Multi-sensor analysis of variability in rice transplanting dates in smallholder rice production systems in South Asia
crossref(2024)
摘要
South Asia is one of the world’s rice baskets, with rice production critical to both regional and global food security. However, the region currently experiences significant rice yield gaps and vulnerability to climate change. Timely rice establishment is crucial for rice yields as it ensures phenological phases are synchronised with favourable weather and environmental conditions. In contrast, delayed or premature transplanting time exposes rice crops to adverse conditions such as water and heat stress, and affects the planning of subsequent on-farm activities such as irrigation and fertiliser application. Therefore, understanding spatial and temporal variability in rice transplanting dates is crucial for targeting sustainable intensification interventions to address rice productivity gaps. However, the few existing rice phenology maps for South Asia are based on coarse resolution satellite data that obscures variability at field levels, and generally have not been validated against farm-level data. Our study utilises extensive field data of over 9,600 farm-level rice transplanting date observations collected across Bihar and Uttar Pradesh states in India, which cover an area of about 335,000 km2, and are in the heart of India’s main rice producing region (the Indo-Gangetic Plains or IGP). We explore the ability of an array of satellite sensors (MODIS, Landsat-8, Sentinel-2, and Sentinel-1) with diverse spectral and spatial resolutions to capture and map the spatio-temporal variability in rice transplanting in this region. Utilising Google Earth Engine, we extract phenological time series of relevant indices and metrics such as NDVI, EVI, NDFI, and LSWI, and backscatter coefficients for the 2017 and 2018 rice growing seasons from optical and radar data, respectively. We apply machine learning techniques, specifically random forest models, developed separately for each sensor, along with models that integrate optical and radar data to estimate rice transplanting dates based on these phenological time-series metrics. Satellite-based estimates of transplanting dates are validated against field data and accuracy assessment is conducted to demonstrate the potential of improved estimation of rice phenology using multi-sensor data. Subsequently, the best model and sensor-combination is applied to the entire Eastern Indo-Gangetic Plain of India (EIGP) to evaluate the spatial and temporal variations of the rice transplanting. We demonstrate that random forests models can provide robust estimates of transplanting dates, overcoming some of the challenges associated with traditional rule or curve-based crop phenology estimation. We find complex patterns of variability in rice transplanting dates within our study area in the eastern IGP indicated by nuanced changes in rice fields during the transplanting period, reflecting both climatological factors (e.g. monsoon onset timing) and multifaceted socio-economic conditions on the ground for farmers (e.g. varying levels of irrigation access). Our results can help to identify areas prone to delayed transplanting, and help to target investigation to identify underlying factors influencing these patterns. This will pave the way for developing targeted interventions to support farmers in making informed decisions during the transplanting period, thereby forming the basis for enhanced productivity, adaptation to changing climate, and sustainable rice management practices in the region.
更多查看译文
AI 理解论文
溯源树
样例
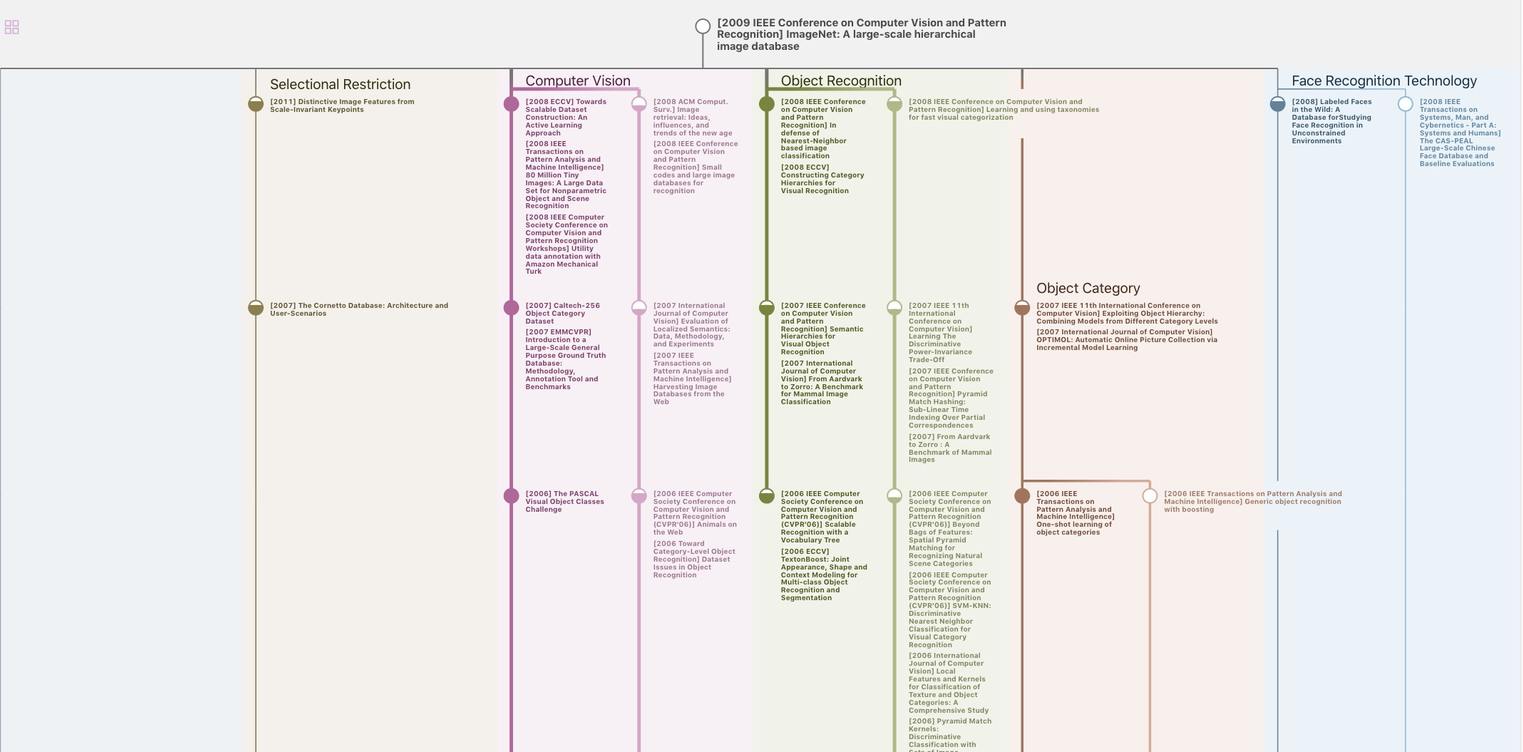
生成溯源树,研究论文发展脉络
Chat Paper
正在生成论文摘要