Hybrid physics-machine learning models for predicting rate of penetration in the Halahatang oil field, Tarim Basin
Scientific Reports(2024)
摘要
Rate of penetration (ROP) is a key factor in drilling optimization, cost reduction and drilling cycle shortening. Due to the systematicity, complexity and uncertainty of drilling operations, however, it has always been a problem to establish a highly accurate and interpretable ROP prediction model to guide and optimize drilling operations. To solve this problem in the Tarim Basin, this study proposes four categories of hybrid physics-machine learning (ML) methods for modeling. One of which is residual modeling, in which an ML model learns to predict errors or residuals, via a physical model; the second is integrated coupling, in which the output of the physical model is used as an input to the ML model; the third is simple average, in which predictions from both the physical model and the ML model are combined; and the last is bootstrap aggregating (bagging), which follows the idea of ensemble learning to combine different physical models’ advantages. A total of 5655 real data points from the Halahatang oil field were used to test the performance of the various models. The results showed that the residual modeling model, with an R 2 of 0.9936, had the best performance, followed by the simple average model and bagging with R 2 values of 0.9394 and 0.5998, respectively. From the view of prediction accuracy, and model interpretability, the hybrid physics-ML model with residual modeling is the optimal method for ROP prediction.
更多查看译文
关键词
Prediction of the rate of penetration,Physical model,Machine learning,Hybrid model
AI 理解论文
溯源树
样例
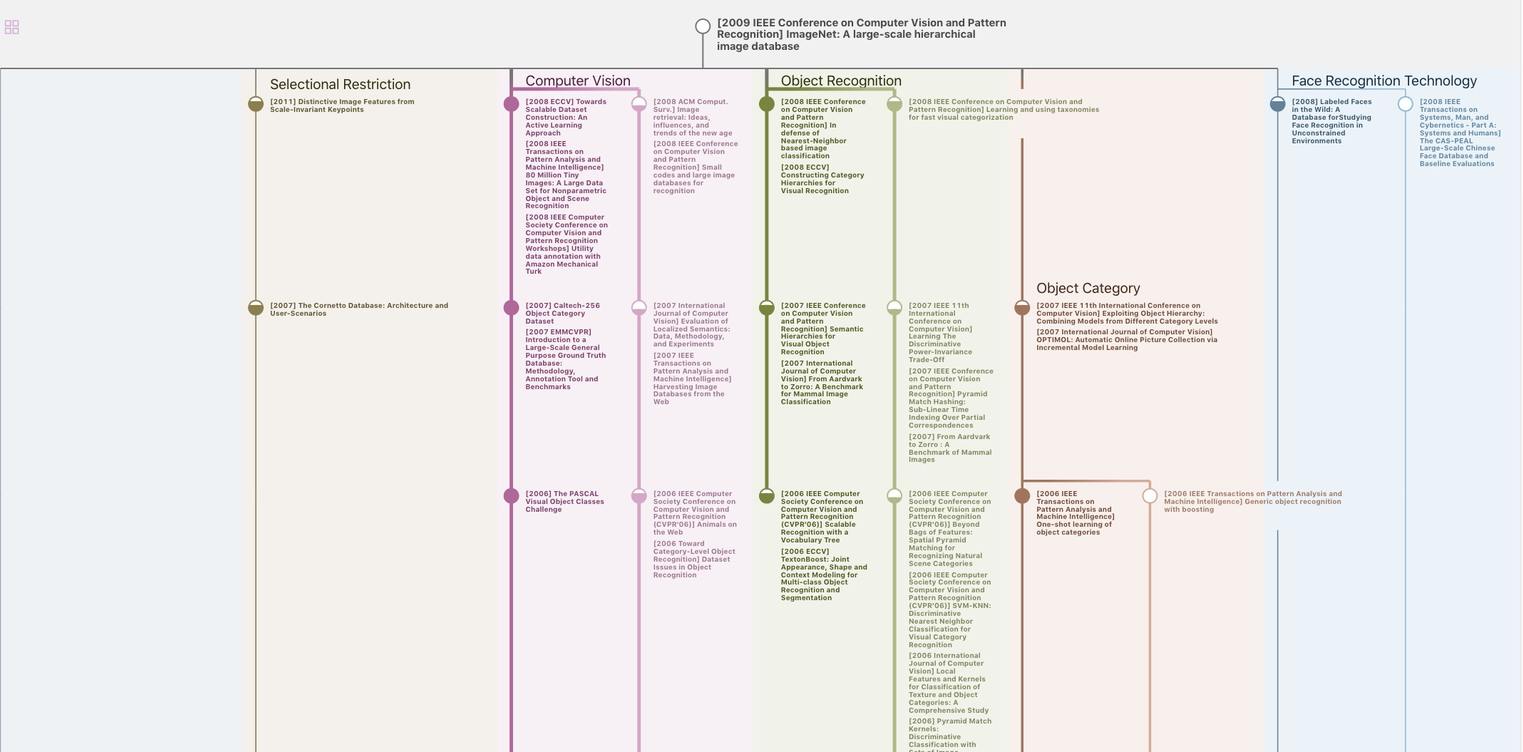
生成溯源树,研究论文发展脉络
Chat Paper
正在生成论文摘要