Estimation of Lateral River Aquifer Exchanges with Physics Informed Neural Networks
crossref(2024)
Abstract
This study introduces a novel approach for estimating lateral river-aquifer exchanges by employing Physics Informed Neural Networks (PINNs). The methodology compares the predictive capabilities of neural networks with the physics-based modeling provided by MODFLOW's Horizontal Flow Barrier (HBF) package, implemented through FloPy. As a foundation, the HBF package in MODFLOW establishes a baseline model, serving as a benchmark for performance comparison. The integrated model leverages observed data and the fundamental principles of hydrogeology, enabling a robust estimation of lateral exchanges. The synergy of PINNs and MODFLOW HBF enhances the model's adaptability to diverse hydrogeological conditions, providing accurate predictions of intricate river-aquifer interactions. The comparative analysis with the MODFLOW HBF package underscores the efficacy of the proposed approach, offering insights for improved water resource management and environmental decision-making.
MoreTranslated text
AI Read Science
Must-Reading Tree
Example
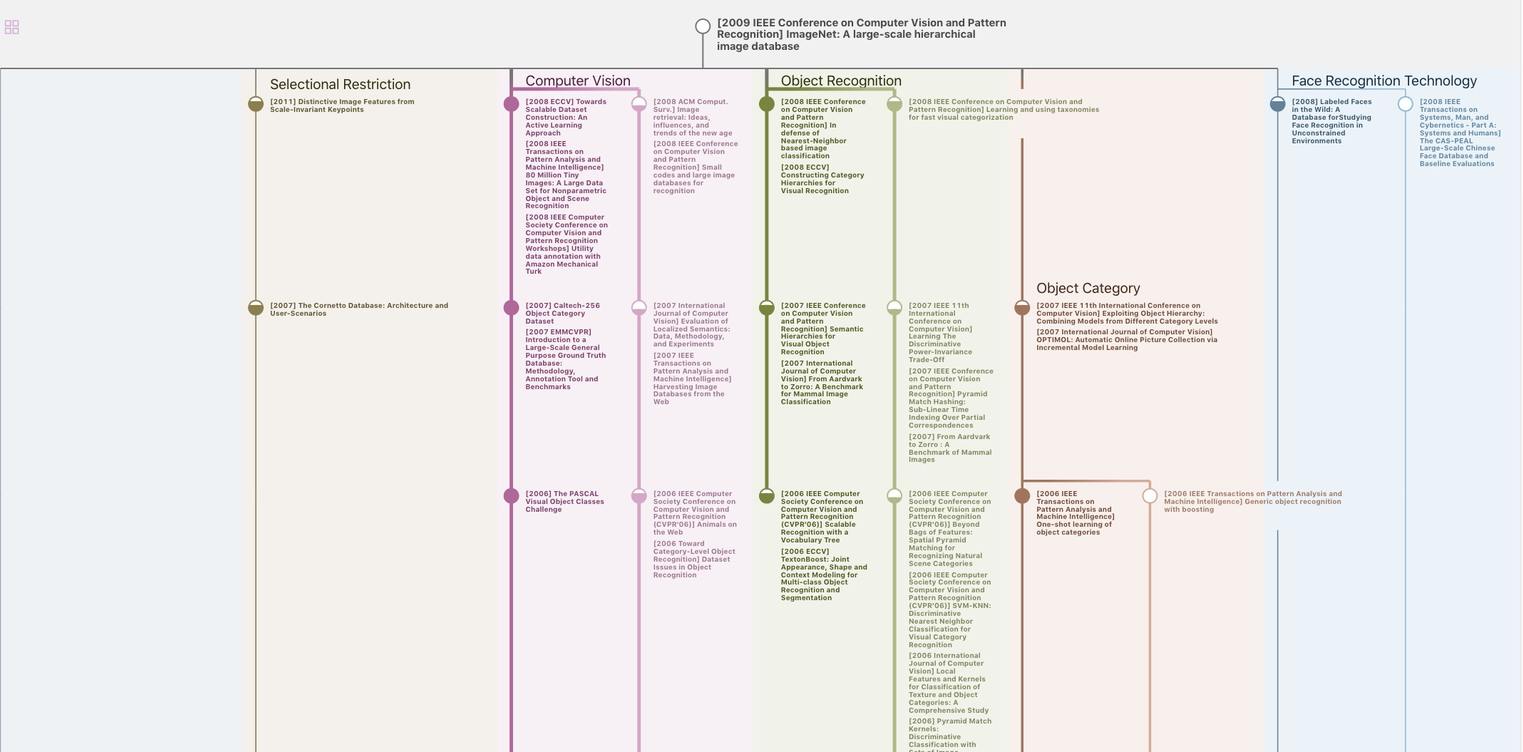
Generate MRT to find the research sequence of this paper
Chat Paper
Summary is being generated by the instructions you defined