A Deep Learning Approach to Merge Rule-Based and Human-Operated Camera Control for Teleoperated Robotic Systems
ROBOTICS(2024)
摘要
Controlling a laparoscopic camera during robotic surgery represents a multifaceted challenge, demanding considerable physical and cognitive exertion from operators. While manual control presents the advantage of enabling optimal viewing angles, it is offset by its taxing nature. In contrast, current autonomous camera systems offer predictability in tool tracking but are often rigid, lacking the adaptability of human operators. This research investigates the potential of two distinct network architectures: a dense neural network (DNN) and a recurrent network (RNN), both trained using a diverse dataset comprising autonomous and human-driven camera movements. A comparative assessment of network-controlled, autonomous, and human-operated camera systems is conducted to gauge network efficacies. While the dense neural network exhibits proficiency in basic tool tracking, it grapples with inherent architectural limitations that hinder its ability to master the camera's zoom functionality. In stark contrast, the recurrent network excels, demonstrating a capacity to sufficiently replicate the behaviors exhibited by a mixture of both autonomous and human-operated methods. In total, 96.8% of the dense network predictions had up to a one-centimeter error when compared to the test datasets, while the recurrent network achieved a 100% sub-millimeter testing error. This paper trains and evaluates neural networks on autonomous and human behavior data for camera control.
更多查看译文
关键词
robotic surgery,deep neural network,autonomous camera control
AI 理解论文
溯源树
样例
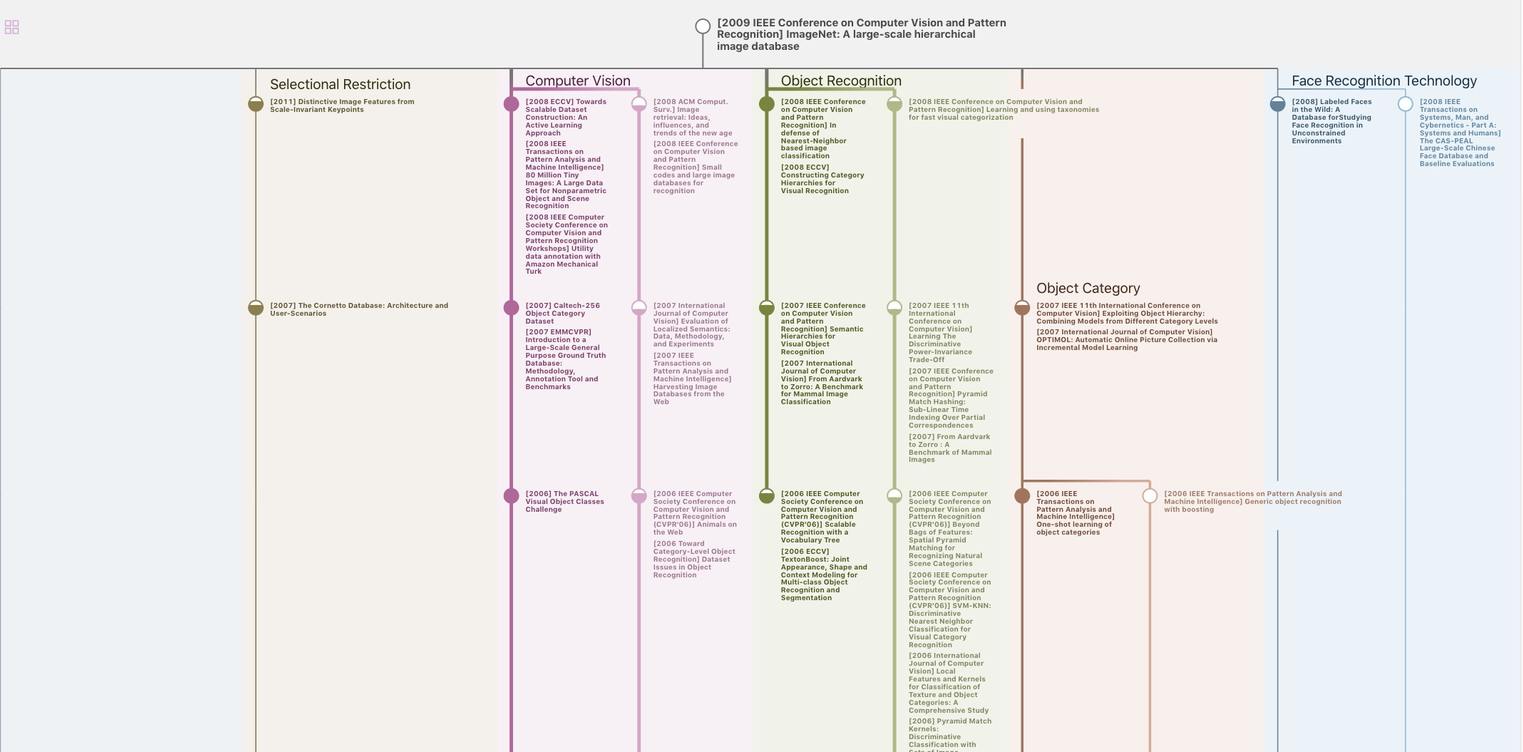
生成溯源树,研究论文发展脉络
Chat Paper
正在生成论文摘要