Understanding Hydrological Model Performance through Variability Analysis of Observed Water Balance Components and Meteorological Forcings
crossref(2024)
摘要
Hydrological modelling forms a pivotal component in assessing water balance closure and providing valuable seasonal forecasts for essential climate variables such as soil moisture and streamflow. In the pursuit of enhancing forecasting capabilities, this study employs four land surface and hydrological models (HTESSEL, JULES, mHM, and PCR-GLOBWB) driven by four distinct meteorological forcings (ERA5LAND, EM-EARTH, MSWEP, and WE5E). The investigation spans the reference period from 1993 to 2019, focusing on a comprehensive evaluation of streamflow, latent heat, runoff flux, and terrestrial water storage as integral components of the water balance equation. The assessment begins by scrutinising the performance of observation datasets globally, aiming to discern areas of robust agreement and potential limitations. Subsequently, the simulations, generated by diverse meteorological forcings, are analysed to gauge the individual skill of each hydrological model and forcing combination.The study then delves into a variability analysis to determine the impact of forcings on hydrological model performance. Furthermore, exploring the elasticity of runoff and streamflow to changes in precipitation adds an additional layer to understanding system dynamics. This multi-faceted approach seeks to quantify the relative contributions of meteorological forcings and hydrological models, providing insights into the intricacies of their interactions and their collective influence on model performance. In conclusion, this research offers a differentiated perspective on the global applicability and performance of these four hydrological models under four meteorological forcings. By systematically assessing the impacts of forcing variability and model structure, the study contributes valuable information for refining hydrological modelling practices and enhancing the accuracy of seasonal forecasts. Observational datasets are inconsistent in certain regions, where no single meteorological forcing stands out as the best performance. These areas are predominantly arid regions such as the Sahara, South Western Australia, and Eastern Brazil, in addition to mountainous regions like the Himalayas, where water balance closure poses a challenge.
更多查看译文
AI 理解论文
溯源树
样例
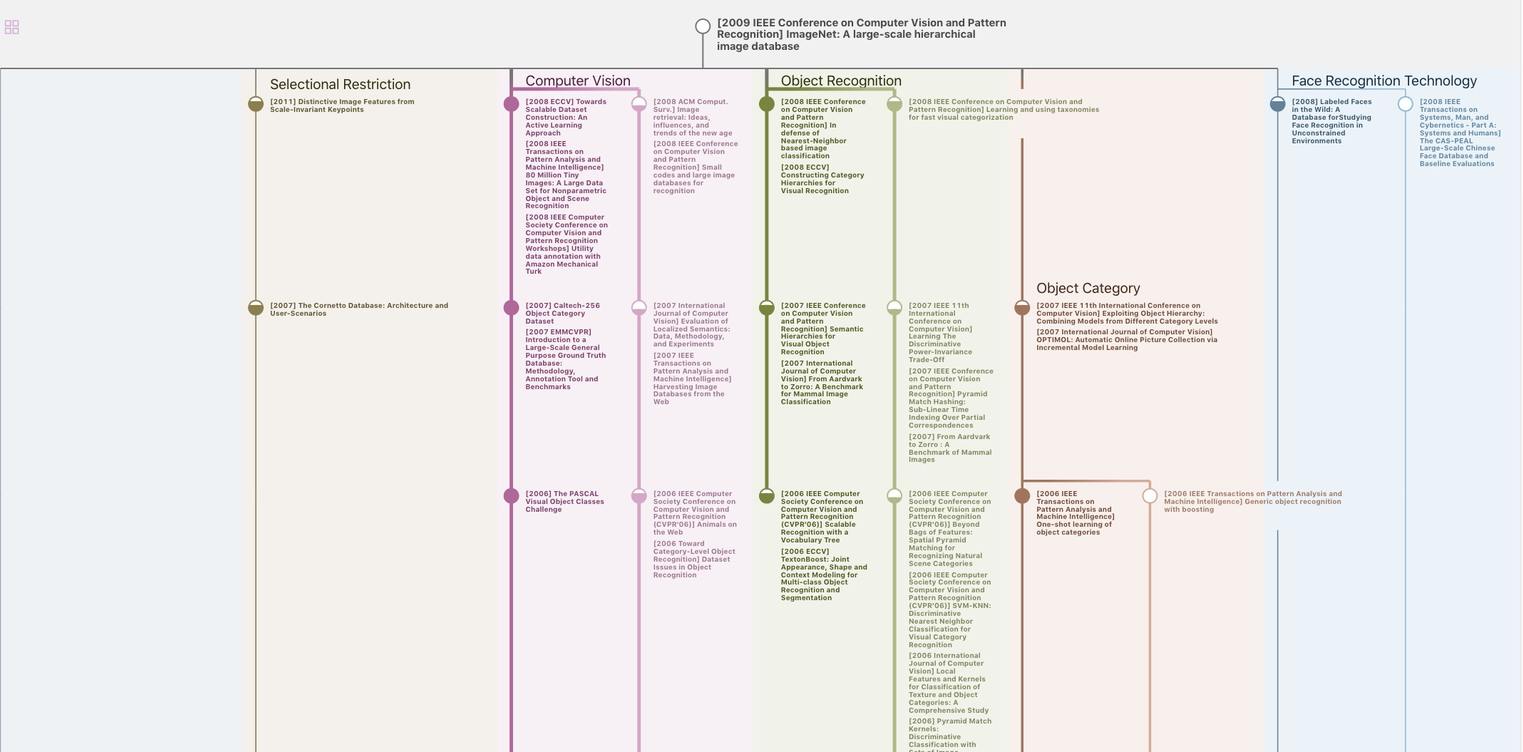
生成溯源树,研究论文发展脉络
Chat Paper
正在生成论文摘要