Geometrically-Regularized Fast Independent Vector Extraction by Pure Majorization-Minimization
IEEE Transactions on Signal Processing(2024)
摘要
We propose computationally efficient algorithms for extracting a single source of interest (SOI) using geometrically-regularized independent vector extraction (GR-IVE). Conventional GR-IVE relies on a block majorization-minimization (block MM) algorithm, which successively optimizes each part (block) of the separation matrix based on the minimization of a surrogate function. We here extend the block MM to a pure MM by developing a global optimization algorithm for minimizing the same surrogate function when the number of SOI is one. Our global optimization is reduced to finding the minimum solution of the so-called secular equation. We also study an orthonormal approach to GR-IVE, i.e., when the feasible region is restricted to unitary matrices. For this problem, we also develop a pure MM algorithm for the single SOI case. Numerical experiments for audio source extraction demonstrate that our proposed MM algorithms converge consistently faster than the conventional block MM algorithm for GR-IVE. Also, the proposed orthonormal approach improves the separation accuracy if the regularization parameter is chosen appropriately.
更多查看译文
关键词
Independent component analysis (ICA),independent vector analysis (IVA),source separation,majorization-minimization (MM),secular equation
AI 理解论文
溯源树
样例
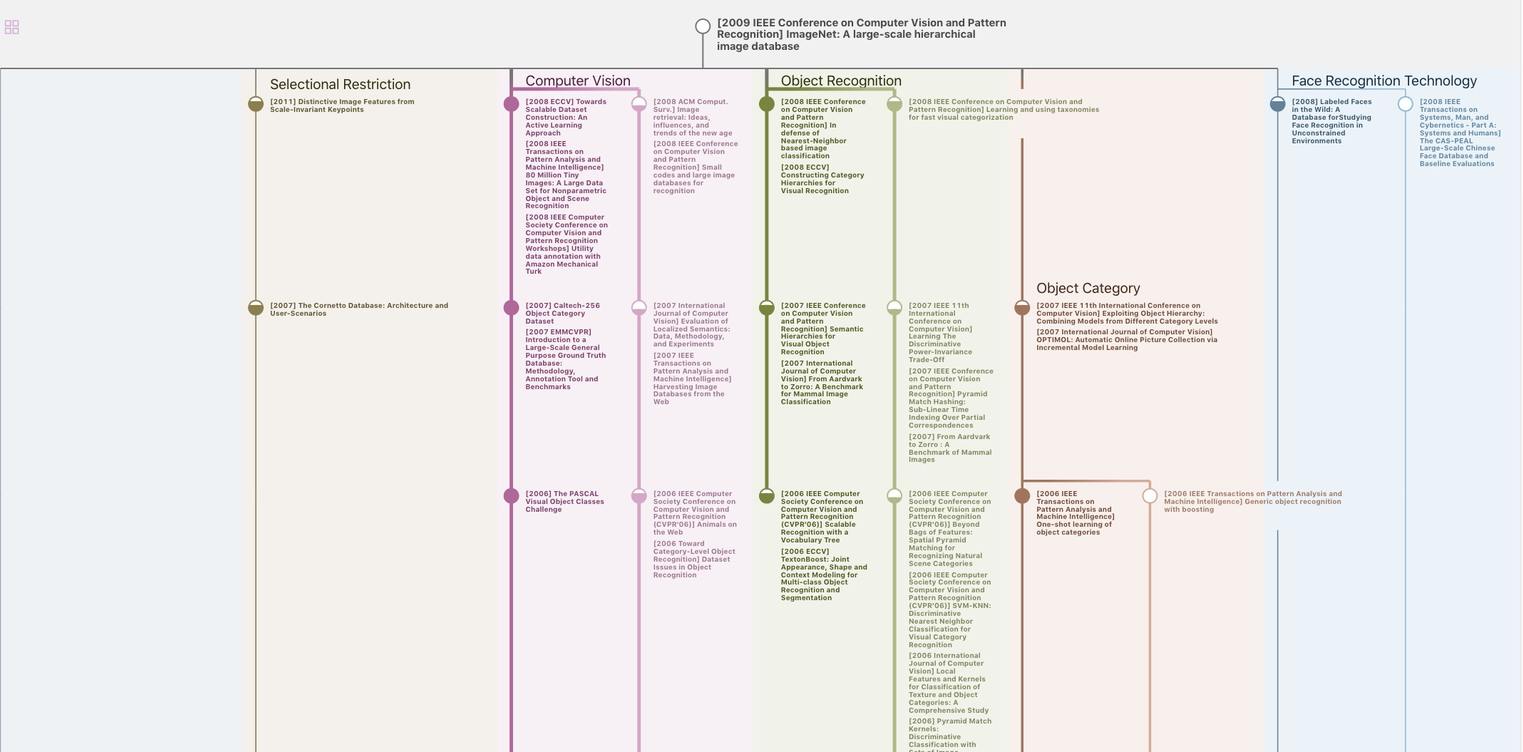
生成溯源树,研究论文发展脉络
Chat Paper
正在生成论文摘要