Artificial intelligence driven malnutrition diagnostic model for patients with acute abdomen based on GLIM criteria: a cross-sectional research protocol.
BMJ open(2024)
摘要
BACKGROUND:Patients with acute abdomen often experience reduced voluntary intake and a hypermetabolic process, leading to a high occurrence of malnutrition. The Global Leadership Initiative on Malnutrition (GLIM) criteria have rapidly developed into a principal methodological tool for nutritional diagnosis. Additionally, machine learning is emerging to establish artificial intelligent-enabled diagnostic models, but the accuracy and robustness need to be verified. We aimed to establish an intelligence-enabled malnutrition diagnosis model based on GLIM for patients with acute abdomen.
METHOD:This study is a single-centre, cross-sectional observational investigation into the prevalence of malnutrition in patients with acute abdomen using the GLIM criteria. Data collection occurs on the day of admission, at 3 and 7 days post-admission, including biochemical analysis, body composition indicators, disease severity scoring, nutritional risk screening, malnutrition diagnosis and nutritional support information. The occurrence rate of malnutrition in patients with acute abdomen is analysed with the GLIM criteria based on the Nutritional Risk Screening 2002 and the Mini Nutritional Assessment Short-Form to investigate the sensitivity and accuracy of the GLIM criteria. After data cleansing and preprocessing, a machine learning approach is employed to establish a predictive model for malnutrition diagnosis in patients with acute abdomen based on the GLIM criteria.
ETHICS AND DISSEMINATION:This study has obtained ethical approval from the Ethics Committee of the Sichuan Academy of Medical Sciences and Sichuan Provincial People's Hospital on 28 November 2022 (Yan-2022-442). The results of this study will be disseminated in peer-reviewed journals, at scientific conferences and directly to study participants.
TRIAL REGISTRATION NUMBER:ChiCTR2200067044.
更多查看译文
AI 理解论文
溯源树
样例
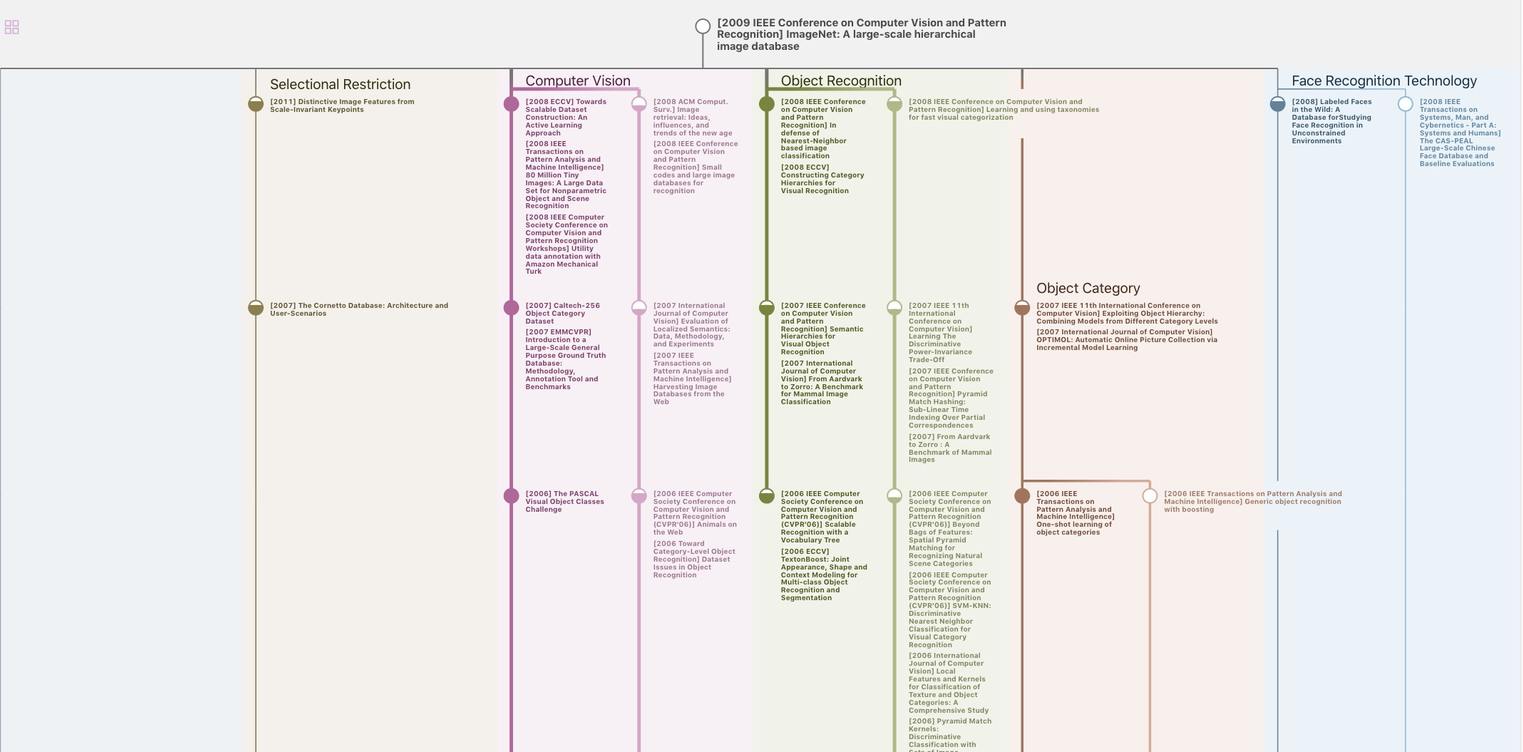
生成溯源树,研究论文发展脉络
Chat Paper
正在生成论文摘要