Crop model calibration at multiple spatial levels in southern Sweden using leaf area index, evapotranspiration and winter wheat yield data
crossref(2024)
摘要
Matching the rising demand for food from a rapidly increasing population is a crucial challenge for the 21st century. Crop yield can be strongly impacted by weather conditions, especially extreme events (e.g., floods and droughts). Therefore, understanding the spatial and temporal vaiations of crop yield enables us to develop effective adaptation strategies for enhancing the resilience of agriculture sectors under climate change. Dynamic global vegetation models (DGVMs) represent growth and development of vegetation based on the understanding of underlying physical and physiological processes, which are efficient tools to assess impacts of climate change and human management on vegetation response to these variations. During the past two decades, satellite observations have emerged as essential gridded datasets to calibrate various vegetation-related variables at large spatial scales, and are often used to benchmark DGVMs. Commonly used satellite-derived variables for model calibration include the fraction of absorbed photosynthetically active radiation, phenological dates, soil properties, leaf area index (LAI), and evapotranspiration (ET). One of the process-based DGVMs LPJ-GUESS (Lund-Potsdam-Jena General Ecosystem Simulator) has shown its acceptable performance in simulating crop yield at global and regional scales. However, to the best of our knowledge, no studies have comprehensively investigated the added value of using multi-source data, particularly satellite-derived estimates for calibrating LPJ-GUESS at multiple spatial scales. Therefore, we aim to bridge this gap by calibrating LPJ-GUESS at both site and district level to improve model performance of simulating the winter wheat yield in southern Sweden. First, all the parameters sensitive to LAI, ET and crop yield in LPJ-GUESS, along with their ranges, are derived from the literature and sensitivity analysis. Second, the LAI-related parameters are calibrated against the in-situ observed LAI at the experimental site in Alnarp during 2022–2023. The simulated yield based on the optimized parameters (achieved from calibration results) is further validated by the observed winter wheat yield at the same site. At the district level, the parameters for ET and crop yield are subsequently calibrated against the satellite-derived ET and crop yield estimates, and the observed district-level winter wheat yield (from Jordbruksverket), respectively during 2003–2012. Finally, the observed district-level yield during 2013–2022 are used for the model validation to access the performance of the calibrated LPJ-GUESS. We expect this proposed calibration framework can be applied to other DGVMs and geographic extents focusing on high details of local landscape.
更多查看译文
AI 理解论文
溯源树
样例
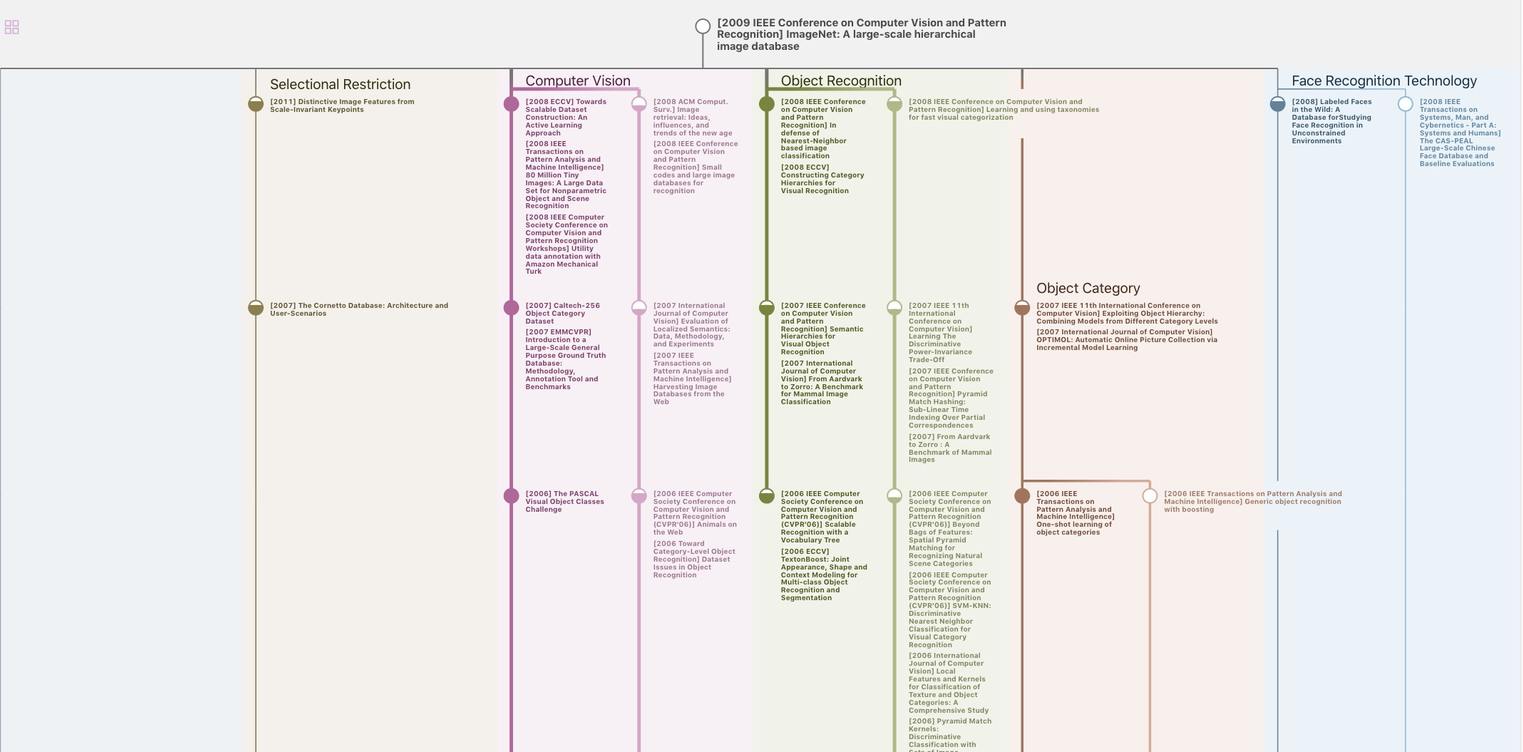
生成溯源树,研究论文发展脉络
Chat Paper
正在生成论文摘要