Optimizing telescoped heterogeneous catalysis with noise-resilient multi-objective Bayesian optimization
crossref(2024)
摘要
This study evaluates the noise resilience of multi-objective Bayesian optimization (MOBO) algorithms in chemical synthesis, an aspect critical for processes like telescoped reactions and heterogeneous catalysis but seldom systematically assessed. Through simulation experiments on amidation, acylation, and SNAr reactions under varying noise levels, we identify the qNEHVI acquisition function as notably proficient in handling noise. Subsequently, qNEHVI is employed to optimize a two-step heterogeneous catalysis for the continuous-flow synthesis of hexafluoroisopropanol. Achieving considerable optimization within just 20 experimental runs, we report an E-factor of 0.3744 and a conversion rate of 76.20%, with optimal conditions set at 5.00 sccm and 35.00℃ for the first step, and 80.00 sccm and 170℃ for the second. This research highlights qNEHVI’s potential in noisy multi-objective optimization and its practical utility in refining complex synthesis processes.
更多查看译文
AI 理解论文
溯源树
样例
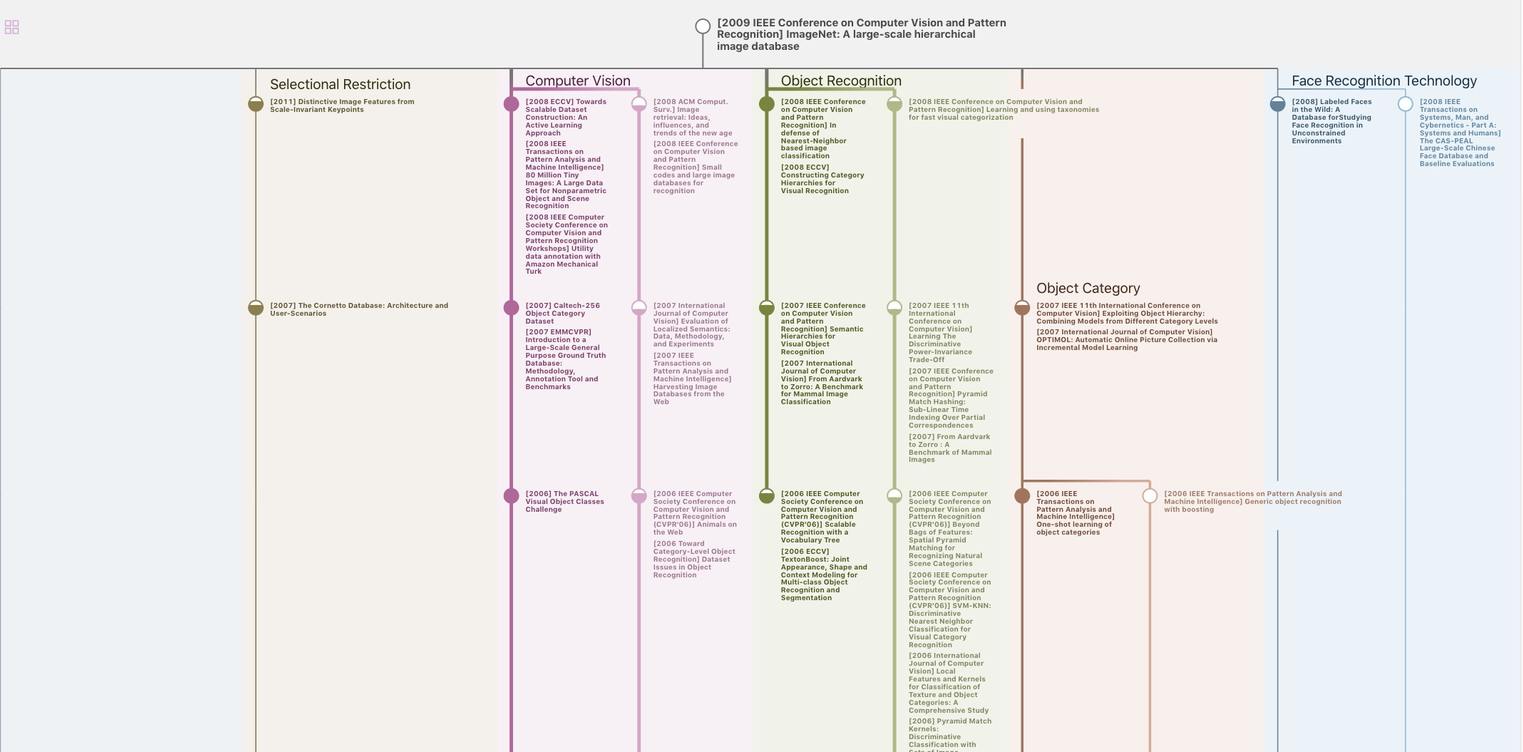
生成溯源树,研究论文发展脉络
Chat Paper
正在生成论文摘要