A new tool for energy conservation in operating room: the role of machine learning models in enhancing airflow control
Energy and Buildings(2024)
Abstract
Maintaining high ventilation rates in operating rooms (ORs) is critical to ensure the safety of healthcare professionals and patients. Yet, the significant energy consumption associated with ventilation systems is a growing global concern. While traditional approaches have predominantly focused on optimizing ventilation design, limited attention has been given to operational control strategies. This study aims to control ceiling air supply speed by exposure aerosol concentrations exhaled by the patient. It achieves this by integrating empirical data with machine learning (ML) models, effectively balancing the need for maintaining optimal ventilation conditions and ensuring a safe aerosol exposure concentration. Experimental results from a full-scale OR setup, manipulating ceiling air supply speed, respiratory flow rate, and surgical bed height, generated 81 datasets. These datasets served as the basis for assessing the performance of 11 ML models. Notably, the extra trees (ET) and multi-layer perceptron (MLP) models outperformed the others, exhibiting impressive coefficient of determination (R2) values of 0.954 and 0.904, respectively. Moreover, the models were further enhanced by assessing their robustness with Gaussian noise and interval prediction. This study presents a novel approach that holds significant relevance for the practical control of ventilation systems, particularly with regard to health and energy efficiency considerations.
MoreTranslated text
Key words
operating room,energy consumption,machine learning,ventilation,aerosol
AI Read Science
Must-Reading Tree
Example
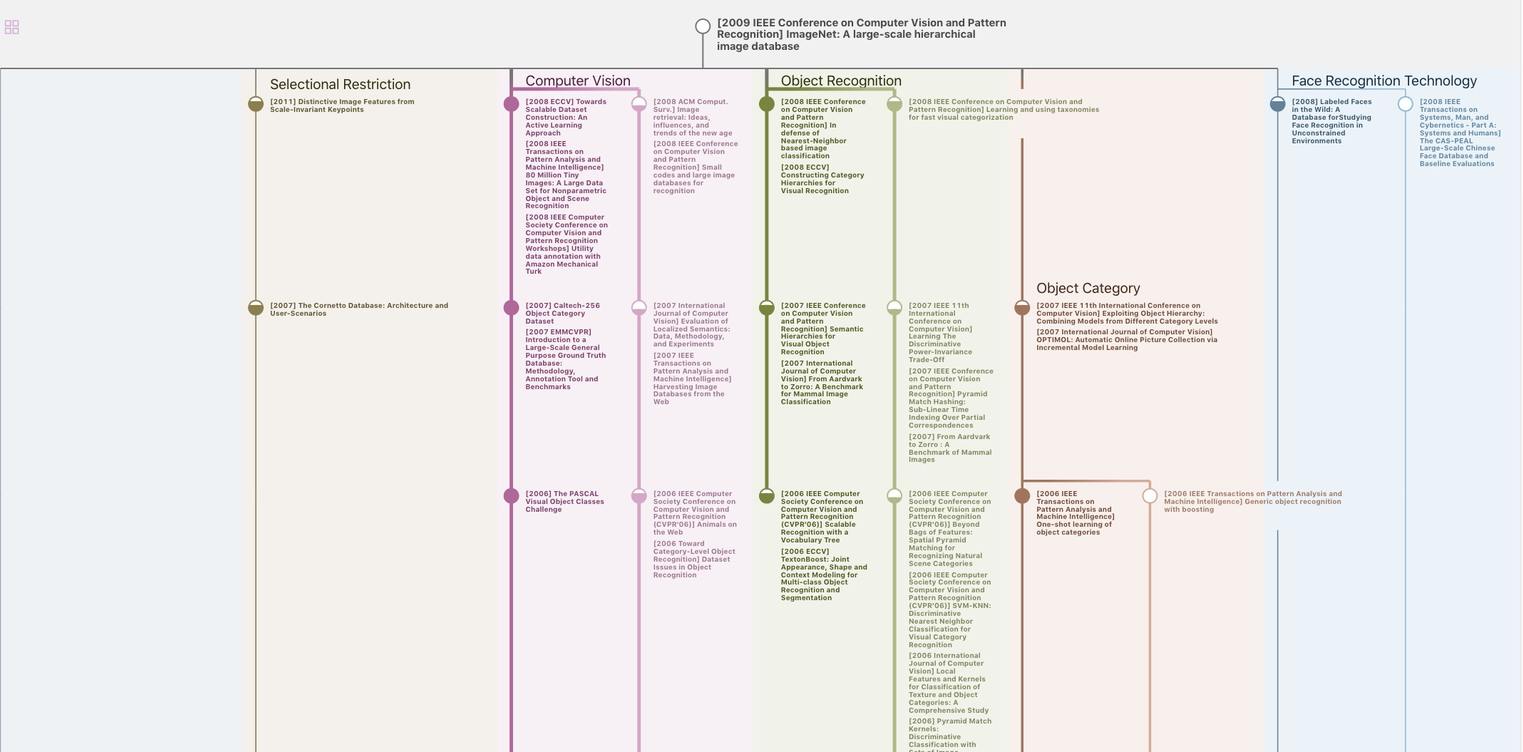
Generate MRT to find the research sequence of this paper
Chat Paper
Summary is being generated by the instructions you defined