A differentiable, physics-informed ecosystem modeling: investigating the photosynthetic capacity parameterization 
crossref(2024)
摘要
Different parameters serve as key determinants in simulating the photosynthesis process and representing photosynthetic traits as the net photosynthetic rates and the stomatal conductance. Previous methods of calibrating these photosynthetic parameters at individual sites encountered challenges in harnessing large-scale datasets and encountered issues such as overfitting or non-uniqueness of parameters. Moreover, they often relied solely on plant functional types (PFTs) to capture the inherent variability in these parameters or used other empirical values. These ignored the impact of acclimation of these parameters to the prevalent environmental conditions. Here, we utilized a recently developed differentiable ecosystem model—a hybrid, physics-informed machine learning system that can digest large global dataset and output interpretable intermediate variables —to do parameter inversion using PFT along with various environmental factors, including air temperature, radiation, and vapor pressure deficit, both over short-term and long-term durations. We mainly focused on the photosynthetic capacity related parameters represented in the maximum carboxylation rate standardized to 25 ॰C (Vc,max25), the stomatal conductance parameters, and some temperature response function parameters. To achieve this, we employed different neural network (NN) configurations to explore the dependence of these sets of parameters on environmental conditions while employing a loss function that uses the observations of both net photosynthetic rates (An) and stomatal conductance (gs). Using this framework we were able to have a better understanding of the correlation between physical parameters and the surrounding environment for different PFTs.
更多查看译文
AI 理解论文
溯源树
样例
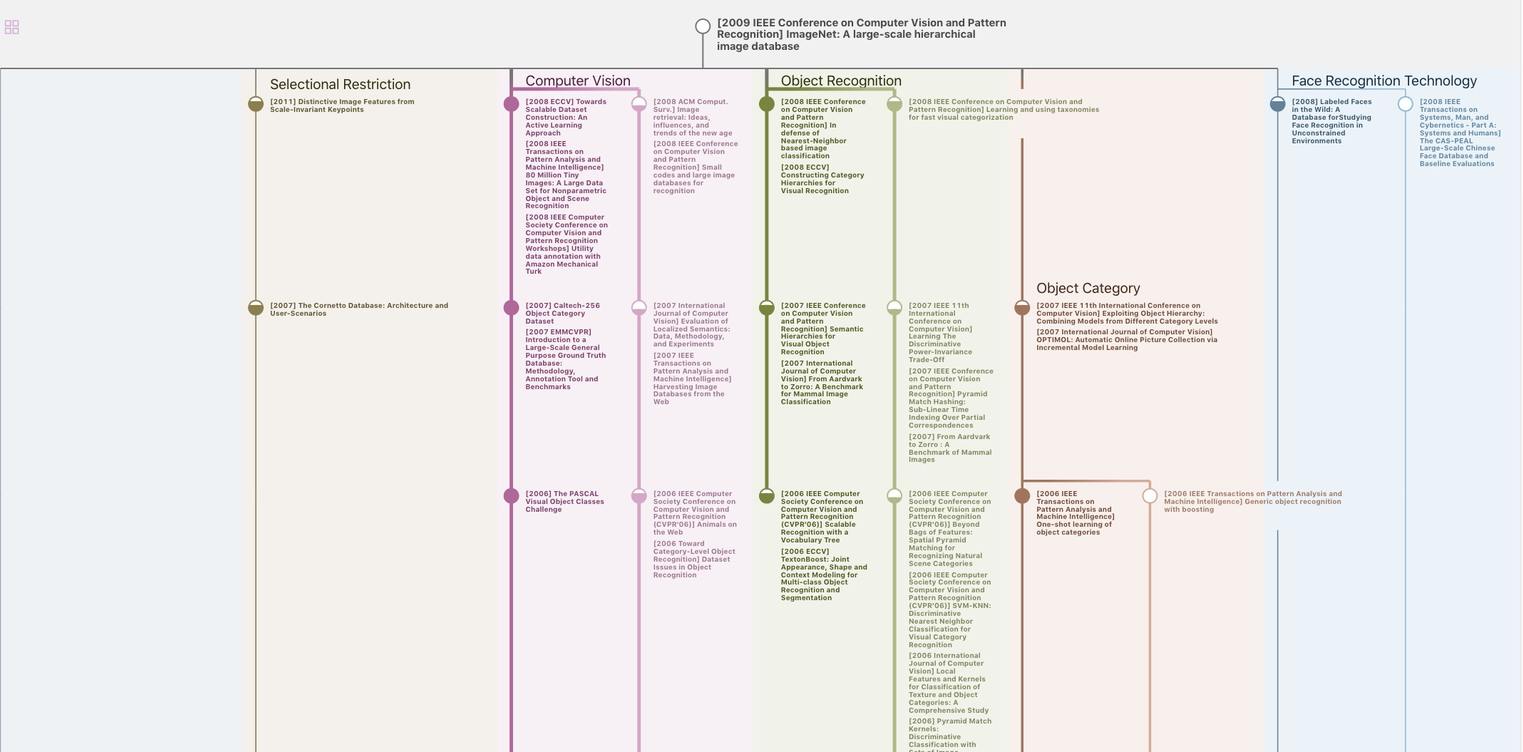
生成溯源树,研究论文发展脉络
Chat Paper
正在生成论文摘要