A Transfer Learning-Enhanced Generative Adversarial Network for Downscaling Sea Surface Height through Heterogeneous Data Fusion
REMOTE SENSING(2024)
摘要
In recent decades, satellites have played a pivotal role in observing ocean dynamics, providing diverse datasets with varying spatial resolutions. Notably, within these datasets, sea surface height (SSH) data typically exhibit low resolution, while sea surface temperature (SST) data have significantly higher resolution. This study introduces a Transfer Learning-enhanced Generative Adversarial Network (TLGAN) for reconstructing high-resolution SSH fields through the fusion of heterogeneous SST data. In contrast to alternative deep learning approaches that involve directly stacking SSH and SST data as input channels in neural networks, our methodology utilizes bifurcated blocks comprising Residual Dense Module and Residual Feature Distillation Module to extract features from SSH and SST data, respectively. A pixelshuffle module-based upscaling block is then concatenated to map these features into a common latent space. Employing a hybrid strategy involving adversarial training and transfer learning, we overcome the limitation that SST and SSH data should share the same time dimension and achieve significant resolution enhancement in SSH reconstruction. Experimental results demonstrate that, when compared to interpolation method, TLGAN effectively reduces reconstruction errors and fusing SST data could significantly enhance in generating more realistic and physically plausible results.
更多查看译文
关键词
sea surface temperature,sea surface height,super-resolution reconstruction,generative adversarial network,transfer learning,data fusion
AI 理解论文
溯源树
样例
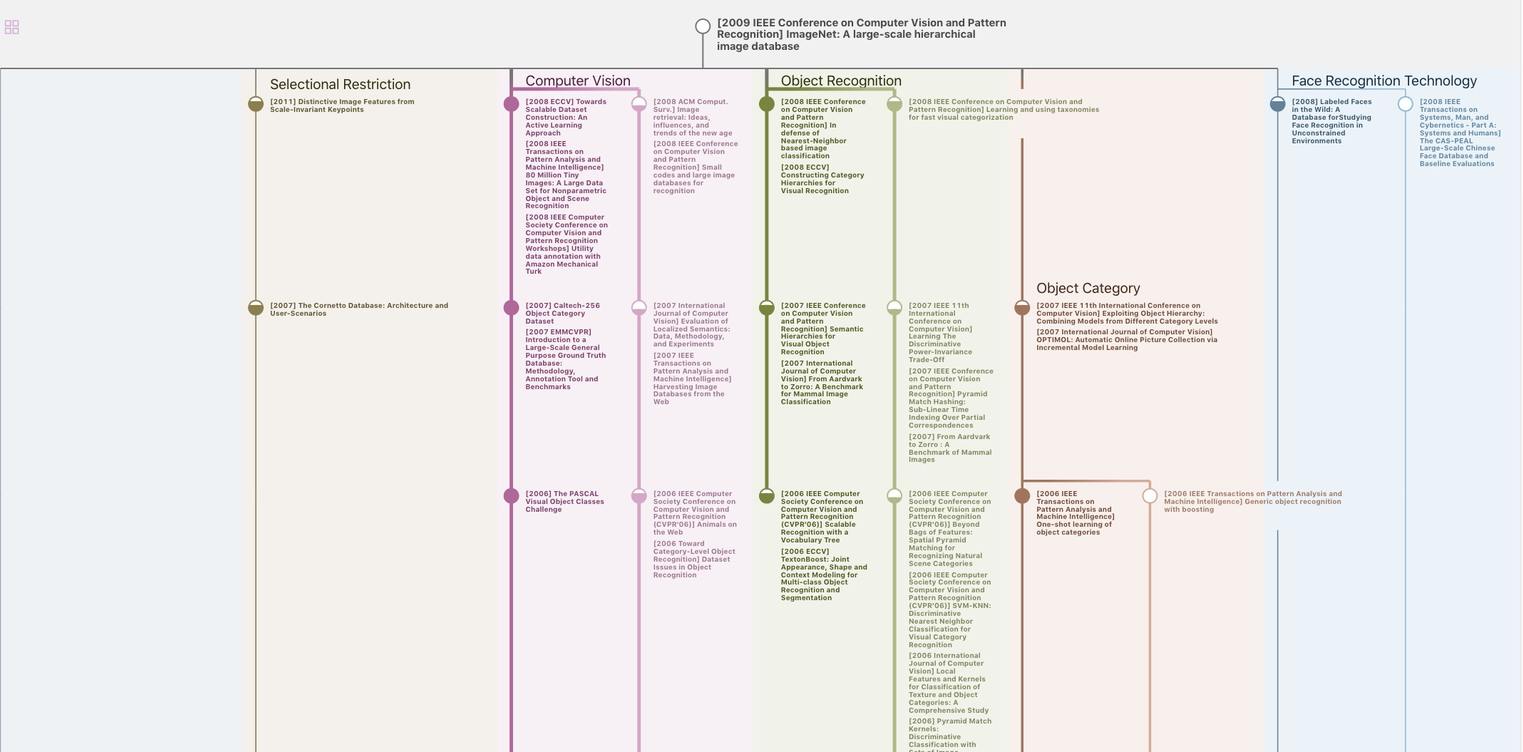
生成溯源树,研究论文发展脉络
Chat Paper
正在生成论文摘要