Shielded Deep Reinforcement Learning for Complex Spacecraft Tasking
CoRR(2024)
摘要
Autonomous spacecraft control via Shielded Deep Reinforcement Learning (SDRL)
has become a rapidly growing research area. However, the construction of
shields and the definition of tasking remains informal, resulting in policies
with no guarantees on safety and ambiguous goals for the RL agent. In this
paper, we first explore the use of formal languages, namely Linear Temporal
Logic (LTL), to formalize spacecraft tasks and safety requirements. We then
define a manner in which to construct a reward function from a co-safe LTL
specification automatically for effective training in SDRL framework. We also
investigate methods for constructing a shield from a safe LTL specification for
spacecraft applications and propose three designs that provide probabilistic
guarantees. We show how these shields interact with different policies and the
flexibility of the reward structure through several experiments.
更多查看译文
AI 理解论文
溯源树
样例
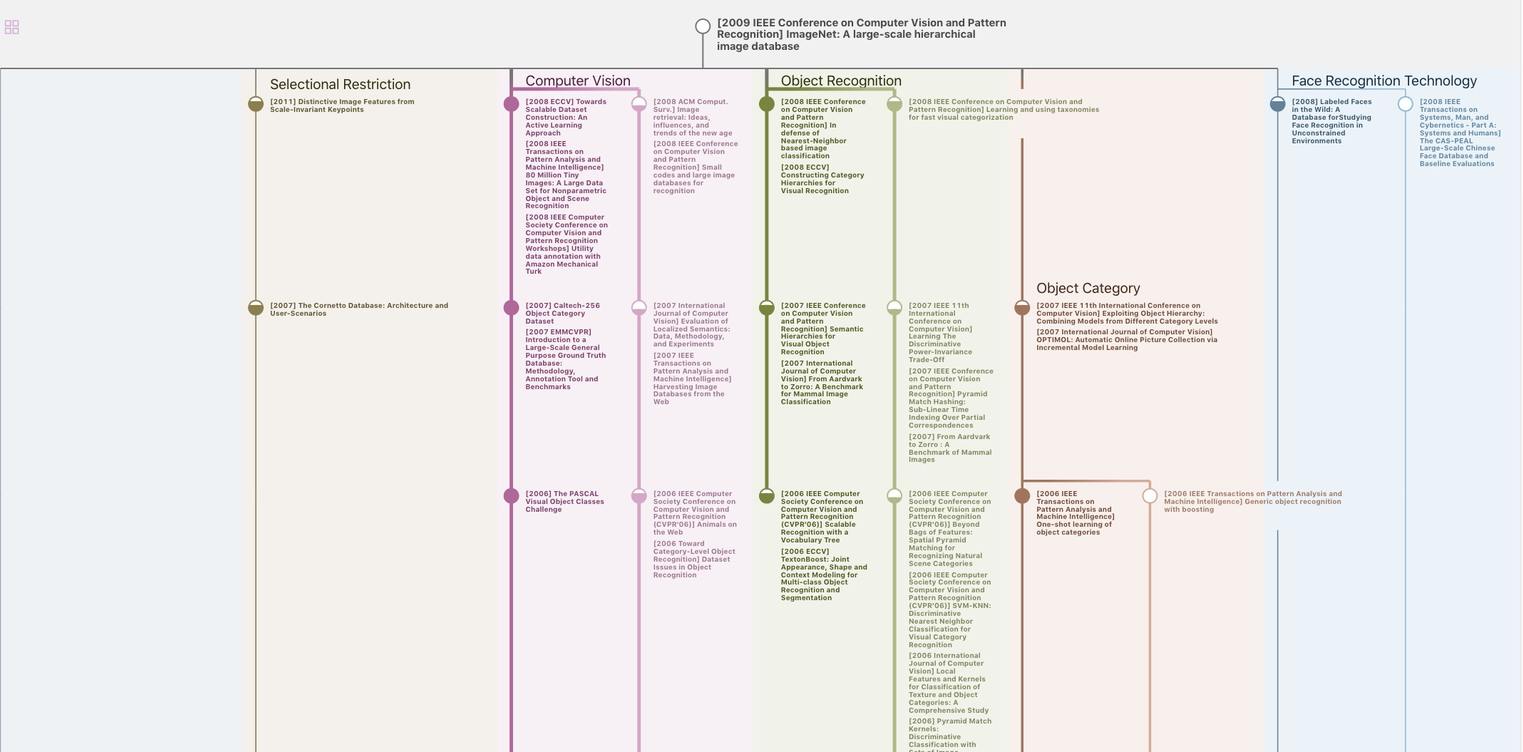
生成溯源树,研究论文发展脉络
Chat Paper
正在生成论文摘要