Comparative Analysis of Text Mining and Clustering Techniques for Assessing Functional Dependency between Manual Test Cases
crossref(2024)
摘要
Abstract Text mining techniques have garnered increasing interest for qualitative data analysis in software testing, especially with the advent of machine learning for natural language processing. However, the intricate nature of text mining techniques can pose challenges to their applicability, particularly in domains characterized by safety-critical systems. In such contexts, simpler and more transparent solutions may be preferable unless they significantly compromise accuracy. This study explores the trade-off between complexity, effort, accuracy, and utility of text mining and clustering technologies. The study evaluates the performance of different techniques in the context of detecting functional dependencies between manual integration test cases within safety-critical systems. Empirical data from an industrial testing project conducted at ALSTOM Sweden is used to assess the effectiveness of these techniques. The findings shed light on the comparative effectiveness of various text mining and clustering approaches in the specific context of safety-critical systems, providing insights for practitioners and researchers.
更多查看译文
AI 理解论文
溯源树
样例
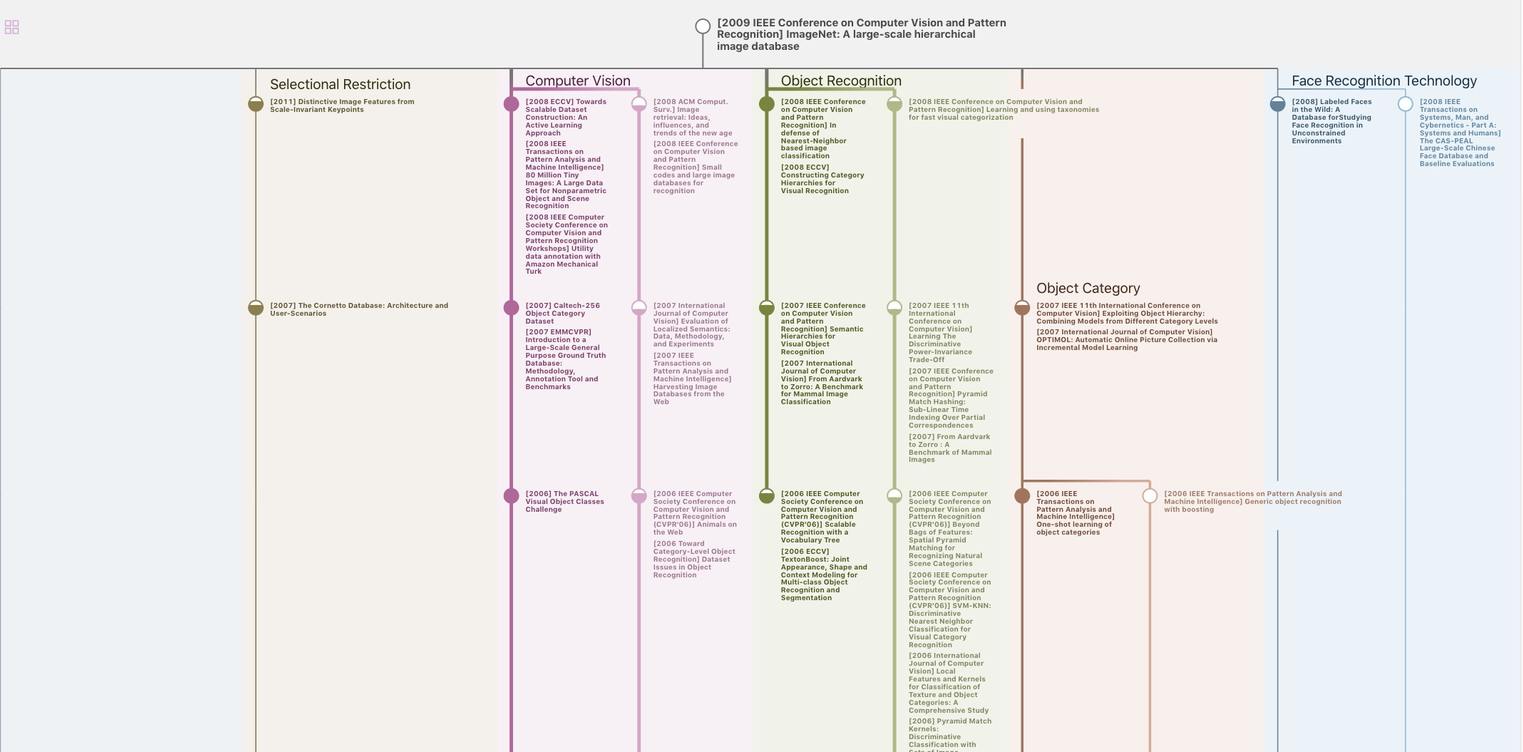
生成溯源树,研究论文发展脉络
Chat Paper
正在生成论文摘要