Backdoor Attacks Leveraging Latent Representation in Competitive Learning
COMPUTER SECURITY. ESORICS 2023 INTERNATIONAL WORKSHOPS, CPS4CIP, PT II(2024)
摘要
Backdoor attacks on machine learning are attacks where an adversary obtains the expected output for a particular input called a trigger, and a previous study which is called latent backdoor attack can resist backdoor removal as their countermeasures, i.e., pruning and transfer learning. In this paper, we present a novel backdoor attack, TALPA, which outperforms the latent backdoor attack with respect to the attack success rate of backdoors as well as keeping the same-level accuracy. The key idea of TALPA is to directly overrides parameters of latent representations in competitive learning between a generative model for triggers and a victim model, and hence can more optimize model parameters and trigger generation than the latent backdoor attack. We demonstrate that TALPA outperforms the latent backdoor attack with respect to the attack success rate and also show that TALPA can resist both pruning and transfer learning through extensive experiments.
更多查看译文
关键词
backdoor attack,pruning,transfer learning,latent representation,competitive learning
AI 理解论文
溯源树
样例
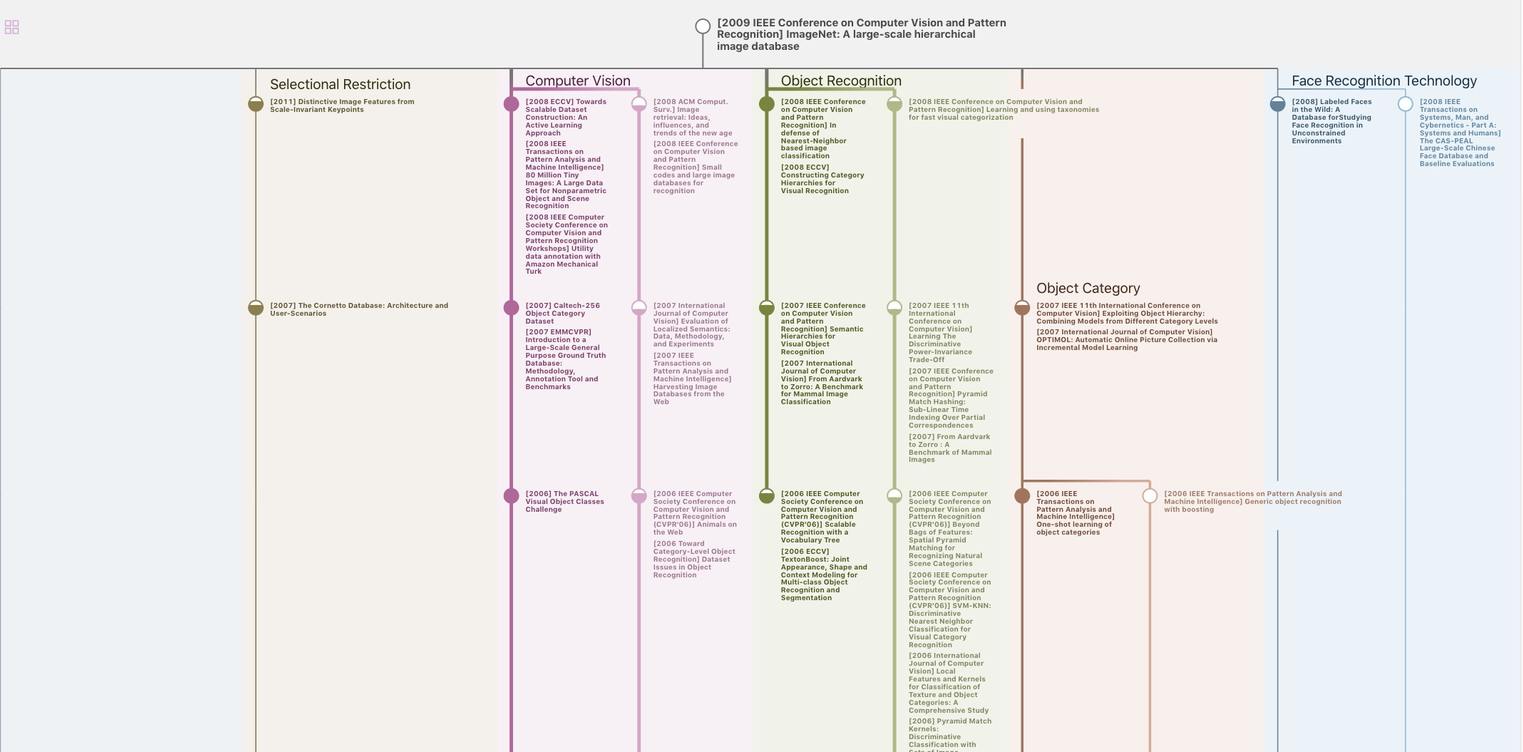
生成溯源树,研究论文发展脉络
Chat Paper
正在生成论文摘要