A deep learning approach based on Richardson and Gauss-Seidel for massive MIMO detection
TRANSACTIONS ON EMERGING TELECOMMUNICATIONS TECHNOLOGIES(2024)
摘要
Massive multiple-input multiple-output (MIMO) systems can improve the spectrum utilization and the system capacity, but this also increases the computational complexity of the signal detection. The existing iterative algorithms can greatly reduce the computational complexity; however, the detection performance is limited. In order to achieve a better balance between the computational complexity and the detection performance, this article combines the model-driven deep learning approached with Massive MIMO signal detection to construct RGNet (RIGS-based deep learning Network). First, RIGS is proposed as a hybrid method of RI (Richardson) and GS (Gauss-Seidel). The RIGS algorithm combines these methods to achieve faster convergence. However, the performance of RIGS joint algorithm is limited to the spatially correlated channel scenarios. To improve robustness, we further extend RIGS, by adding learnable parameters in each iteration and introducing staircase activation functions to significantly improve detection performance. Simulation results show that the proposed RGNet has low computational complexity and a simple and fast training process. It can also achieve excellent detection performance in Rayleigh fading channel and spatially correlated channel.
更多查看译文
AI 理解论文
溯源树
样例
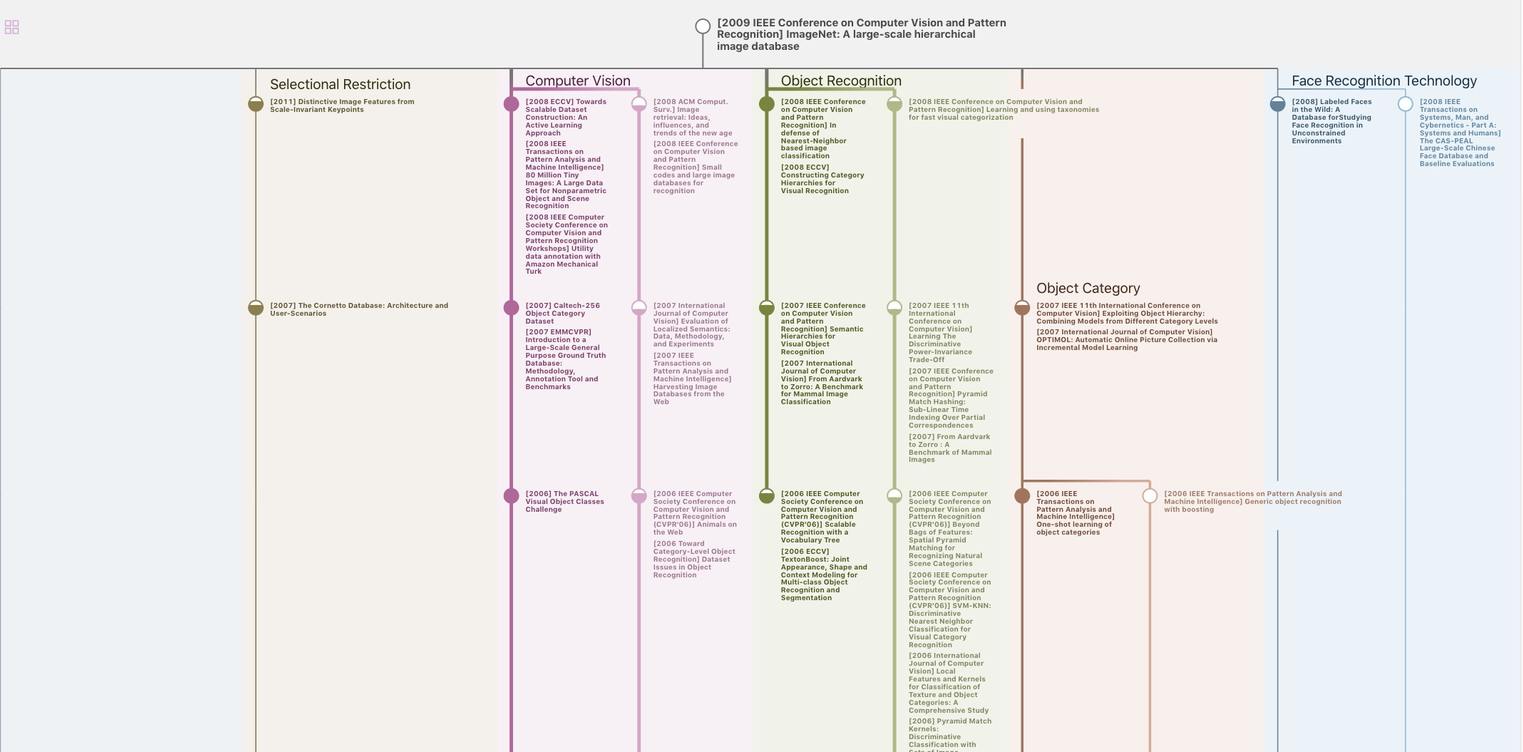
生成溯源树,研究论文发展脉络
Chat Paper
正在生成论文摘要