Open Set Domain Adaptation for Automatic Modulation Classification in Dynamic Communication Environments
IEEE TRANSACTIONS ON COGNITIVE COMMUNICATIONS AND NETWORKING(2024)
关键词
Open-set domain adaptation,modulation classification,dynamic environment,recognition performance,robustness
AI 理解论文
溯源树
样例
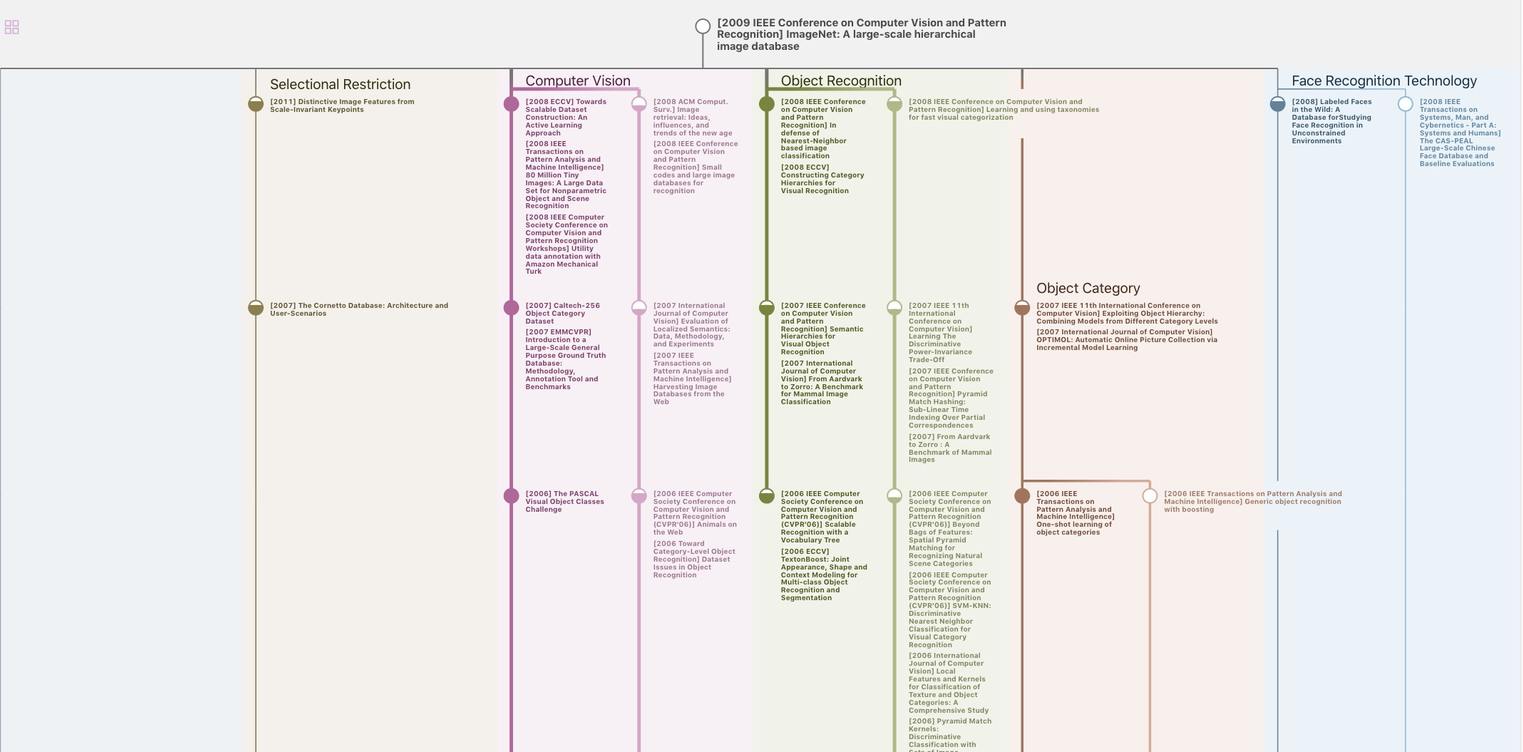
生成溯源树,研究论文发展脉络
Chat Paper
正在生成论文摘要