An Improved Algorithm for Learning Drifting Discrete Distributions
International Conference on Artificial Intelligence and Statistics(2024)
摘要
We present a new adaptive algorithm for learning discrete distributions under
distribution drift. In this setting, we observe a sequence of independent
samples from a discrete distribution that is changing over time, and the goal
is to estimate the current distribution. Since we have access to only a single
sample for each time step, a good estimation requires a careful choice of the
number of past samples to use. To use more samples, we must resort to samples
further in the past, and we incur a drift error due to the bias introduced by
the change in distribution. On the other hand, if we use a small number of past
samples, we incur a large statistical error as the estimation has a high
variance. We present a novel adaptive algorithm that can solve this trade-off
without any prior knowledge of the drift. Unlike previous adaptive results, our
algorithm characterizes the statistical error using data-dependent bounds. This
technicality enables us to overcome the limitations of the previous work that
require a fixed finite support whose size is known in advance and that cannot
change over time. Additionally, we can obtain tighter bounds depending on the
complexity of the drifting distribution, and also consider distributions with
infinite support.
更多查看译文
AI 理解论文
溯源树
样例
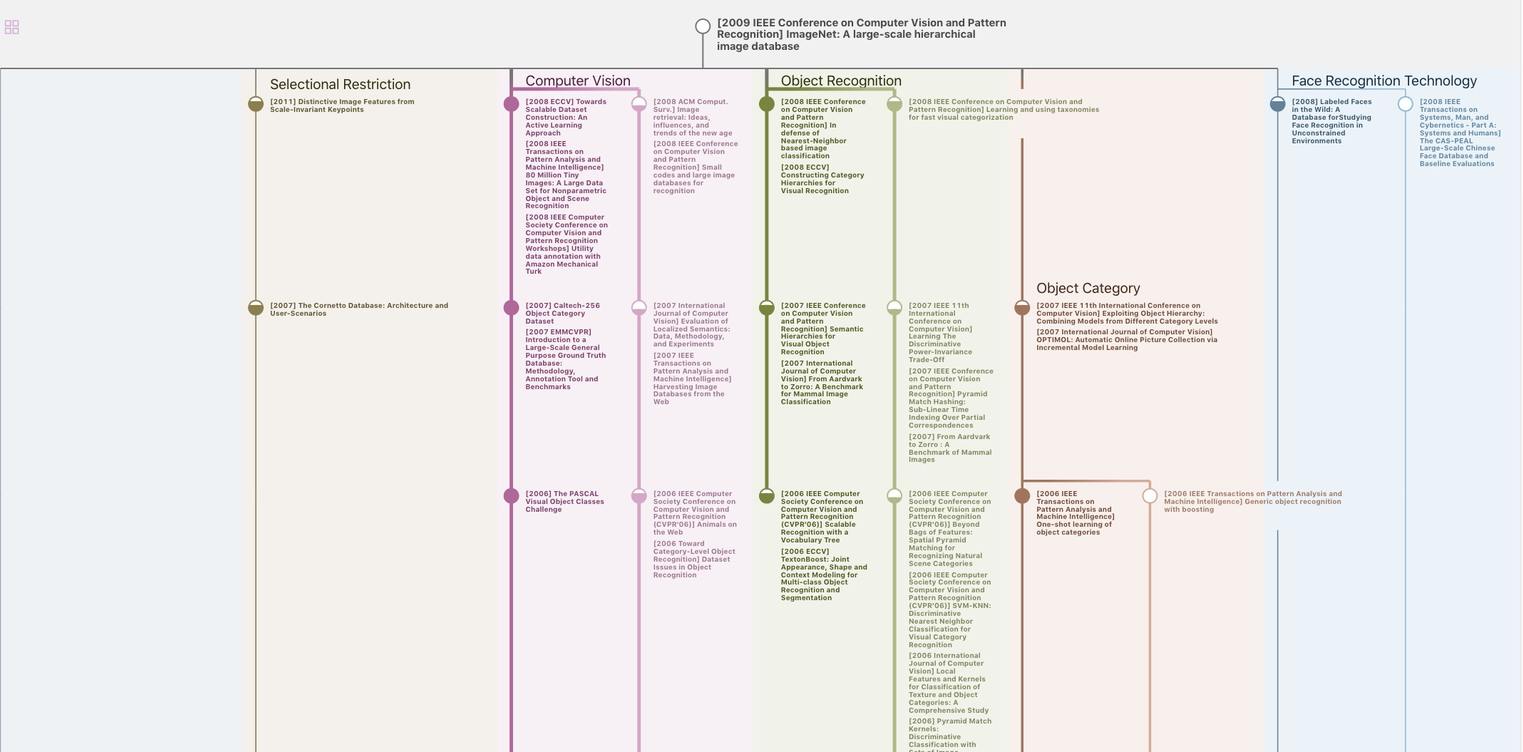
生成溯源树,研究论文发展脉络
Chat Paper
正在生成论文摘要