Optimizing production logistics through advanced machine learning techniques: A study on resource allocation for small-batch and multi-variety challenges
PROCEEDINGS OF THE INSTITUTION OF MECHANICAL ENGINEERS PART E-JOURNAL OF PROCESS MECHANICAL ENGINEERING(2024)
摘要
In light of increasing consumer purchasing power and the growing diversification of demands, the emergence of small-batch and multi-variety requirements has posed substantial challenges to production manufacturing, particularly in on-site production logistics management. This study aims to address the issues of effective material flow and resource allocation in production logistics management by investigating a method for the rapid and accurate allocation of supply logistics resources. Leveraging the extensive logistics resource allocation data available in the Plan For Every Part system, this research utilizes the Term Frequency-Inverse Document Frequency algorithm to filter out less discriminative data. Additionally, a recommender system based on machine learning, specifically the Recsys (Recommender System) employing similarity algorithms, is employed to construct a logistics resource allocation recommendation model. The effectiveness of this approach is validated through a case study that involves material resource allocation recommendations in a specific remote control production workshop. The validation results indicate that, compared to traditional logistics resource allocation processes, the proposed model optimizes resource allocation recommendations in production logistics, resulting in a significant improvement in material delivery punctuality and overall operational efficiency in enterprises.
更多查看译文
关键词
Production manufacturing challenges,material flow,resource allocation,supply logistics resources,machine learning,logistics resource allocation model
AI 理解论文
溯源树
样例
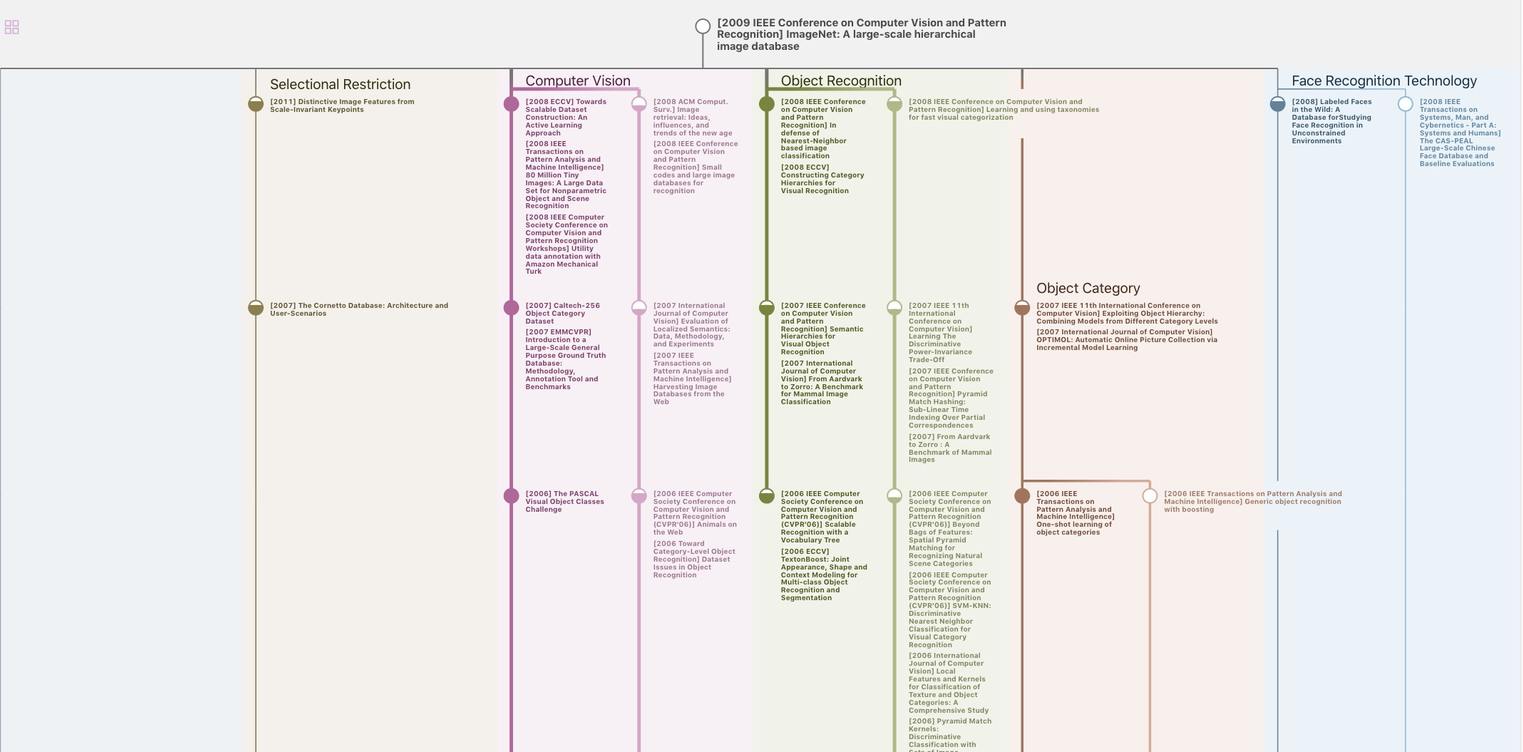
生成溯源树,研究论文发展脉络
Chat Paper
正在生成论文摘要