Multi-scale Structural Graph Convolutional Network for Skeleton-based Action Recognition
IEEE Transactions on Circuits and Systems for Video Technology(2024)
摘要
Graph convolutional networks (GCNs) have attracted considerable interest in skeleton-based action recognition. Existing GCN-based models have proposed methods to learn dynamic graph topologies generated from the feature information of vertices to capture inherent relationships. However, these models have two main limitations. Firstly, they struggle to effectively utilize high-dimensional or structural information, which limits their capacity for feature representation and consequently hinders performance improvement. Secondly, among these models, the multi-scale methods that aggregate information at different scales often over-capture unnecessary relationships between vertices. This leads to an over-smoothing problem where smoothed features are extracted, making it difficult to distinguish the features of each vertex. To address these limitations, we propose the multi-scale structural graph convolutional network (MSS-GCN) for skeleton-based action recognition. Within the MSS-GCN framework, the common intersection graph convolution (CI-GC) leverages the overlapped neighbor information, indicating the overlap between neighboring vertices for a given pair of root vertices. The graph topology of CI-GC is designed to compute the structural correlation between neighboring vertices corresponding to each hop, thereby enriching the context of inter-vertex relationships. Then, our proposed multi-scale spatio-temporal modeling aggregates local-global features to provide a comprehensive representation. In addition, we propose a Graph Weight Annealing (GWA) method, which is a graph scheduling method to mitigate the over-smoothing caused by multi-scale aggregation. By varying the importance between a vertex and its neighbors, we demonstrate that the over-smoothing problem can be effectively mitigated. Moreover, our proposed GWA method can easily be adapted to different GCN models to enhance performance. Combining the MSS-GCN model and the GWA method, we propose a powerful feature extractor that effectively classifies actions for skeleton-based action recognition in various datasets. We evaluate our approach on three benchmark datasets: NTU RGB+D, NTU RGB+D 120, and NW-UCLA. The proposed MSS-GCN achieves state-of-the-art performance on all three datasets, further validating the effectiveness of our approach.
更多查看译文
关键词
Skeleton-based Action Recognition,Graph Convolutional Network,Link Prediction
AI 理解论文
溯源树
样例
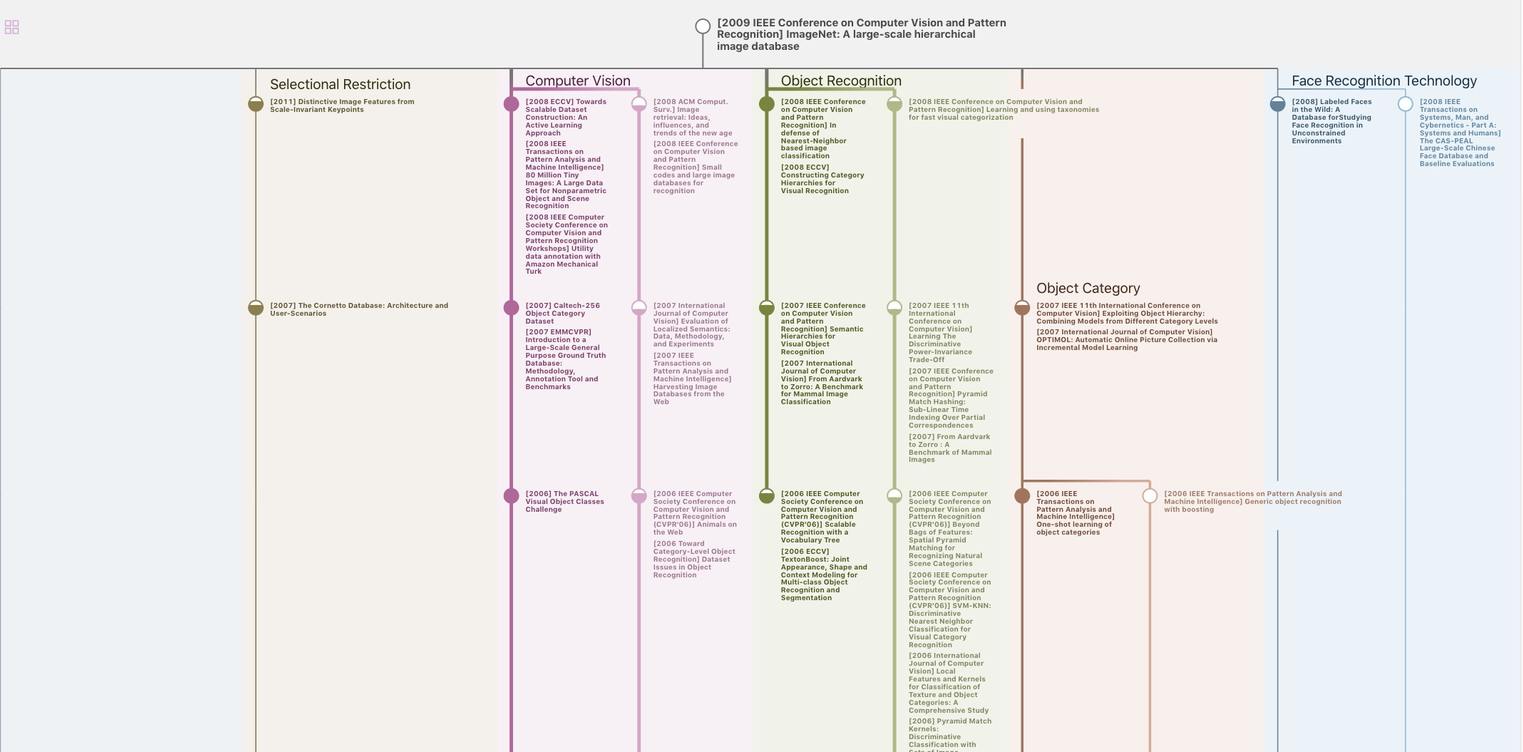
生成溯源树,研究论文发展脉络
Chat Paper
正在生成论文摘要