A Hierarchical Transfer-Generative Framework for Automating Multianalytical Tasks in Rail Surface Defect Inspection
IEEE Internet of Things Journal(2024)
摘要
Rail surface inspection is crucial for ensuring the safety and longevity of rail transport systems, grapples with the challenges posed by the scarcity of defective samples. Additionally, contemporary techniques in this domain typically fail to concurrently identify and localize defects at both image level and pixel levels. Addressing these intricacies, we present a hierarchical transfer-generative framework, the HTg-Net. This innovative framework is geared toward the automation and enhancement of multianalytical tasks in rail surface inspection. The HTg-Net architecture synergistically melds two pivotal subnetworks: 1) the memory-guided generation subnetwork (MGN), which is endowed with a cutting-edge memory mechanism. This mechanism adeptly captures and recalls the typical patterns observed in rail images, facilitating the detection of anomalies or deviations and 2) the attention-focused segmentation subnetwork (ASN) is fortified with a gated attention mechanism and hierarchical weights transferred from MGN, enabling parallel feature extraction and enhancing defect localization. Rigorous evaluations of HTg-Net on three data sets elucidate its superior efficiency and performance over prevailing benchmarks, positioning it as an advanced solution for the comprehensive inspection of rail surface defects.
更多查看译文
关键词
Deep learning,defect inspection,machine-vision analytics,rail transport safety,system health management
AI 理解论文
溯源树
样例
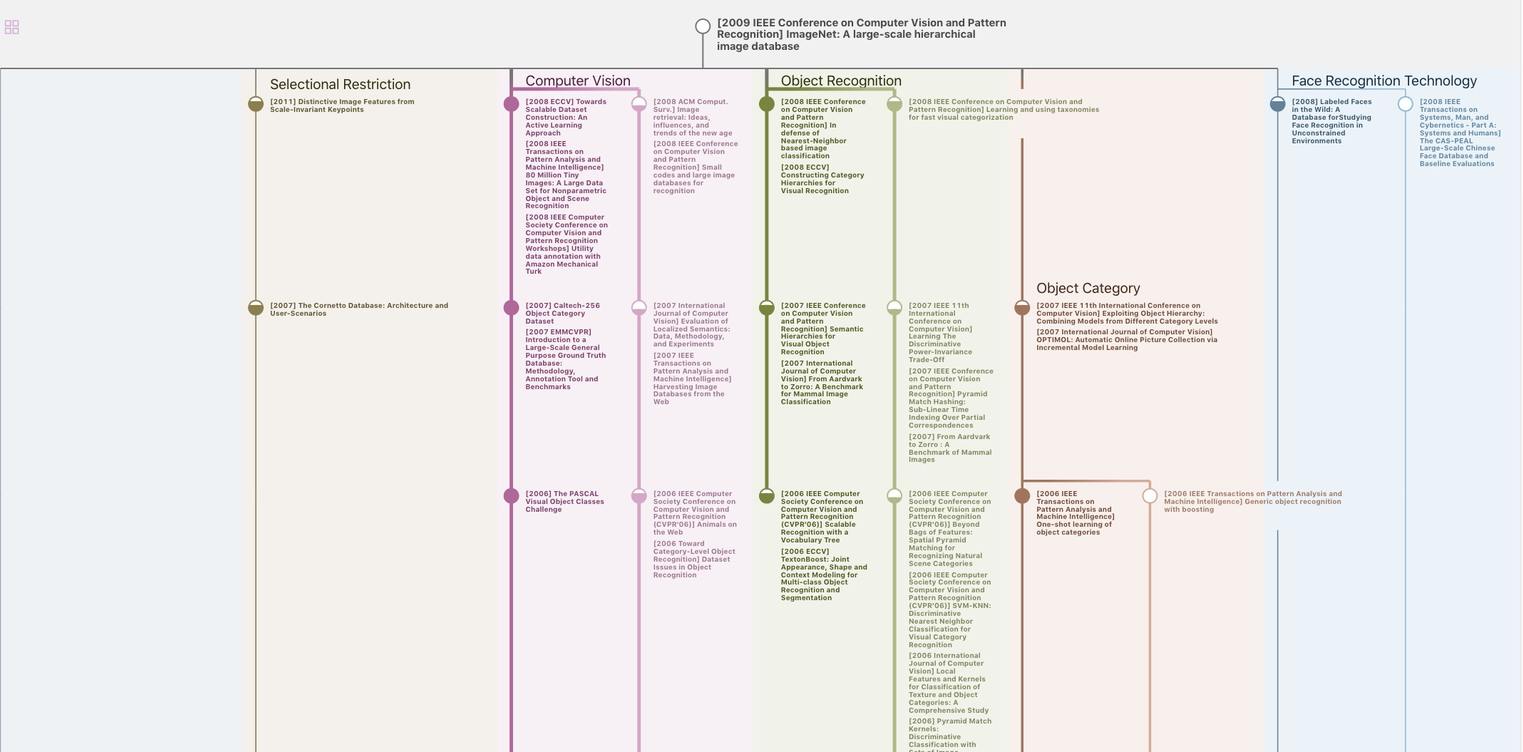
生成溯源树,研究论文发展脉络
Chat Paper
正在生成论文摘要