Permeability prediction of complex porous materials by conjugating generative adversarial and convolutional neural networks
COMPUTATIONAL MATERIALS SCIENCE(2024)
摘要
Empirical formulas, established by experimental statistical methods, have been a traditional method for predicting permeability in porous materials, but they suffer from shortcomings in terms of prediction accuracy. In this paper, a framework conjugating generative adversarial nets (GAN) and convolutional neural networks (CNN) is proposed for the rapid prediction of permeability based on 3D Berea sandstone images. This framework aims to surpass the limitations of empirical formulas in achieving accurate predictions. The framework involves three steps: porous material images reconstruction based on a conditional porosity progressive growth of generative adversarial nets (CPPGGAN), permeability label calculation based on the Lattice Boltzmann method (LBM) and deep learning prediction model construction using convolutional neural network (CNN). The CNN predictor trained on 3920 CPPGGAN synthetic images achieved an R2 value of 0.927 and 0.925 on 560 synthetic images and 60 original images, respectively. This indicated the effectiveness of the CPPGGAN synthetic images in the permeability prediction framework. The framework can also be adapted to a wider range of porous material types and shows great potential for exploring materials with exceptional properties in nature.
更多查看译文
关键词
Porous materials,Generative Adversarial Nets,Convolutional neural network,Permeability prediction
AI 理解论文
溯源树
样例
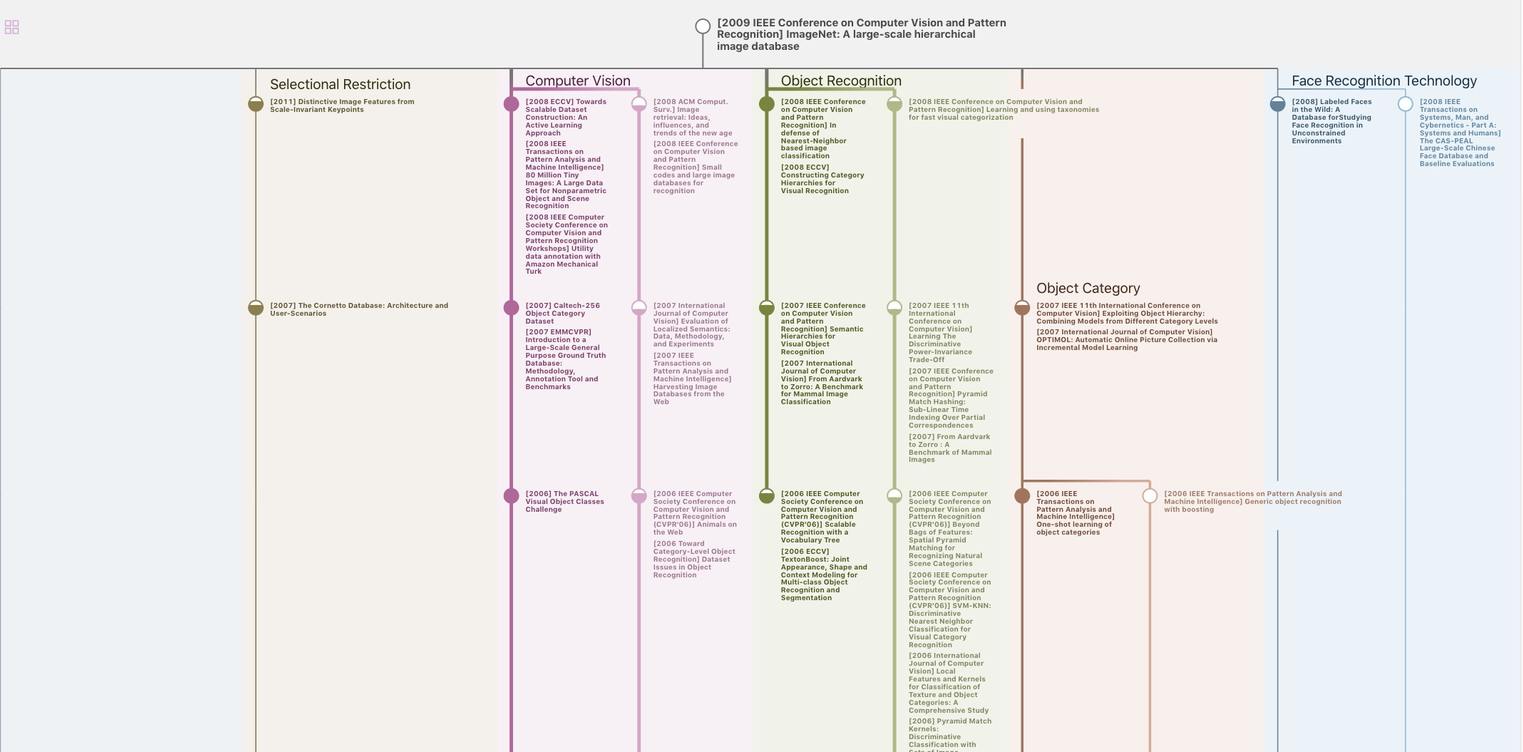
生成溯源树,研究论文发展脉络
Chat Paper
正在生成论文摘要