Flexible Constraints-Based Adaptive Intelligent Event-Triggered Control for Slowly Switched Nonlinear Systems Using Reinforcement Learning
IEEE Transactions on Artificial Intelligence(2024)
摘要
In this note, an adaptive event-triggered optimized tracking control problem is investigated for nonlinear switched systems with flexible output constraints under extended mode-dependent average dwell time (MDADT). Initially, a new shifting function and an improved barrier function are constructed to solve flexible output constraints under the practical background. Subsequently, a global performance function with the exponential discount factor based on the error variable is designed under optimized backstepping (OB), which not only ensures that the performance function converges, but also evaluates the tracking performance of the system, reflecting the energy consumption. The corresponding Hamilton-Jacobi-Bellman (HJB) equation is constructed to solve the optimal control strategy. To remove the restriction on the maximum asynchronous time, an event-triggered optimization strategy for subsystems is utilized to exclude Zeno behavior. Furthermore, we demonstrate that the signals of the closed-loop system are bounded and the flexible output constraints are strictly obeyed. Finally, the application of the above control technique to the manipulator system is validated.
更多查看译文
关键词
Switched nonlinear system,neural network,event-triggered mechanism,flexible output constraints,reinforcement learning
AI 理解论文
溯源树
样例
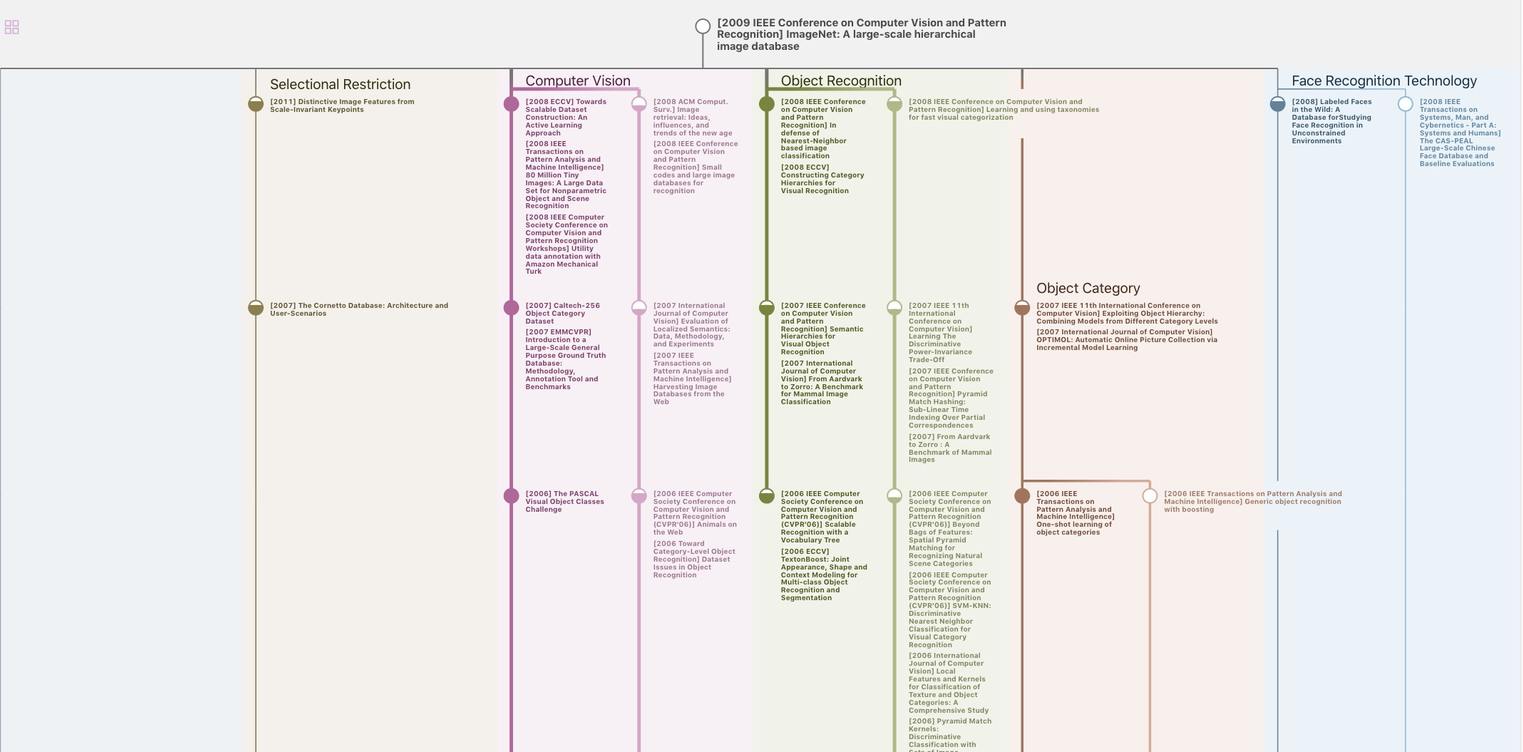
生成溯源树,研究论文发展脉络
Chat Paper
正在生成论文摘要