Object-based characterization of continental Shallow Cumulus Cloud properties using high-resolution Sentinel-2 observations
crossref(2024)
摘要
Shallow convective cumulus clouds (ShCu) play a major role in determining the global radiation balance and the water cycle. However, such clouds are characterized by small-scale spatiotemporal variability that is inadequately represented in observations and climate models, making ShCu a significant contributor to uncertainty in future climate projections. While large observational efforts have been devoted to better understand the mechanisms of marine ShCu in the Tropical trades, continental ShCu has received less attention.Using 7 years of very high-resolution multispectral images with 10x10m2 pixel size acquired by polar-orbiting Copernicus Sentinel-2 satellites we will give the first analysis of these high-resolution observations focused on continental ShCu around the Central Facility of the ARM Southern Great Plains site in the United States. We will show, that the clouds and cloud shadows can be considered as individual objects with associated properties, such as shape and size parameters as well as their geometric relationship, but also as part of an object field. The cloud fraction, cloud-size-distribution and organization index are calculated for various continental ShCu scenes. The influence of large-scale meteorological conditions and surface properties on the cloud fields will be discussed.Furthermore, a technique will be presented for deriving cloud height and cloud thickness from Sentinel-2 observation, which exploits the geometric relationship between cloud objects and their shadows. The satellite-based estimation will be evaluated using ground-based observational data at the ARM Southern Great Plains Facility, such as the Clouds Optically Gridded by Stereo (COGS) 4-D cloud product. We will discuss how cloud height affects the properties of the cloud fields and thus the radiative forcing.
更多查看译文
AI 理解论文
溯源树
样例
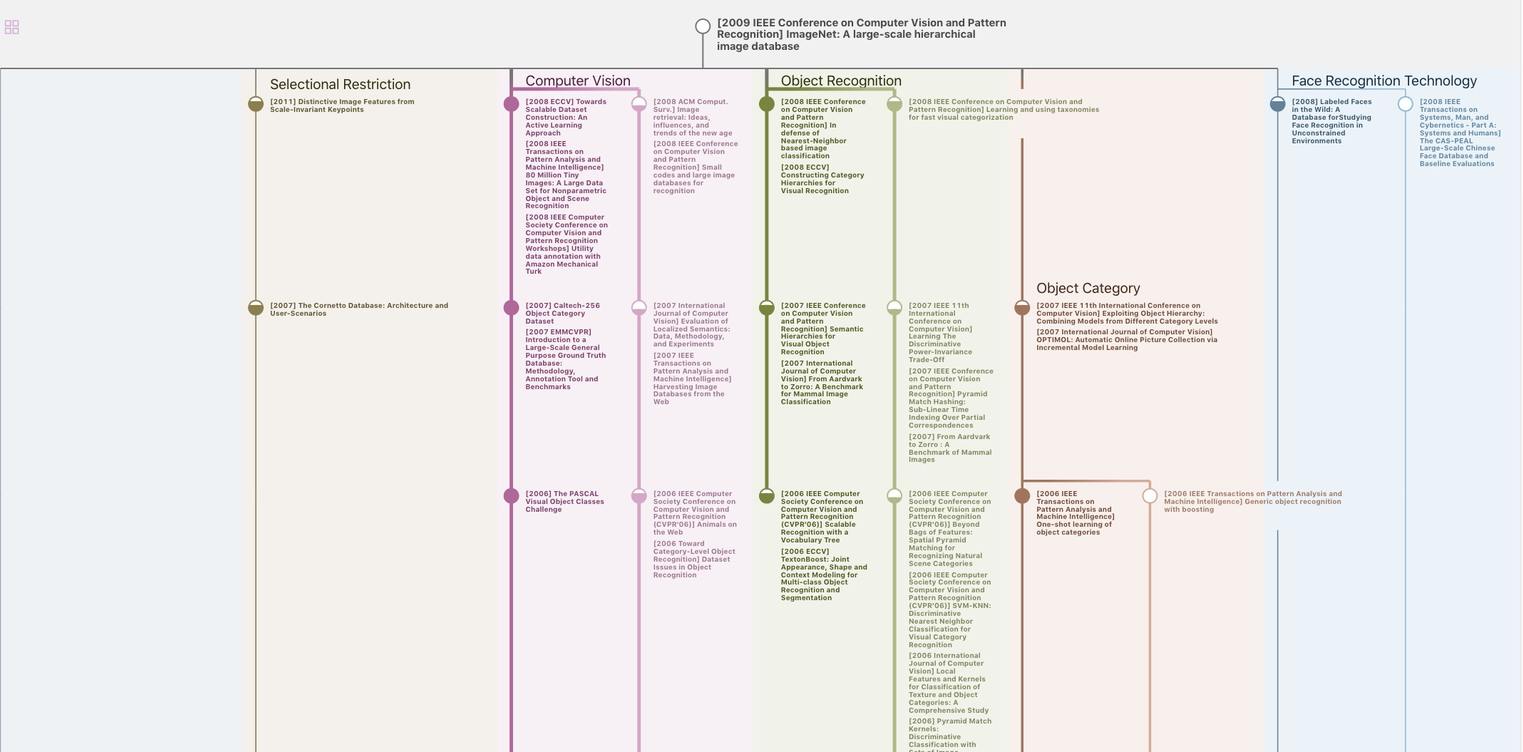
生成溯源树,研究论文发展脉络
Chat Paper
正在生成论文摘要