Fortune favors the invariant: Enhancing GNNs' generalizability with Invariant Graph Learning
KNOWLEDGE-BASED SYSTEMS(2024)
Abstract
Generalizable and transferrable graph representation learning endows graph neural networks (GNN) withthe ability to extrapolate potential test distributions. Nonetheless, current endeavors recklessly ascribe thedemoralizing performance on a single entity (feature or edge) distribution shift and resort to uncontrollableaugmentation. By inheriting the philosophy of Invariant graph learning (IGL), which characterizes a fullgraph as an invariant core subgraph (rationale) and a complementary trivial part (environment), we proposea universal operator termed InMvie to release GNN's out-of-distribution generation potential. The advantagesof our proposal can be attributed to two main factors: the comprehensive and customized insight on eachlocal subgraph, and the systematical encapsulation of environmental interventions. Concretely, a rationaleminer is designed to find a small subset of the input graph - rationale, which injects the model with featureinvariance while filtering out the spurious patterns, i.e., environment. Then, we utilize systematic environmentintervention to ensure the out-of-distribution awareness of the model. Our InMvie has been validated throughexperiments on both synthetic and real-world datasets, proving its superiority in terms of interpretability andgeneralization ability for node classification over the leading baselines
MoreTranslated text
Key words
Graph neural networks,Invariant Graph Learning,Out-of-distribution generalization
AI Read Science
Must-Reading Tree
Example
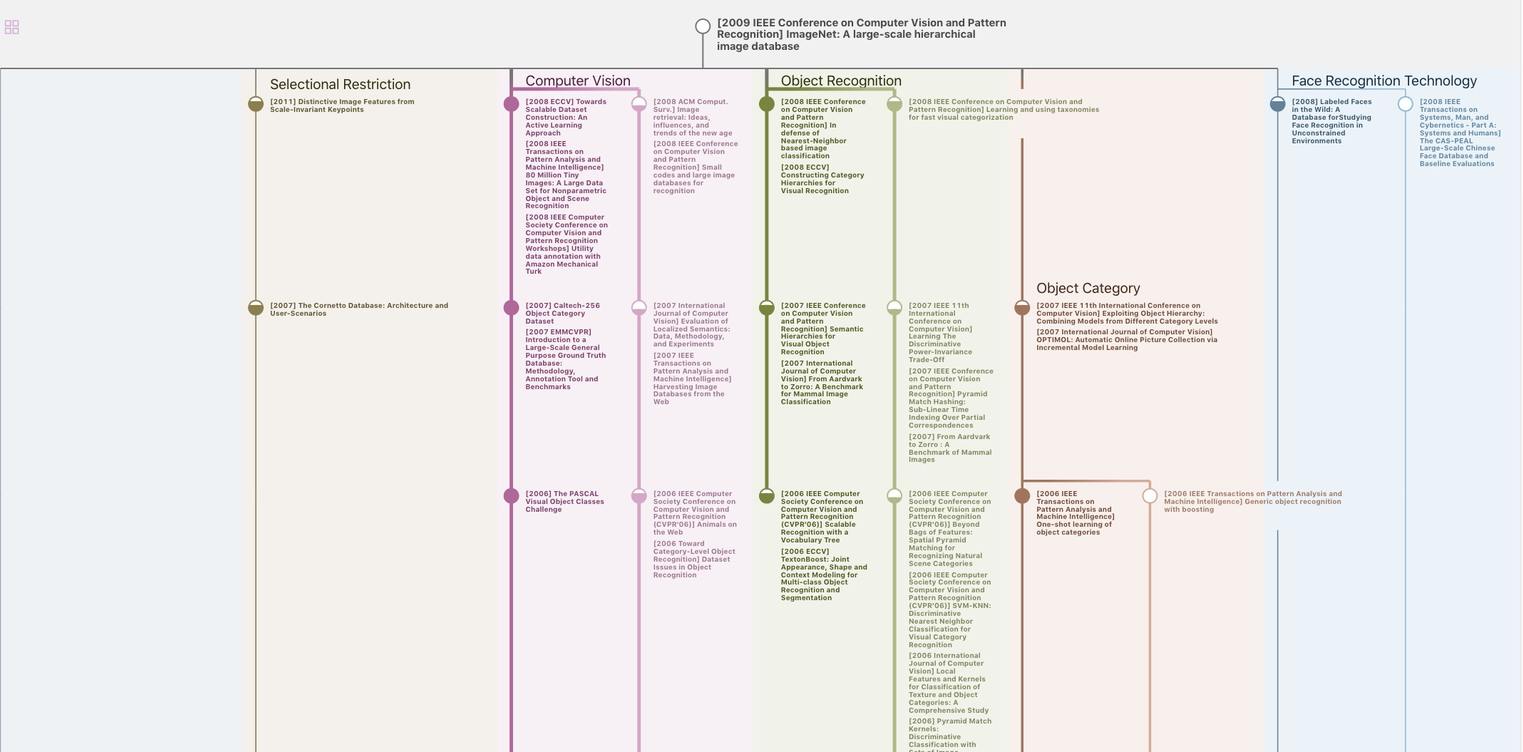
Generate MRT to find the research sequence of this paper
Chat Paper
Summary is being generated by the instructions you defined