Online Identification of Stochastic Continuous-Time Wiener Models Using Sampled Data
CoRR(2024)
摘要
It is well known that ignoring the presence of stochastic disturbances in the
identification of stochastic Wiener models leads to asymptotically biased
estimators. On the other hand, optimal statistical identification, via
likelihood-based methods, is sensitive to the assumptions on the data
distribution and is usually based on relatively complex sequential Monte Carlo
algorithms. We develop a simple recursive online estimation algorithm based on
an output-error predictor, for the identification of continuous-time stochastic
parametric Wiener models through stochastic approximation. The method is
applicable to generic model parameterizations and, as demonstrated in the
numerical simulation examples, it is robust with respect to the assumptions on
the spectrum of the disturbance process.
更多查看译文
关键词
Stochastic Model,Model Identification,Online Identification,Wiener Model,Model Parameters,Numerical Simulations,Numerical Examples,Simulation Example,Recursive Algorithm,Stochastic Approximation,Continuous-time Model,Square Root,Prediction Error,Linear System,Nonlinear Systems,Input Signal,Model Plant,Measurement Noise,Independent Processes,Convergence Of Algorithm,Disturbance Model,Stochastic Differential Equations,Transfer Operator,Stochastic Integral,Irregular Time,Hill Coefficient,True Parameter,Single-input Single-output
AI 理解论文
溯源树
样例
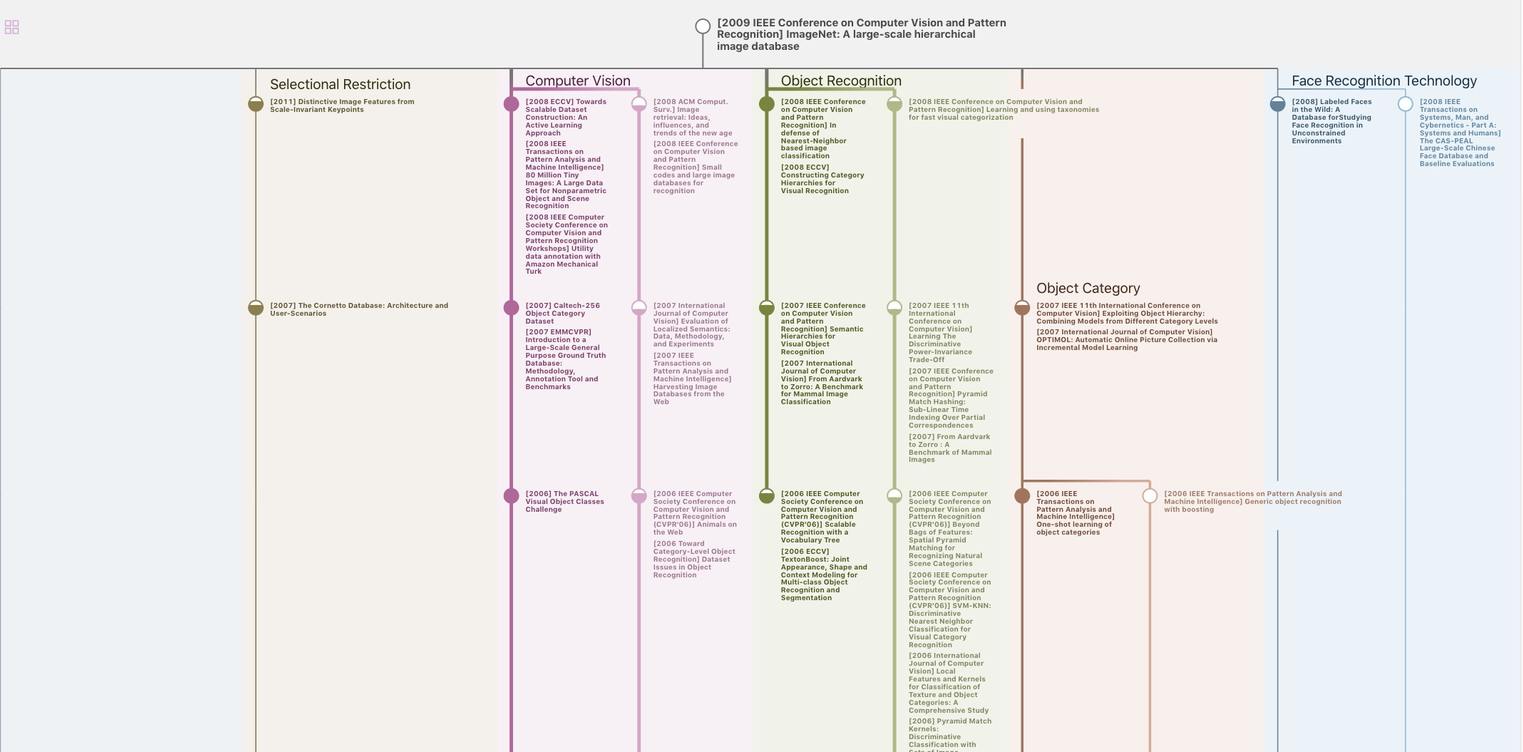
生成溯源树,研究论文发展脉络
Chat Paper
正在生成论文摘要