Reconciling risk-based and storyline attribution with Bayes theorem
crossref(2024)
摘要
Current research on climate change attribution falls into two broad camps. Classic “risk-based” studies typically assess differences in the distribution of some climate variable between two scenarios: one representing factual conditions and one without man-made climate change. More recently, this line of investigation has been complemented by “storyline” approaches, which consider the impact of climate change, conditional on a particular state of the internal climate variability.The apparent gap between the two approaches can be bridged with Bayesian statistics. We demonstrate that a conditional attribution statement depends on two unconditional Bayesian decisions between the scenarios, one using all information and one using everything except the event of interest.To illustrate this result, we employ Gaussian mixture models to conduct conditional and unconditional attribution studies of European summer temperatures based on multiple CMIP6 ensemble simulations. We find that the resulting attribution statements can be either strengthened or weakened by the conditioning, depending on the estimated covariance structure.
更多查看译文
AI 理解论文
溯源树
样例
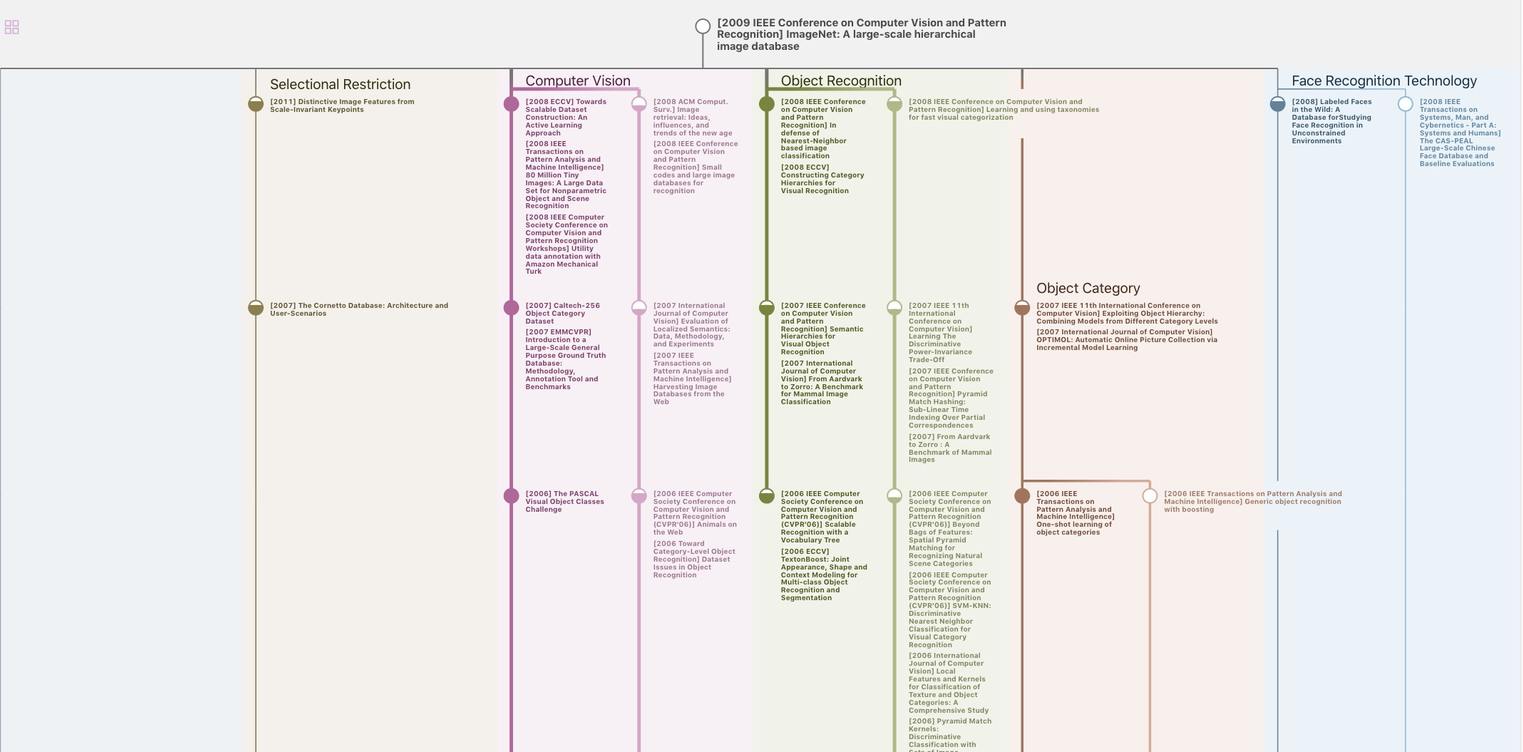
生成溯源树,研究论文发展脉络
Chat Paper
正在生成论文摘要