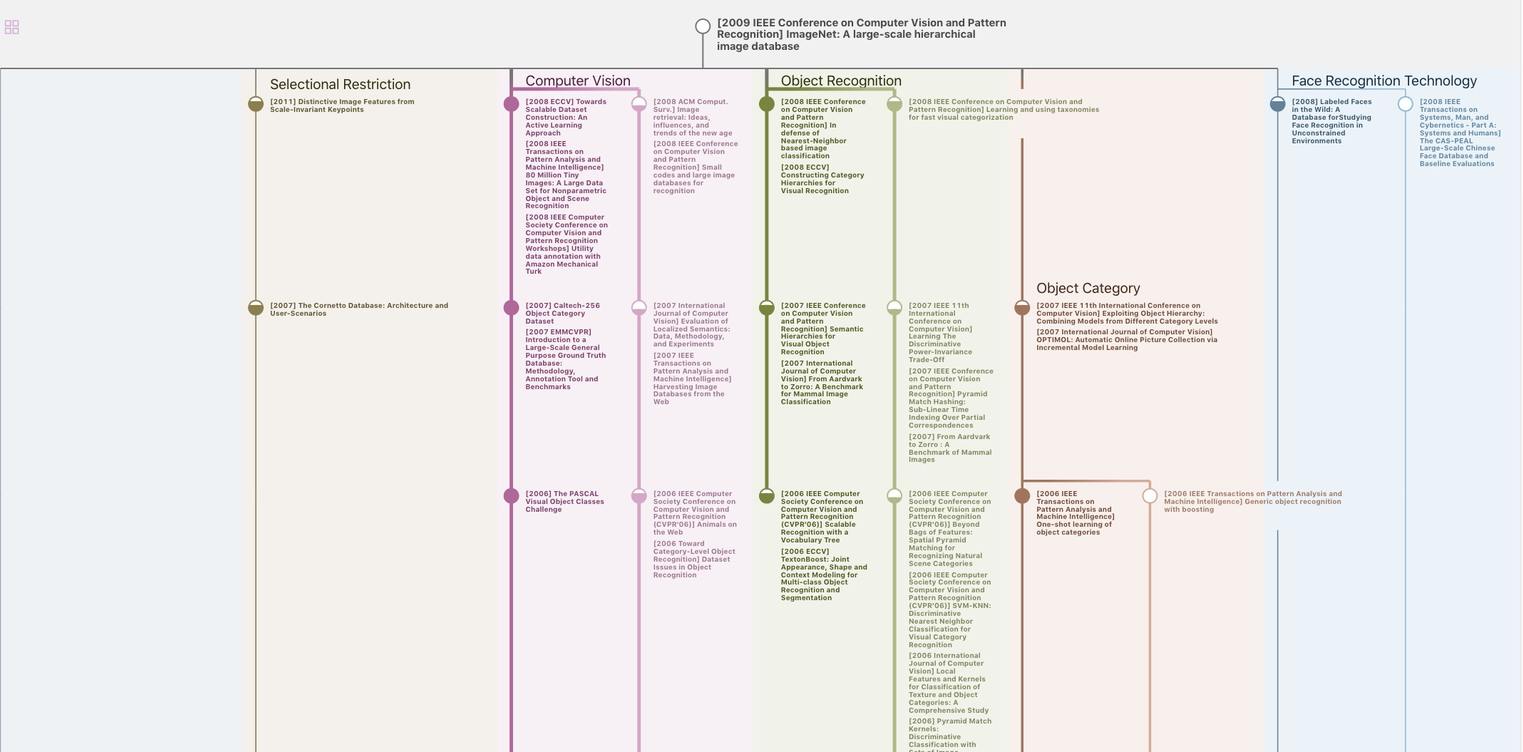
Generate MRT to find the research sequence of this paper
Chat Paper
Summary is being generated by the instructions you defined