Reliable Spatial-Temporal Voxels For Multi-Modal Test-Time Adaptation
CoRR(2024)
Abstract
Multi-modal test-time adaptation (MM-TTA) is proposed to adapt models to an
unlabeled target domain by leveraging the complementary multi-modal inputs in
an online manner. Previous MM-TTA methods rely on predictions of cross-modal
information in each input frame, while they ignore the fact that predictions of
geometric neighborhoods within consecutive frames are highly correlated,
leading to unstable predictions across time. To fulfill this gap, we propose
ReLiable Spatial-temporal Voxels (Latte), an MM-TTA method that leverages
reliable cross-modal spatial-temporal correspondences for multi-modal 3D
segmentation. Motivated by the fact that reliable predictions should be
consistent with their spatial-temporal correspondences, Latte aggregates
consecutive frames in a slide window manner and constructs ST voxel to capture
temporally local prediction consistency for each modality. After filtering out
ST voxels with high ST entropy, Latte conducts cross-modal learning for each
point and pixel by attending to those with reliable and consistent predictions
among both spatial and temporal neighborhoods. Experimental results show that
Latte achieves state-of-the-art performance on three different MM-TTA
benchmarks compared to previous MM-TTA or TTA methods.
MoreTranslated text
AI Read Science
Must-Reading Tree
Example
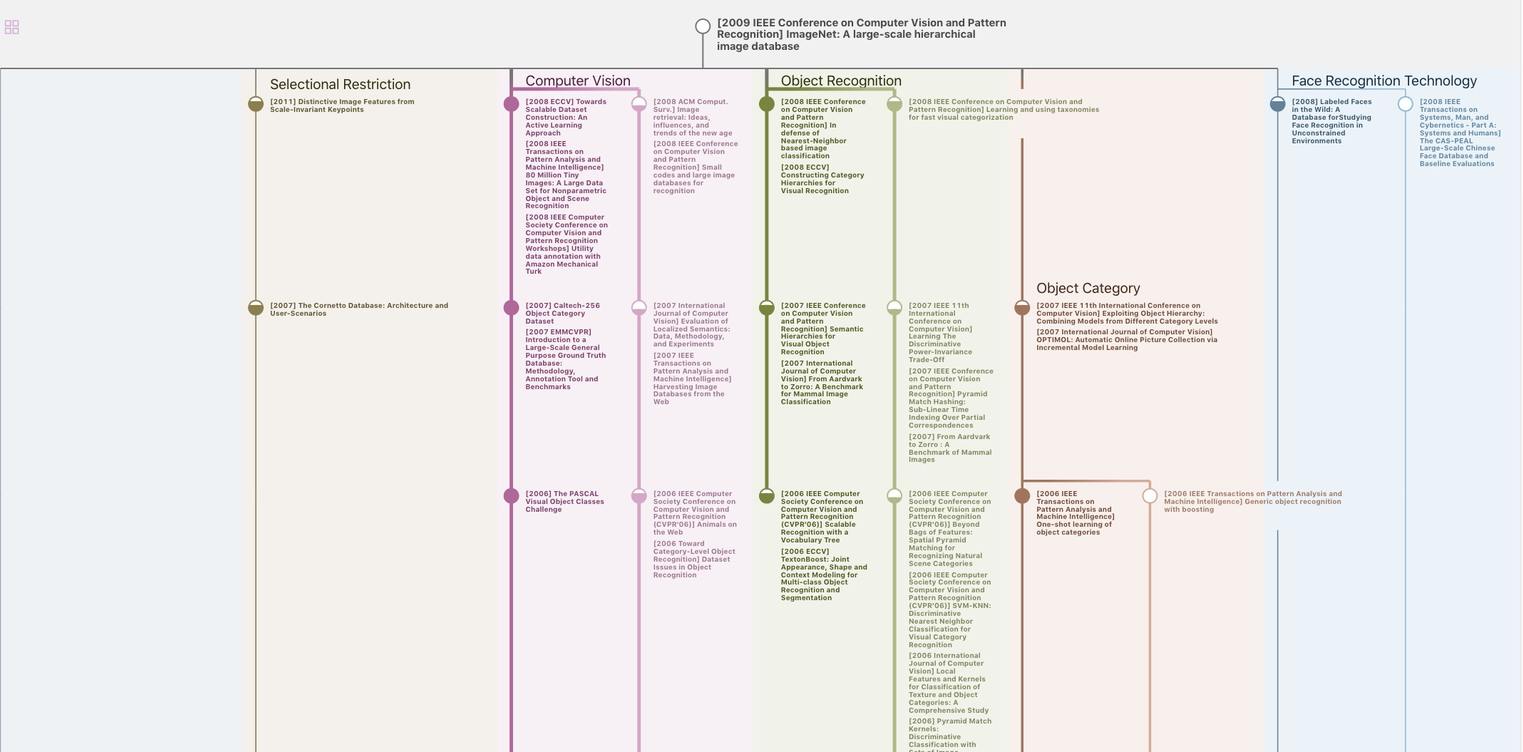
Generate MRT to find the research sequence of this paper
Chat Paper
Summary is being generated by the instructions you defined