Explaining GNSS station movements based on Earth observation data
crossref(2024)
摘要
Global Navigation Satellite Systems (GNSS) are best known for their accurate positioning, navigation and timing capabilities. In total, over 20.000 permanent high-grade GNSS stations are available worldwide, the positions of which are monitored with millimeter accuracy. Thanks to the high accuracy and the fact that these stations are mounted on the ground, subtle movements due to hydrological changes and crustal deformation can be observed. Thus, the GNSS observations contain valuable geophysical information. Although many geodetic applications require these movements to be properly understood and potentially corrected, this is not trivial due to the complexity of the interactions within the Earth’s system. Therefore, there is a severe lack of available models explaining residual GNSS station movements beyond conventionally modeled effects. On the opposite, if these movements are properly understood, GNSS observations might contribute to the correct interpretation of emerging environmental changes. This study exploits the wealth of satellite-derived Earth observation (EO) data to derive suitable models to explain GNSS station movements. We combine GNSS station coordinate time series and EO variables with the help of machine learning techniques to benefit from various types of information. While the target vector consists of concatenated GNSS station coordinate time series over Europe, EO variables such as precipitation, soil water, snow water equivalent, and land cover data are used as input features. Different machine learning models, including Random Forest, XGBoost, and Multilayer Perceptron, are investigated and compared. Additionally, a sensitivity analysis is performed to determine the individual impact of EO variables to quantify what drives GNSS movements, which in turn, might allow monitoring the corresponding Earth system processes based on GNSS observations.
更多查看译文
AI 理解论文
溯源树
样例
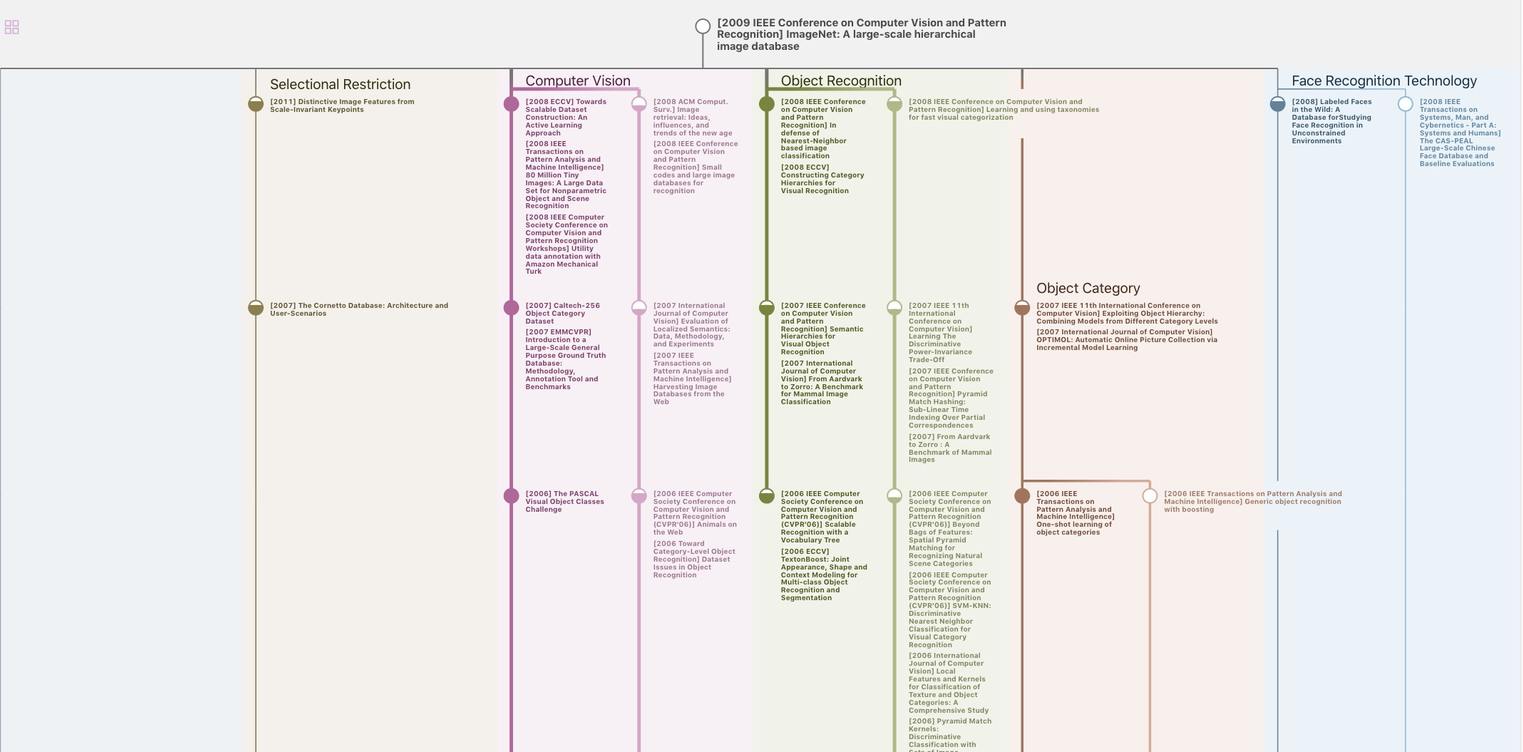
生成溯源树,研究论文发展脉络
Chat Paper
正在生成论文摘要