Pretraining Foundation Models: Unleashing the Power of Forgotten Spectra for Advanced Geological Applications
crossref(2024)
摘要
X-ray fluorescence (XRF) core scanning, renowned for its high-resolution, non-destructive, and user-friendly operation, is pivotal in geological research for analyzing chemical, physical, and biological signals. Despite the extensive applications of XRF data for various research purposes, the quantification of this data into specific geological proxies remains challenging due to the inherent non-linearity caused by simple sample pretreatment during core scanning. Leveraging advancements in deep learning, computing power and large-scale scientific drilling programs, our study aims to address this non-linearity by harnessing the often-overlooked raw XRF spectra stored in laboratory databases. We introduce an approach involving self-supervised pretraining on 54,643 spectra from marine sediments in the high-latitude sectors of the Pacific Ocean (cruises SO178, SO264, PS97, PS75, LV29). Our model, underpinned by a deep bidirectional image transformer (ViT-base), is trained to reconstruct heavily masked spectra (75%) with an R2 accuracy of 0.996, demonstrating its proficiency in feature extraction from limited data portions. This foundational model is anticipated to serve as a versatile tool for various downstream geological applications after finetuning with specific labeled data, such as quantifying high-resolution calcium carbonate (CaCO3) and detecting machinery anomalies. Future work includes expanding the spectrum database with diverse materials and machine settings to enhance the model's generalizability, ultimately broadening its applicability beyond core scanning for geological applications to encompass all XRF measurement techniques.
更多查看译文
AI 理解论文
溯源树
样例
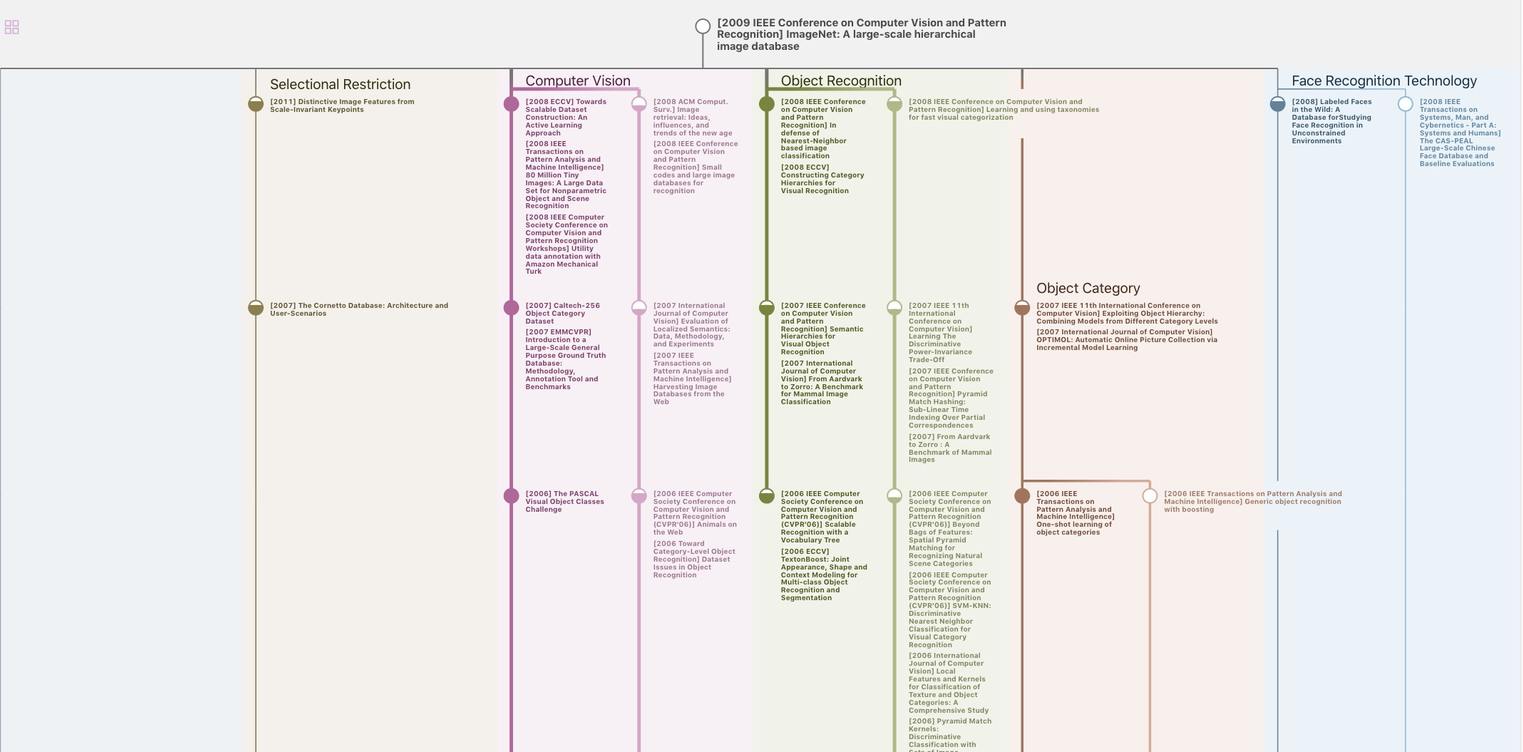
生成溯源树,研究论文发展脉络
Chat Paper
正在生成论文摘要