Machine-Learning-Based Ground Motion Models for Crustal and Subduction Zones of West Java, Indonesia
crossref(2024)
摘要
This study presents Ground-motion Models developed to predict Peak Ground Acceleration (PGA), Peak Ground Velocity (PGV), and 5% damped pseudo-spectral acceleration (PSA) at periods of 0.3, 1, and 3 seconds in West Java, Indonesia. The models consider Moment Magnitude, Epicentral Distance, Depth, and Soil site condition. We develop comprehensive ground motion models using three machine-learning techniques—Artificial Neural Network (ANN), Random Forest (RF), and Extreme Gradient Boosting (XGB) for the study area. The dataset comprises 2016 ground motions recorded at 60 stations during 211 earthquakes, including the notable M 5.6 Cianjur events. To assess model performance, we utilize evaluation metrics such as coefficient of determination (R2), root mean square error (RMSE), mean absolute error (MAE), standard deviation of residuals, and between-event and within-event residuals. Comparative analysis reveals the superiority of the three machine learning models over the classical regression approach, with Random Forest exhibiting the most robust performance. The best model is effectively applied to recent earthquakes, providing accurate predictions at various stations. These models will be valuable for updating seismic hazard analyses and contributing as inputs in developing an earthquake early warning system in this region.
更多查看译文
AI 理解论文
溯源树
样例
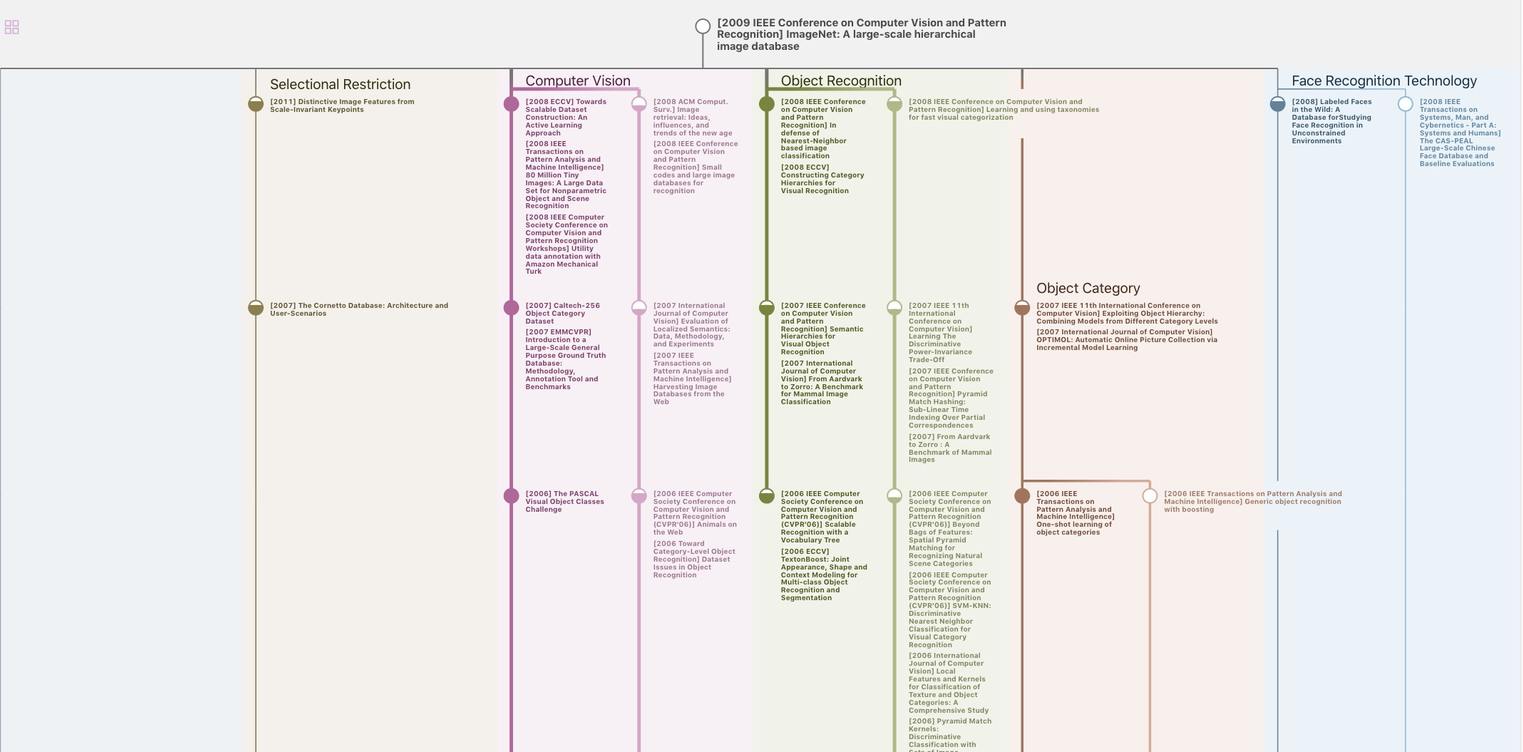
生成溯源树,研究论文发展脉络
Chat Paper
正在生成论文摘要