Advancing EOP Prediction: Bridging the Gaps
crossref(2024)
摘要
Understanding Earth's orientation parameters (EOP) is paramount for unraveling intricate mass redistribution, gravitational interactions, and geodynamic processes within the Earth's system. With a growing interest in EOP across diverse scientific disciplines such as Earth science, astronomy, and climate change studies, the demand for accurate and timely real-time information has become increasingly crucial. This is particularly evident in applications like satellite navigation, interplanetary spacecraft tracking, and weather forecasting. Despite the precision enabled by modern space geodetic techniques (e.g., Very Long Baseline Interferometry - VLBI, Global Navigation Satellite Systems - GNSS, and Satellite Laser Ranging - SLR), the complexity of data processing and associated delays necessitates significant progress in EOP prediction, especially for applications requiring timely information. This study addresses these challenges by introducing a redesigned prediction package that effectively bridges the gap between observational data and final estimated products. Utilizing a sophisticated combination of deterministic and stochastic methods, our prediction algorithm is applied to both the official International Earth Rotation and Reference Systems Service (IERS) EOP series and the Federal Agency for Cartography and Geodesy (BKG) single-specific and combined technique time series. The results of this study make a significant contribution to efforts that aim to enhance the accuracy of predicted Earth Orientation Parameters (EOP). By thoroughly exploring the selection of input data and prediction methods, our research strives to improve the dependability of EOP forecasts, especially for short-term predictions. By tackling crucial challenges in the field, this work not only deepens our understanding of Earth's dynamic processes but also opens doors for more accurate and timely applications across various scientific disciplines that rely on EOP data.
更多查看译文
AI 理解论文
溯源树
样例
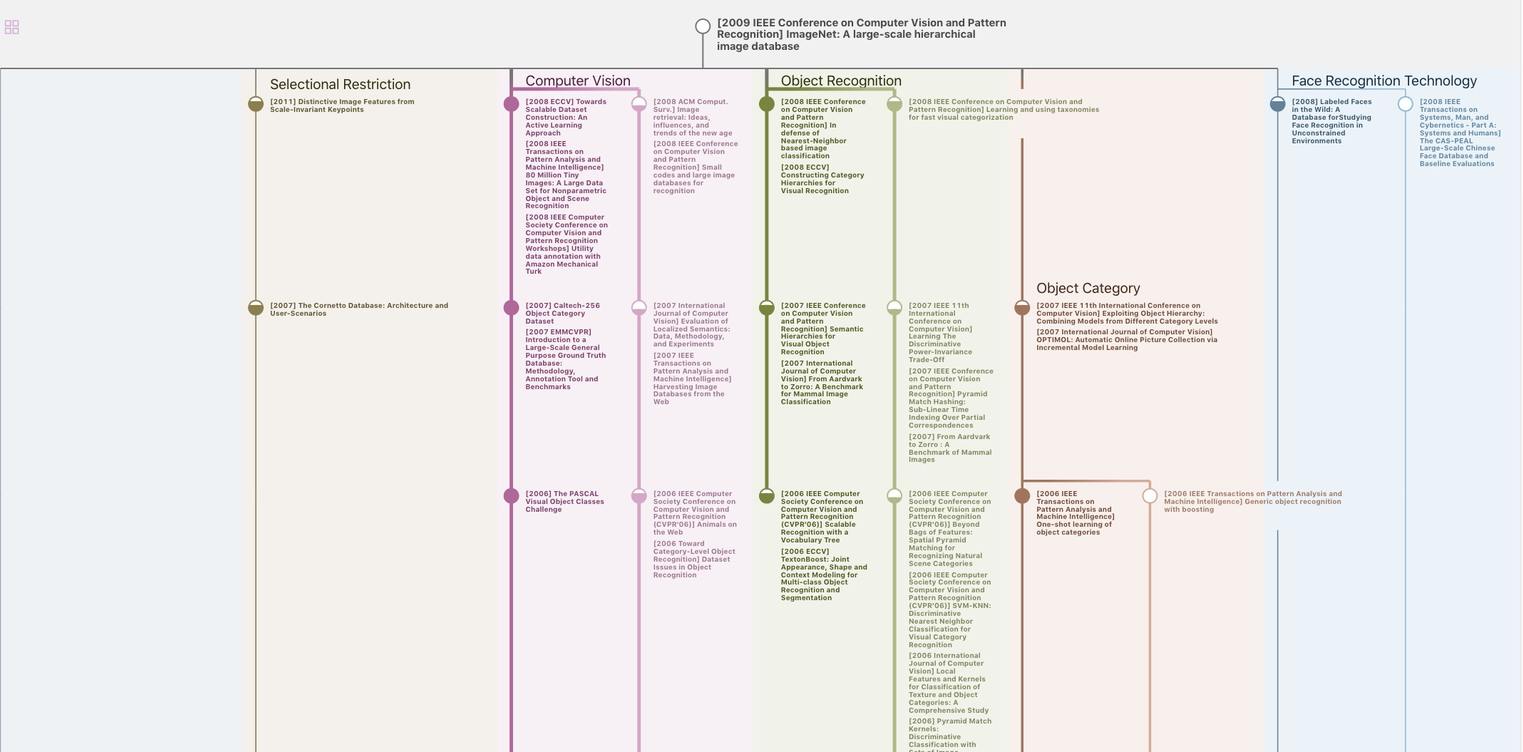
生成溯源树,研究论文发展脉络
Chat Paper
正在生成论文摘要