Snowpack Stability Prediction with Machine Learning
crossref(2024)
摘要
Snow avalanches are destructive hazards, impacting life and infrastructure in snow-covered mountain regions around the world. Avalanche warning and management are essential in those regions and involve a diversity of tasks, ranging from numerical modeling of regional avalanche danger, search and rescue of avalanche victims, to artificially triggering avalanches with explosives in a controlled way. Avalanche warning systems rely on in-situ point measurements of snowpack stability in conjunction with numerical models, to predict the avalanche risk at different exposition and height levels. Avalanche teams are performing those in-situ measurements involving various time-intensive tests, such as Rutschblock tests (RB) and manual snow profiles. There are faster measurement approaches, such as using the Snow Micro Pen (SMP), but snowpack stability scores cannot directly be derived from an SMP profile. Machine learning (ML) has supported and improved numerical modeling and forecasting of regional avalanche danger in multiple ways. However, the question if ML can assist in-situ assessments of snowpack stability, remains, to our knowledge, unanswered. In order to retrieve faster and more intuitive assessments, we are proposing to use ML to directly predict snowpack stability scores from SMP profiles. Our ML pipeline 1) is flexible and can be adapted for other measurement devices, 2) allows training to retrieve further physical parameters, and 3) allows the integration of explainability methods. Furthermore, we outline criteria relevant to collecting the data needed for training the ML model. For safe operational use, training on large datasets, uncertainty estimates, and explainability must be addressed. We believe that our approach represents a significant step towards assisting SMP practitioners in the analysis of snowpack stability. We also see the potential for coupling regional avalanche forecasting systems with in-situ snowpack stability estimates further down the line. If done correctly, we believe that ML can be a useful tool to assist in managing in-situ avalanche risk.
更多查看译文
AI 理解论文
溯源树
样例
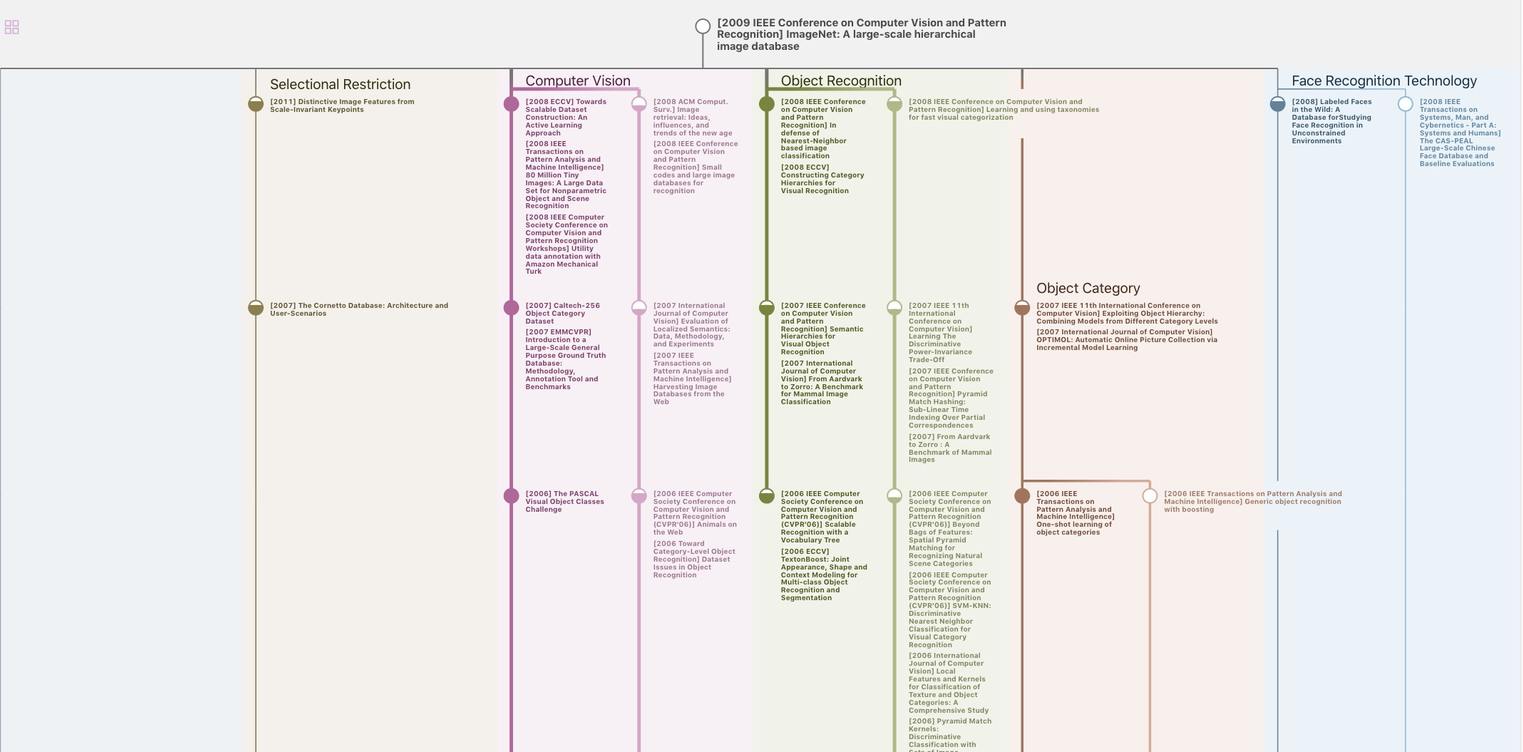
生成溯源树,研究论文发展脉络
Chat Paper
正在生成论文摘要