Sediment core DNA-Metabarcoding and chitinous remain identification: Integrating complementary methods to characterise Chironomidae biodiversity in lake sediment archives
crossref(2024)
摘要
Chironomidae, so-called non-biting midges, are considered key bioindicators of aquatic ecosystem variability. Data derived from morphologically identifying their chitinous remains in sediments documents chironomid larvae assemblages, which are studied to reconstruct ecosystem changes over time. Recent developments in sedimentary DNA (sedDNA) research have demonstrated that molecular techniques are suitable for determining past and present occurrences of organisms. Nevertheless, sedDNA records documenting alterations in chironomid assemblages remain largely unexplored. To close this gap, we examined the applicability of sedDNA metabarcoding to identify Chironomidae assemblages in lake sediments by sampling and processing three 21 – 35 cm long sediment cores from Lake Sempach in Switzerland. With a focus on developing analytical approaches, we compared an invertebrate-universal (FWH) and a newly designed Chironomidae-specific metabarcoding primer set (CH) to assess their performance in detecting Chironomidae DNA. We isolated and identified chitinous larval remains and compared the morphotype assemblages with the data derived from sedDNA metabarcoding. Results showed a good overall agreement of the morphotype assemblage-specific clustering among the chitinous remains and the metabarcoding datasets. Both methods indicated higher chironomid assemblage similarity between the two littoral cores in contrast to the deep lake core. Moreover, we experienced a pronounced primer bias effect resulting in more Chironomidae detections with the CH primer combination compared to the FWH combination. Overall, we conclude that sedDNA metabarcoding can supplement traditional remain identifications and potentially provide independent reconstructions of past chironomid assemblage changes. Furthermore, it has the potential of more efficient workflows, better sample standardisation and species-level resolution datasets.
更多查看译文
AI 理解论文
溯源树
样例
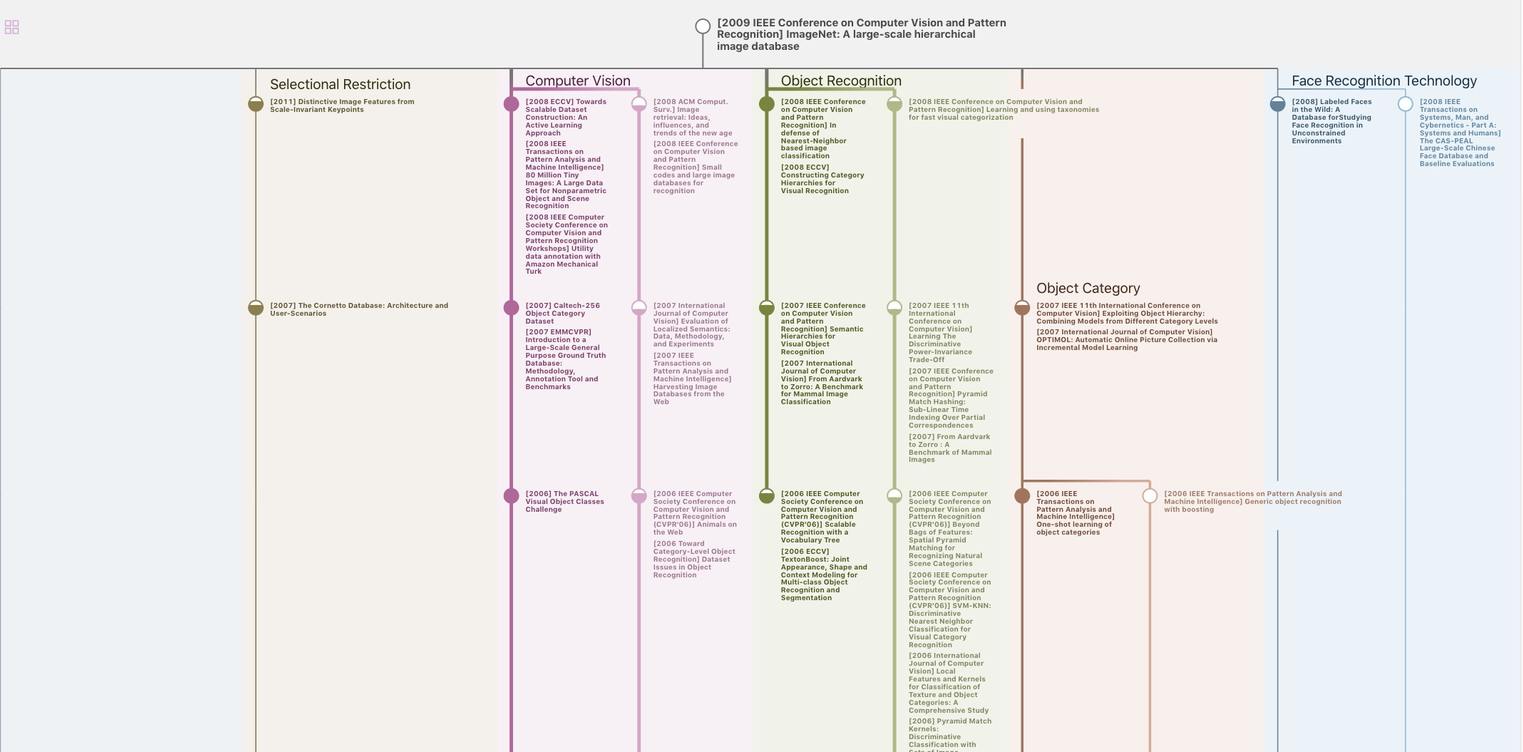
生成溯源树,研究论文发展脉络
Chat Paper
正在生成论文摘要