Identification of Active Components for Sports Supplements: Machine Learning-Driven Classification and Cell-Based Validation
ACS OMEGA(2024)
摘要
The identification of active components is critical for the development of sports supplements. However, high-throughput screening of active components remains a challenge. This study sought to construct prediction models to screen active components from herbal medicines via machine learning and validate the screening by using cell-based assays. The six constructed models had an accuracy of >0.88. Twelve randomly selected active components from the screening were tested for their active potency on C2C12 cells, and 11 components induced a significant increase in myotube diameters and protein synthesis. The effect and mechanism of luteolin among the 11 active components as potential sports supplements were then investigated by using immunofluorescence staining and high-content imaging analysis. It showed that luteolin increased the skeletal muscle performance via the activation of PGC-1 alpha and MAPK signaling pathways. Thus, high-throughput prediction models can be effectively used to screen active components as sports supplements.
更多查看译文
AI 理解论文
溯源树
样例
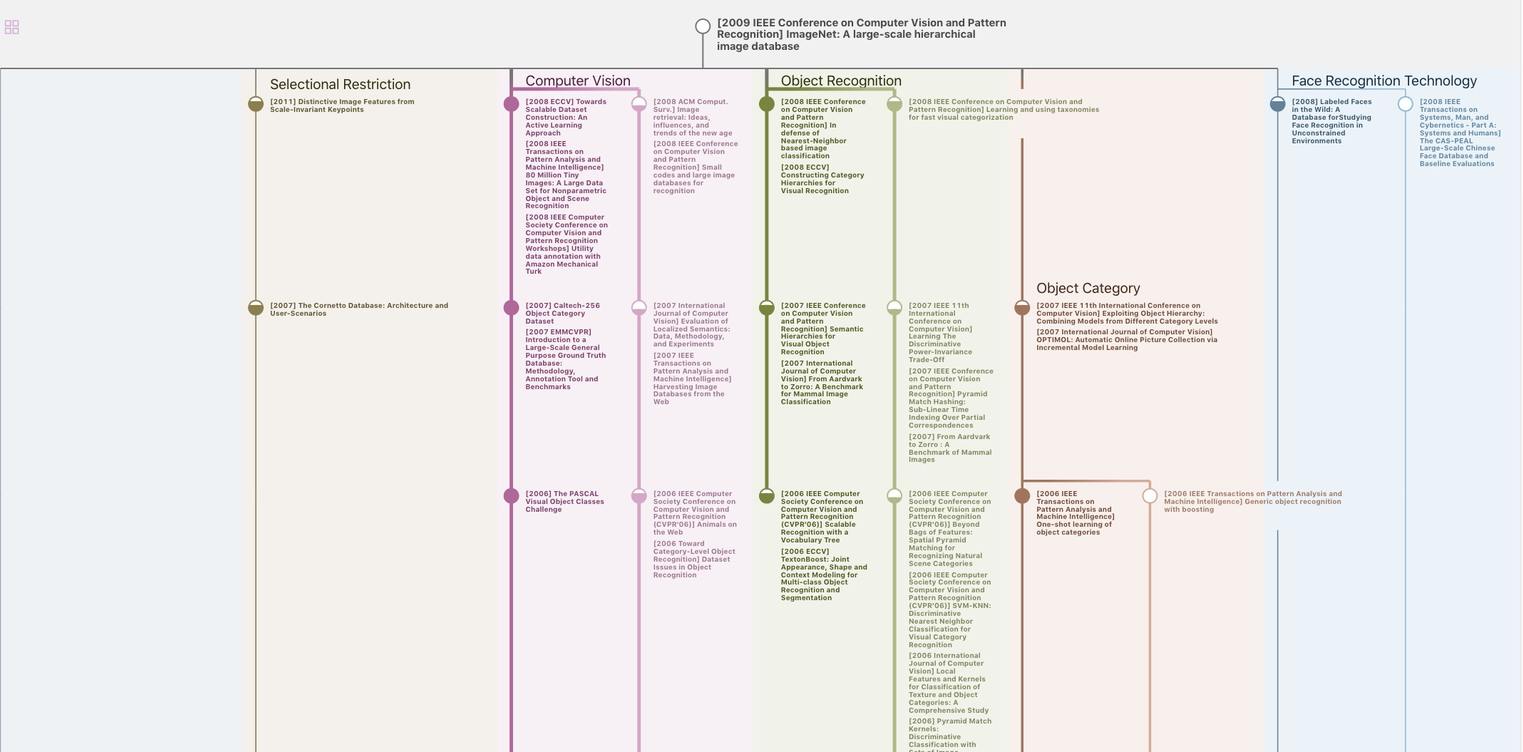
生成溯源树,研究论文发展脉络
Chat Paper
正在生成论文摘要