Energy-efficient craters detection based on spiking neural network using digital elevation models
Expert Systems with Applications(2024)
摘要
Craters are the primary topographic features on celestial bodies and play a vital role in deep space exploration missions. Recently, artificial neural networks (ANNs) have excelled in crater detection. However, when compared to ANNs, spike neural networks (SNNs) have the advantage of low power consumption, making them more suitable for resource-limited deep space environments. To the best of our knowledge, this is the first spiked-based crater detection model. Firstly, two conversion learning algorithms based on cluster neurons and vary-time windows neurons are proposed to address the non-differentiability problem of SNNs. Secondly, a calibration mechanism is introduced to correct the conversion loss between ANNs and SNNs. Thirdly, the experimental results demonstrate that the proposed scheme exhibits sufficient precision, while reducing the energy consumption cost by more than 10 times compared to the previous method. Additionally, this method is expected to offer new insights for segmentation tasks in resource-constrained environments.
更多查看译文
关键词
Crater detection algorithm,Spike neural network,Deep space exploration,ANN-to-SNN conversion,Digital elevation models
AI 理解论文
溯源树
样例
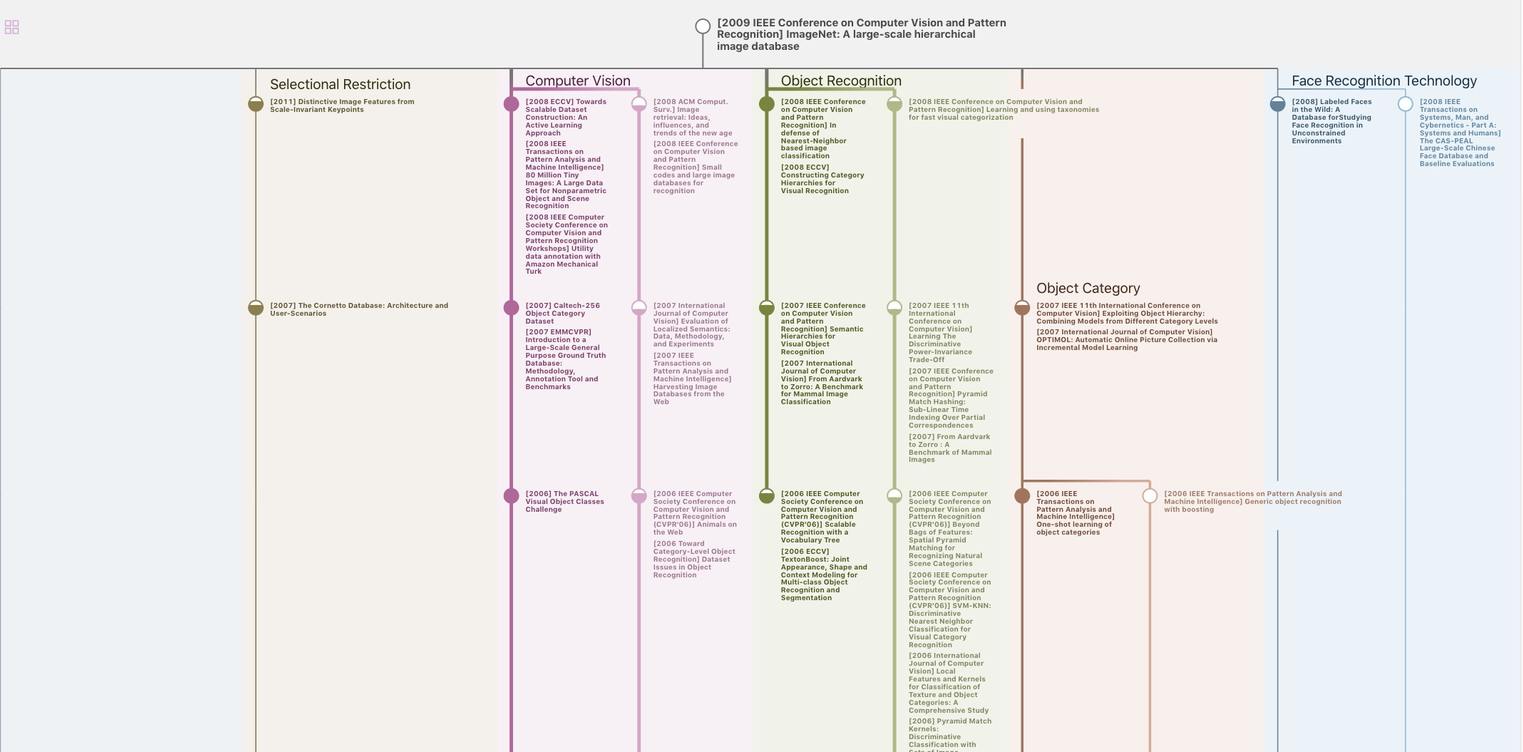
生成溯源树,研究论文发展脉络
Chat Paper
正在生成论文摘要