Privacy-Preserving Sharing of Data Analytics Runtime Metrics for Performance Modeling
Companion of the 15th ACM/SPEC International Conference on Performance Engineering(2024)
摘要
Performance modeling for large-scale data analytics workloads can improve the
efficiency of cluster resource allocations and job scheduling. However, the
performance of these workloads is influenced by numerous factors, such as job
inputs and the assigned cluster resources. As a result, performance models
require significant amounts of training data. This data can be obtained by
exchanging runtime metrics between collaborating organizations. Yet, not all
organizations may be inclined to publicly disclose such metadata.
We present a privacy-preserving approach for sharing runtime metrics based on
differential privacy and data synthesis. Our evaluation on performance data
from 736 Spark job executions indicates that fully anonymized training data
largely maintains performance prediction accuracy, particularly when there is
minimal original data available. With 30 or fewer available original data
samples, the use of synthetic training data resulted only in a one percent
reduction in performance model accuracy on average.
更多查看译文
AI 理解论文
溯源树
样例
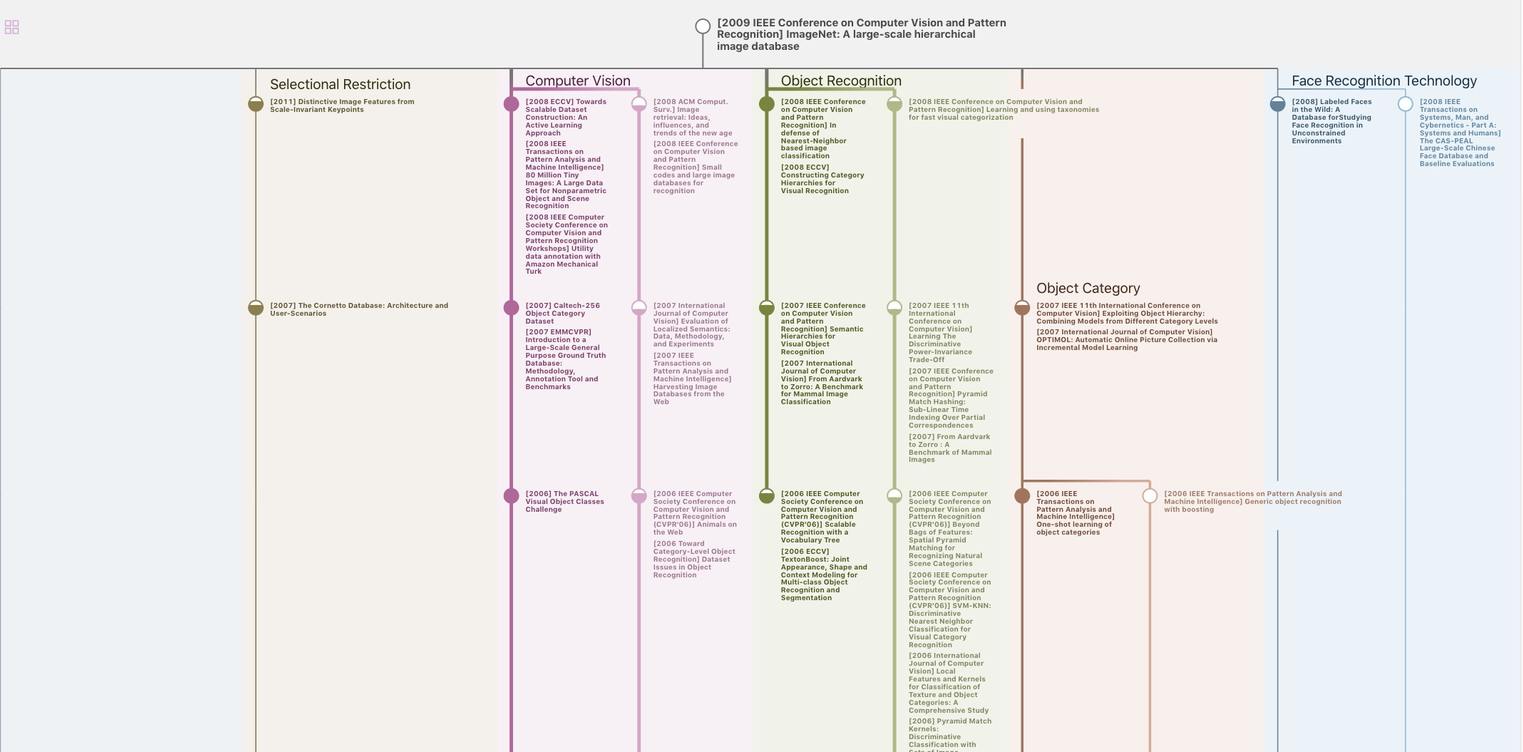
生成溯源树,研究论文发展脉络
Chat Paper
正在生成论文摘要