Divergent Responses of Summer Terrestrial Evapotranspiration to Cloud Increase in East Asia
JOURNAL OF GEOPHYSICAL RESEARCH-ATMOSPHERES(2024)
摘要
Cloud impact on terrestrial evapotranspiration (ET) can be significant, while a fundamental understanding of ET response to cloud change has not been addressed in regional aspects. In this study, seven ET datasets from satellite and land surface models are used to analyze the summer ET in response to low cloud cover (LCC) over East Asia, in combination with satellite microwave and optical vegetation data, as well as ERA5 climatic and surface parameters. In general, all ET datasets present a strong negative relationship with LCC over dense vegetation (e.g., forests), which leads to the ET reduction of more than -0.5 mmday(-1) under the large LCC increase, compared with the least cloudy sky conditions. Such reduction is stronger under more humid and denser forests (aridity index AI > 0.5 and enhanced vegetation index EVI > 0.6). In contrast, a positive relationship between ET and LCC presents over arid and sparse lands (AI < 0.2 and EVI < 0.2), accompanied by enhanced ET (>0.2 mmday(-1)). Analysis shows that under increased LCC conditions, substantial reduction in radiation availability results in the decline in both plant transpiration (ETveg) and soil evaporation (ETsoil) over humid dense vegetation, dominating the overall negative response. The increase of near-surface relative humidity over arid sparse vegetation may indicate a stronger moisture availability, which promotes ETsoil and partly offsets the negative effect of radiation reduction. The change of vegetation water content, indicated by a satellite microwave vegetation index, is important for ETveg over arid mountainous lands with cloud cover. These findings contribute new insights to the understanding of vegetation-atmosphere interactions.
更多查看译文
关键词
evapotranspiration,cloud change,satellite microwave remote sensing,vegetation water content,East Asia
AI 理解论文
溯源树
样例
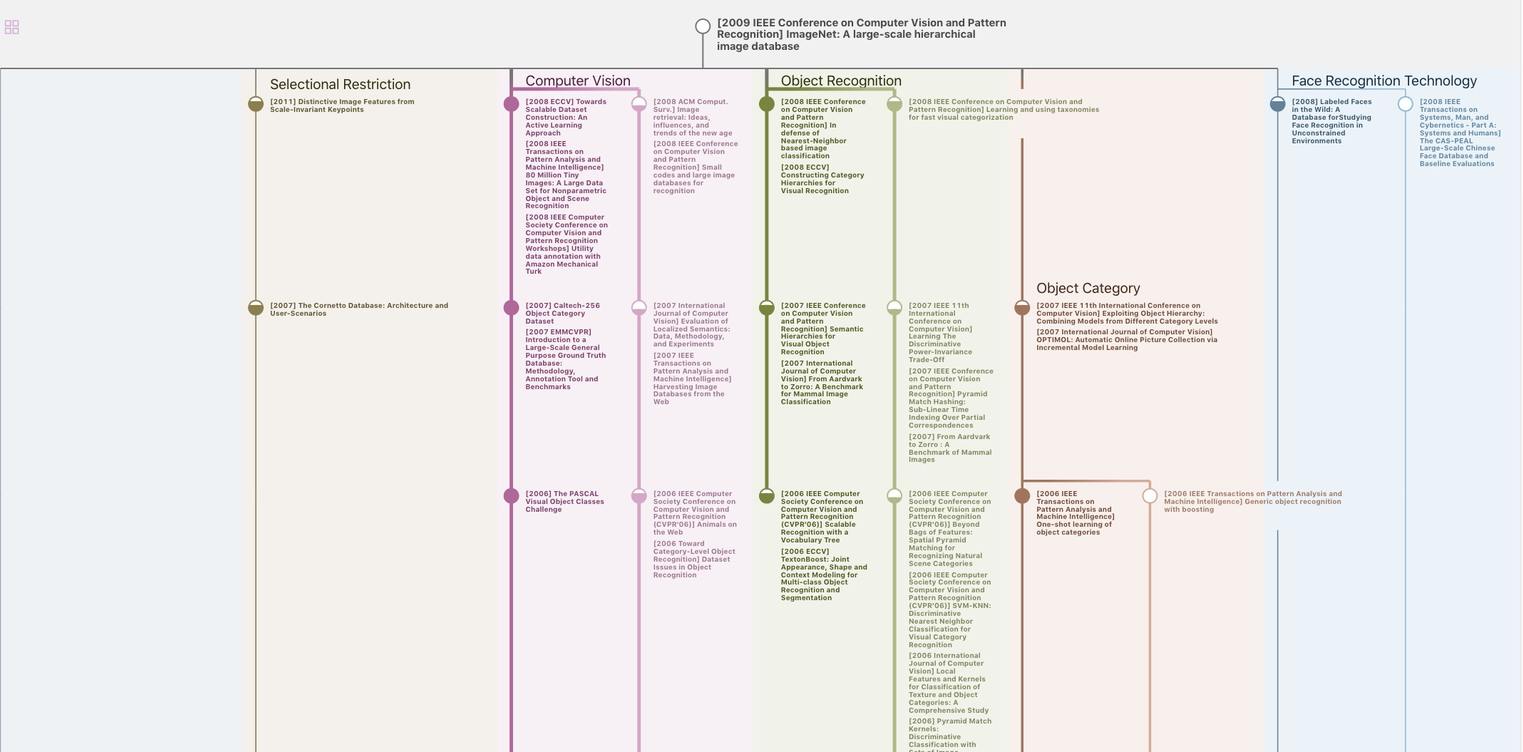
生成溯源树,研究论文发展脉络
Chat Paper
正在生成论文摘要